Heterogeneous Multi-Task Learning With Expert Diversity.
IEEE/ACM Transactions on Computational Biology and Bioinformatics(2022)
摘要
Predicting multiple heterogeneous biological and medical targets is a challenge for traditional deep learning models. In contrast to single-task learning, in which a separate model is trained for each target, multi-task learning (MTL) optimizes a single model to predict multiple related targets simultaneously. To address this challenge, we propose the Multi-gate Mixture-of-Experts with Exclusivity (MMoEEx). Our work aims to tackle the heterogeneous MTL setting, in which the same model optimizes multiple tasks with different characteristics. Such a scenario can overwhelm current MTL approaches due to the challenges in balancing shared and task-specific representations and the need to optimize tasks with competing optimization paths. Our method makes two key contributions: first, we introduce an approach to induce more diversity among experts, thus creating representations more suitable for highly imbalanced and heterogenous MTL learning; second, we adopt a two-step optimization (Finn et al., 2017 and Lee et al., 2020) approach to balancing the tasks at the gradient level. We validate our method on three MTL benchmark datasets, including UCI-Census-income dataset, Medical Information Mart for Intensive Care (MIMIC-III) and PubChem BioAssay (PCBA).
更多查看译文
关键词
Multi-task Learning,neural network,mixture of experts,task balancing
AI 理解论文
溯源树
样例
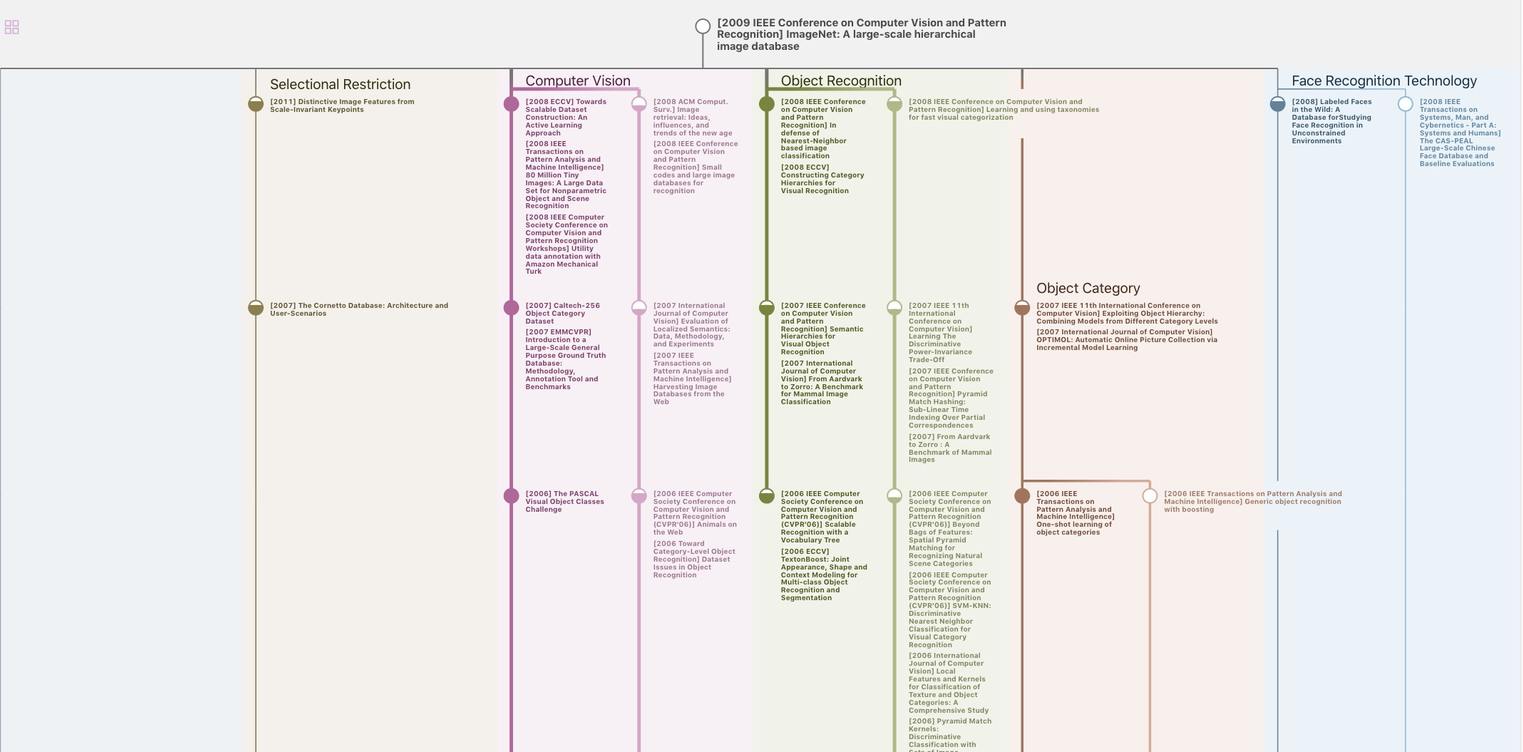
生成溯源树,研究论文发展脉络
Chat Paper
正在生成论文摘要