Multi-contextual design of convolutional neural network for steganalysis
Multimedia Tools and Applications(2024)
摘要
In recent times, deep learning-based steganalysis classifiers have become popular due to their state-of-the-art performance. Most deep steganalysis classifiers usually extract noise residuals using high-pass filters as preprocessing steps and feed them to their deep model for classification. It is observed that recent steganographic embedding does not always restrict their embedding in the high-frequency zone; instead, they distribute it as per embedding policy. Therefore, besides noise residual, learning the embedding zone is another challenging task. In this work, unlike the conventional approaches, the proposed model first extracts the noise residual using learned denoising kernels to boost the signal-to-noise ratio. After preprocessing, the sparse noise residuals are fed to a novel Multi-Contextual Convolutional Neural Network (M-CNET) that uses heterogeneous context size to learn the sparse and low-amplitude representation of noise residuals. The model performance is further improved by incorporating the Self-Attention module to focus on the areas prone to steganalytic embedding. A set of comprehensive experiments is performed to show the proposed scheme’s efficacy over the prior arts. Besides, an ablation study is given to justify the contribution of various modules of the proposed architecture.
更多查看译文
关键词
Steganalysis,Spatial domain steganalysis,Denoising kernels,Multi-contextual learning
AI 理解论文
溯源树
样例
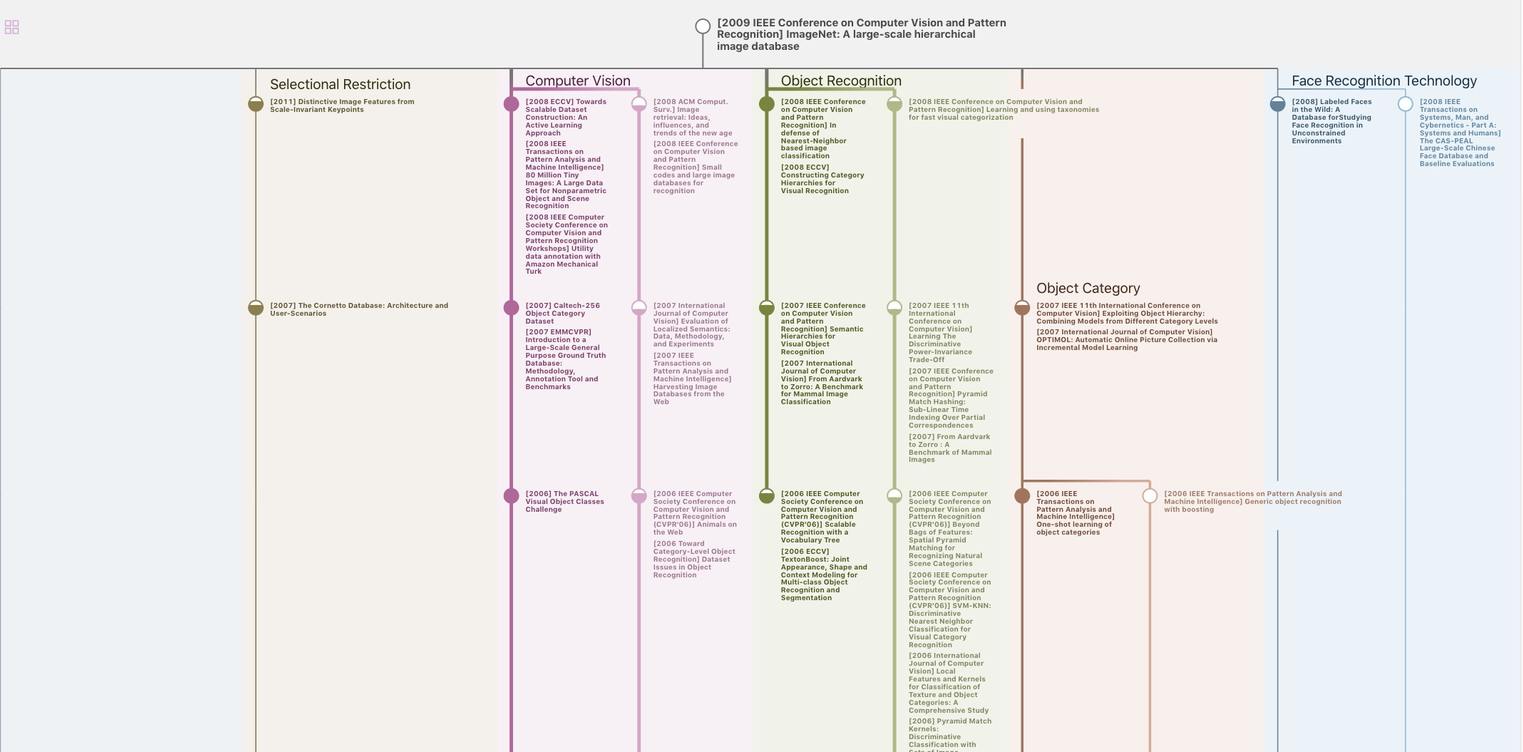
生成溯源树,研究论文发展脉络
Chat Paper
正在生成论文摘要