An Efficient Video Prediction Recurrent Network using Focal Loss and Decomposed Tensor Train for Imbalance Dataset
Great Lakes Symposium on VLSI(2021)
摘要
ABSTRACTNowadays, from companies to academics, researchers across the world are interested in developing recurrent neural networks due to their incredible feats in various applications, such as speech recognition, video detection, predictions, and machine translation. However, the advantages of recurrent neural networks accompanied by high computational and power demands, which are a major design constraint for electronic devices with limited resources used in such network implementations. Optimizing the recurrent neural networks, such as model compression, is crucial to ensure the broad deployment of recurrent neural networks and promote recurrent neural networks for implementing most resource-constrained scenarios. Among many techniques, tensor train (TT) decomposition is considered an up-and-coming technology. Although our previous efforts have achieved 1) expanding limits of many multiplications within eliminating all redundant computations; and 2) decomposing into multi-stage processing to reduce memory traffic, this work still faces some limitations. In particular, current TT decomposition on recurrent neural networks leads to a complex computation sensitive to the quality of training datasets. In this paper, we investigate a new method for TT decomposition on recurrent neural networks for constructing an efficient model within imbalance datasets to overcome this issue. Experimental results show that the proposed new training method can achieve significant improvements in accuracy, precision, recall, F1-score, False Negative Rate (FNR), and False Omission Rate (FOR).
更多查看译文
AI 理解论文
溯源树
样例
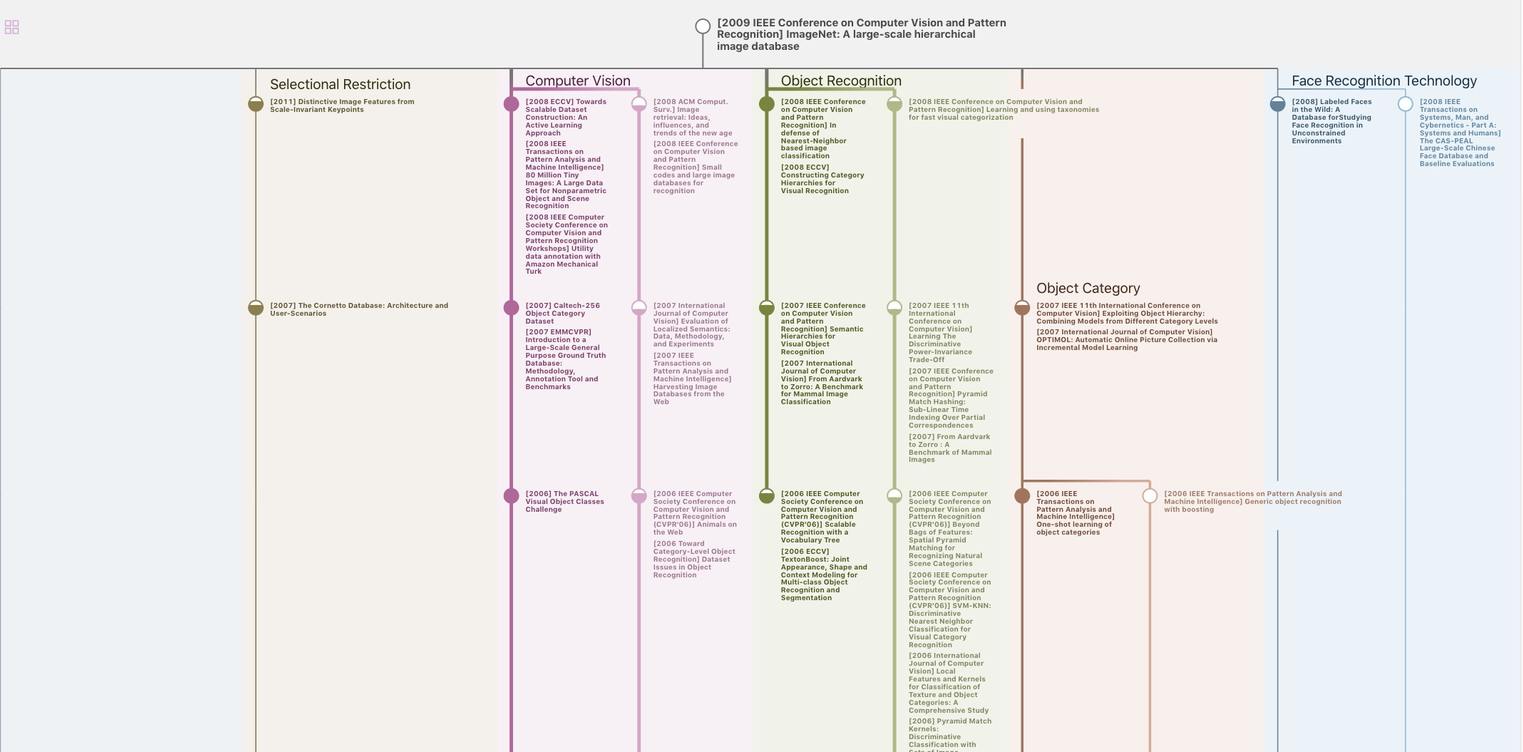
生成溯源树,研究论文发展脉络
Chat Paper
正在生成论文摘要