PACE: Learning Effective Task Decomposition for Human-in-the-loop Healthcare Delivery
International Conference on Management of Data(2021)
摘要
ABSTRACTHuman-in-the-loop data analysis involves both machine learning models and humans in analytic tasks. In healthcare applications, human-in-the-loop data analysis is crucial in that the model can handle "easy" tasks and hand over "hard" ones to medical experts for assistance and medical judgment, where easy tasks are the ones for which the model can provide high accuracy and hard tasks vice versa. In this process, how to decompose tasks in an effective manner is an important stage. To achieve task decomposition, classification with a reject option is a solution. However, existing studies either directly implement a reject option or dive into the theoretical details of the rejection mechanism. Different from such studies, we aim to optimize general classifiers with a reject option and hence, optimize task decomposition for healthcare applications. To this end, we first introduce task decomposition for healthcare applications, which is a crucial stage in human-in-the-loop healthcare delivery. We then devise a framework PACE to learn effective task decomposition concentrating on delivering high performance on the easy tasks. PACE is two-level: on the macro level, PACE employs the Self-Paced Learning method to select easy tasks for each training iteration; on the micro level, PACE adapts the weights of selected tasks through its weighted loss revision strategy. Experimental results in two real-world healthcare datasets show that PACE outperforms baselines in terms of their performance on the easy tasks which are expected to be solved by the learning model.
更多查看译文
关键词
Healthcare, Task decomposition, Human-in-the-loop
AI 理解论文
溯源树
样例
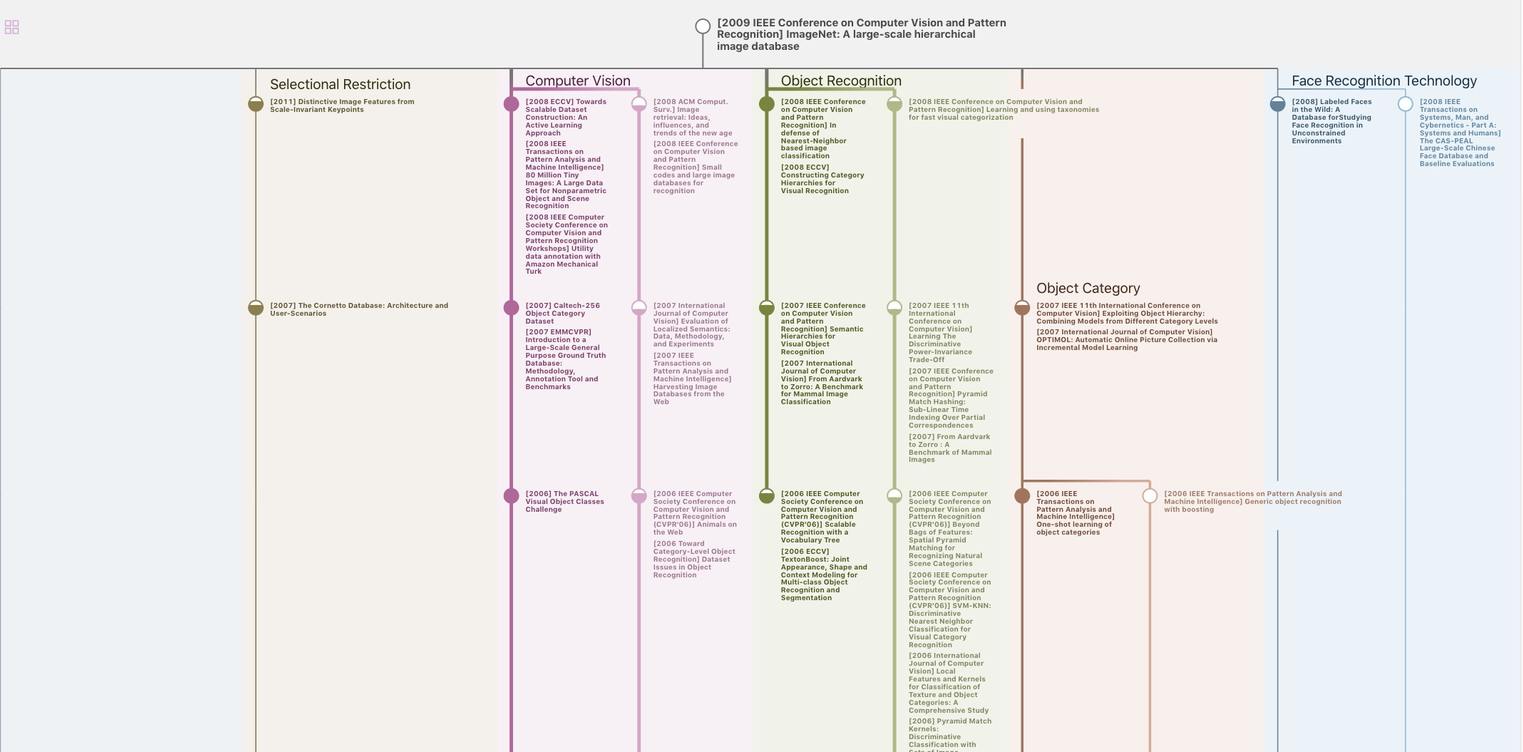
生成溯源树,研究论文发展脉络
Chat Paper
正在生成论文摘要