Pool of Experts: Realtime Querying Specialized Knowledge in Massive Neural Networks
International Conference on Management of Data(2021)
摘要
ABSTRACTIn spite of the great success of deep learning technologies, training and delivery of a practically serviceable model is still a highly time-consuming process. Furthermore, a resulting model is usually too generic and heavyweight, and hence essentially goes through another expensive model compression phase to fit in a resource-limited device like embedded systems. Inspired by the fact that a machine learning task specifically requested by mobile users is often much simpler than it is supported by a massive generic model, this paper proposes a framework, called Pool of Experts (PoE), that instantly builds a lightweight and task-specific model without any training process. For a realtime model querying service, PoE first extracts a pool of primitive components, called experts, from a well-trained and sufficiently generic network by exploiting a novel conditional knowledge distillation method, and then performs our train-free knowledge consolidation to quickly combine necessary experts into a lightweight network for a target task. Thanks to this train-free property, in our thorough empirical study, PoE can build a fairly accurate yet compact model in a realtime manner, whereas it takes a few minutes per query for the other training methods to achieve a similar level of the accuracy.
更多查看译文
关键词
Lightweight Neural Networks, Knowledge Distillation, Model Specialization, Model Compression
AI 理解论文
溯源树
样例
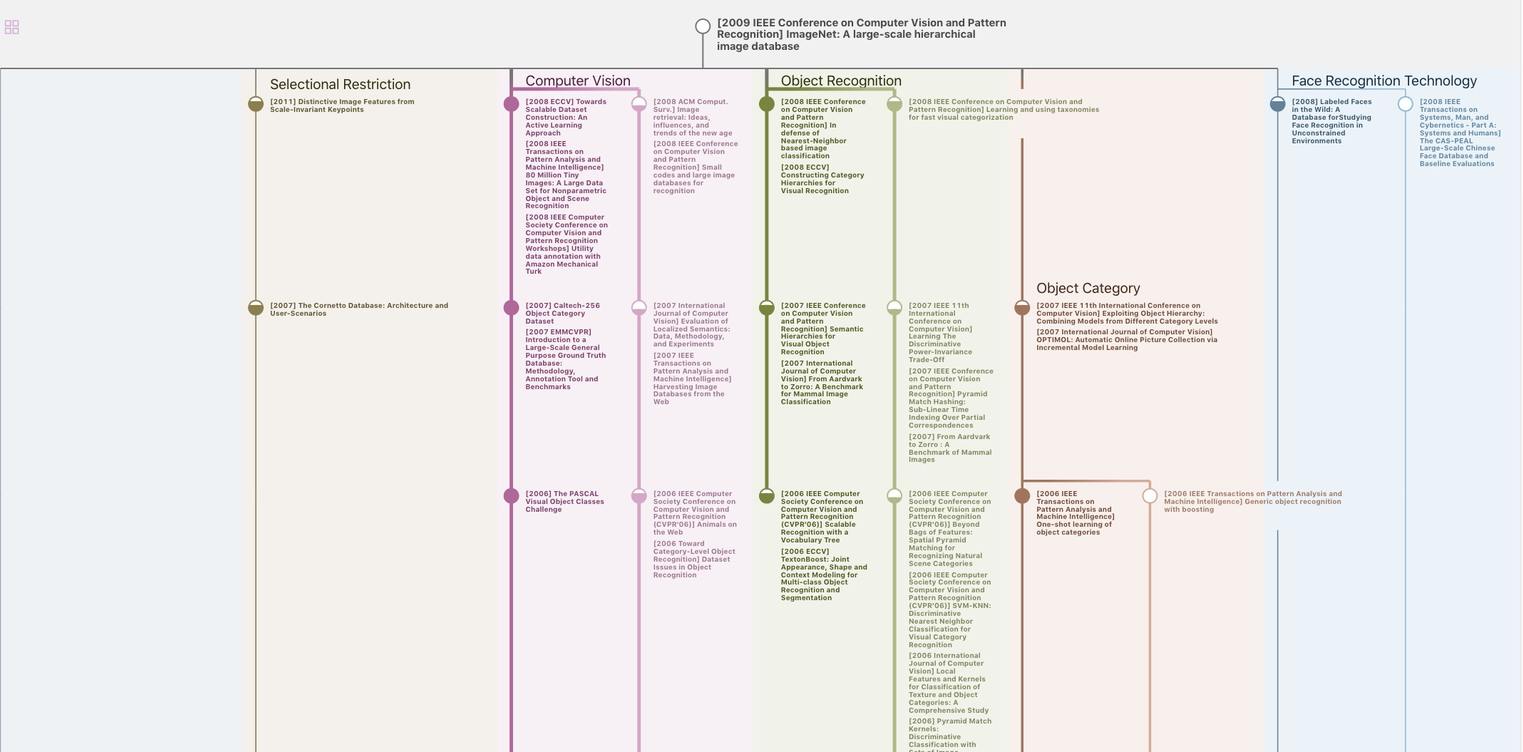
生成溯源树,研究论文发展脉络
Chat Paper
正在生成论文摘要