GraphGem: Optimized Scalable System for Graph Convolutional Networks
International Conference on Management of Data(2021)
摘要
ABSTRACTDeep Learning (DL), especially Graph Convolutional Networks (GCNs) have revolutionized several domains and applications dealing with unstructured data with non-euclidean and graphical relationships. Constructing large-scale Deep GCNs, however, are bottlenecked by glaring systems issues due to memory blow-ups, runtime slowdowns with random access, and I/O costs. This research abstract identifies various systems and scalability issues and proposes a novel system called GraphGem to handle GCN-centric DL tasks end-to-end. GraphGem tackles the bottlenecks by elevating entire GCN workloads for convenient input declarations by the user, and is inspired by lessons from the databases and machine learning systems worlds. This abstract also highlights the bigger picture of the potential research impact alongside tacking systems constraints and what it may mean for data science and deep learning practitioners going forward.
更多查看译文
关键词
Neural Networks, Graph Convolutional Networks, Systems, Deep Learning, I/O, embeddings, feature extraction, storage, random access
AI 理解论文
溯源树
样例
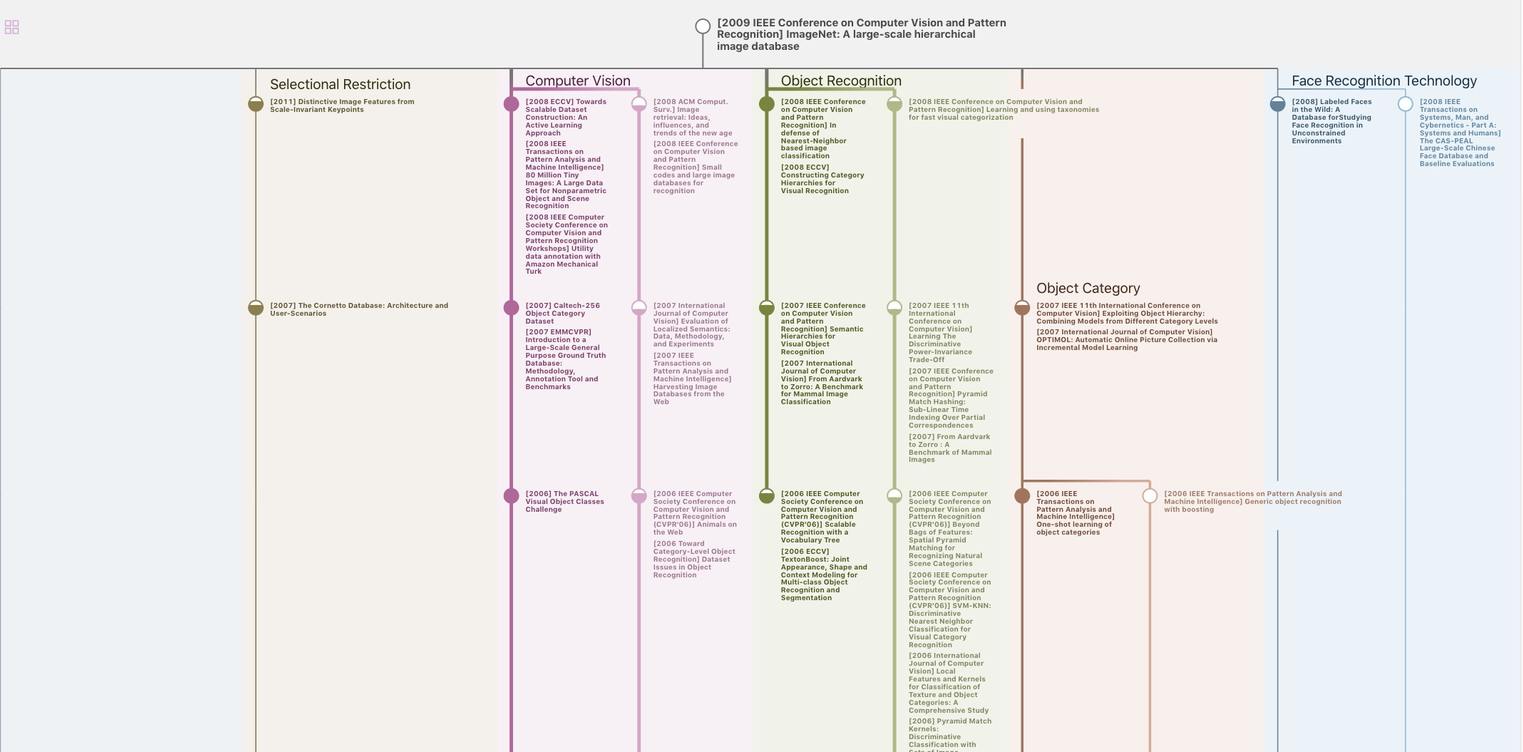
生成溯源树,研究论文发展脉络
Chat Paper
正在生成论文摘要