Nonparametric Differentially Private Confidence Intervals for the Median
JOURNAL OF SURVEY STATISTICS AND METHODOLOGY(2022)
摘要
Differential privacy is a restriction on data processing algorithms that provides strong confidentiality guarantees for individual records in the data. However, research on proper statistical inference, that is, research on properly quantifying the uncertainty of the (noisy) sample estimate regarding the true value in the population, is currently still limited. This article proposes and evaluates several strategies to compute valid differentially private confidence intervals for the median. Instead of computing a differentially private point estimate and deriving its uncertainty, we directly estimate the interval bounds and discuss why this approach is superior if ensuring privacy is important. We also illustrate that addressing both sources of uncertainty-the error from sampling and the error from protecting the output-simultaneously should be preferred over simpler approaches that incorporate the uncertainty in a sequential fashion. We evaluate the performance of the different algorithms under various parameter settings in extensive simulation studies and demonstrate how the findings could be applied in practical settings using data from the 1940 Decennial Census.
更多查看译文
关键词
Robust, Statistical inference, Confidentiality, Disclosure limitation
AI 理解论文
溯源树
样例
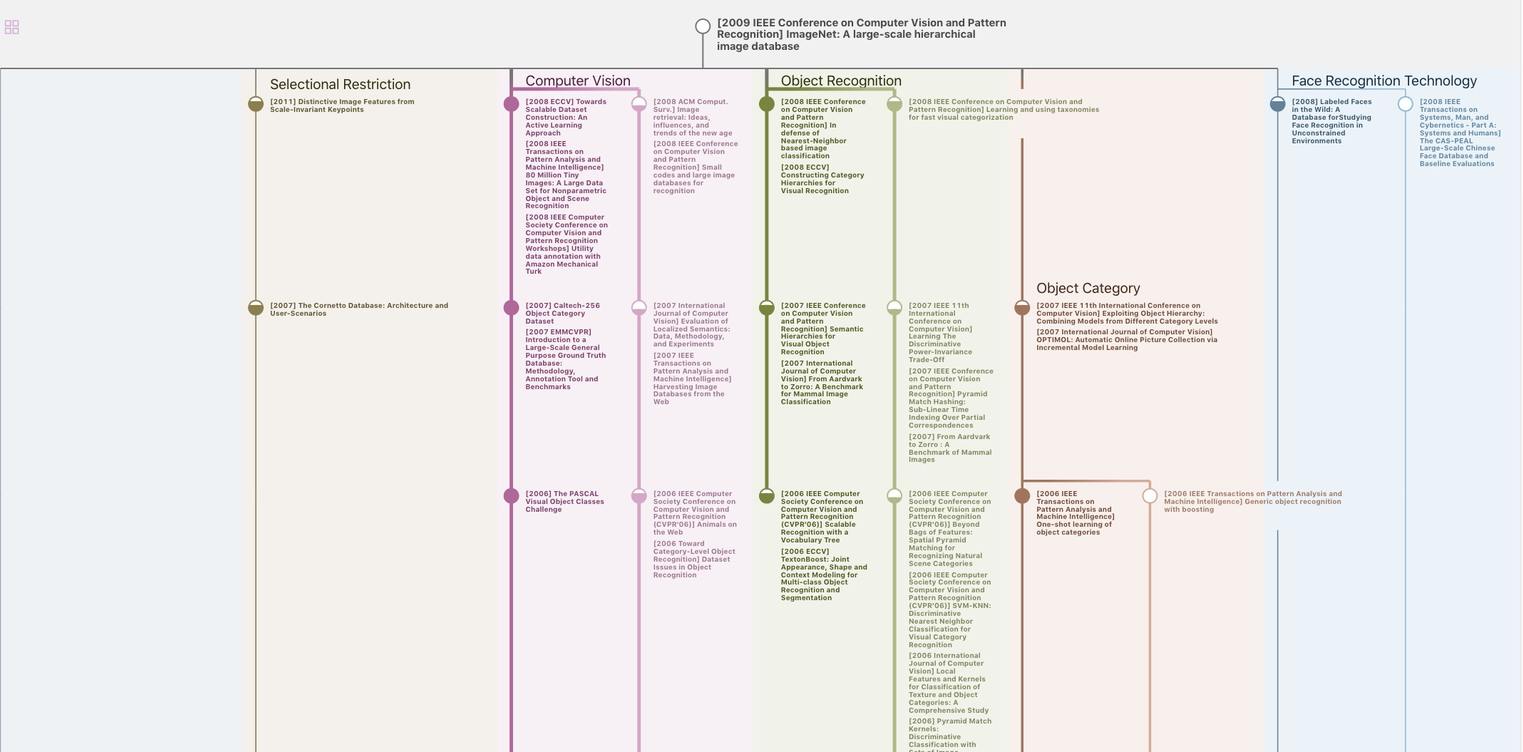
生成溯源树,研究论文发展脉络
Chat Paper
正在生成论文摘要