Weakly Supervised Deep Reinforcement Learning for Video Summarization With Semantically Meaningful Reward
2021 IEEE WINTER CONFERENCE ON APPLICATIONS OF COMPUTER VISION WACV 2021(2021)
摘要
Conventional unsupervised video summarization algorithms are usually developed in a frame level clustering manner For example, frame level diversity and representativeness are two typical clustering criteria used for unsupervised reinforcement learning-based video summarization. Inspired by recent progress in video representation techniques, we further introduce the similarity of video representations to construct a semantically meaningful reward for this task. We consider that a good summarization should also be semantically identical to its original source, which means that the semantic similarity can be regarded as an additional criterion for summarization. Through combining a novel video semantic reward with other unsupervised rewards for training, we can easily upgrade an unsupervised reinforcement learning-based video summarization method to its weakly supervised version. In practice, we first train a video classification sub-network (VCSN) to extract video semantic representations based on a category-labeled video dataset. Then we fix this VCSN and train a summary generation sub-network (SGSN) using unlabeled video data in a reinforcement learning way. Experimental results demonstrate that our work significantly surpasses other unsupervised and even supervised methods. To the best of our knowledge, our method achieves state-of-the-art performance in terms of the correlation coefficients, Kendall's and Spearman's p.
更多查看译文
关键词
weakly supervised version,video classification sub-network,video semantic representations,category-labeled video dataset,unlabeled video data,reinforcement learning way,unsupervised methods,even supervised methods,weakly supervised deep reinforcement,semantically meaningful reward,conventional unsupervised video summarization algorithms,frame level clustering manner,frame level diversity,representativeness,typical clustering criteria,video representation techniques,video representations,good summarization,semantic similarity,video semantic reward,unsupervised rewards,unsupervised reinforcement learning-based video summarization method
AI 理解论文
溯源树
样例
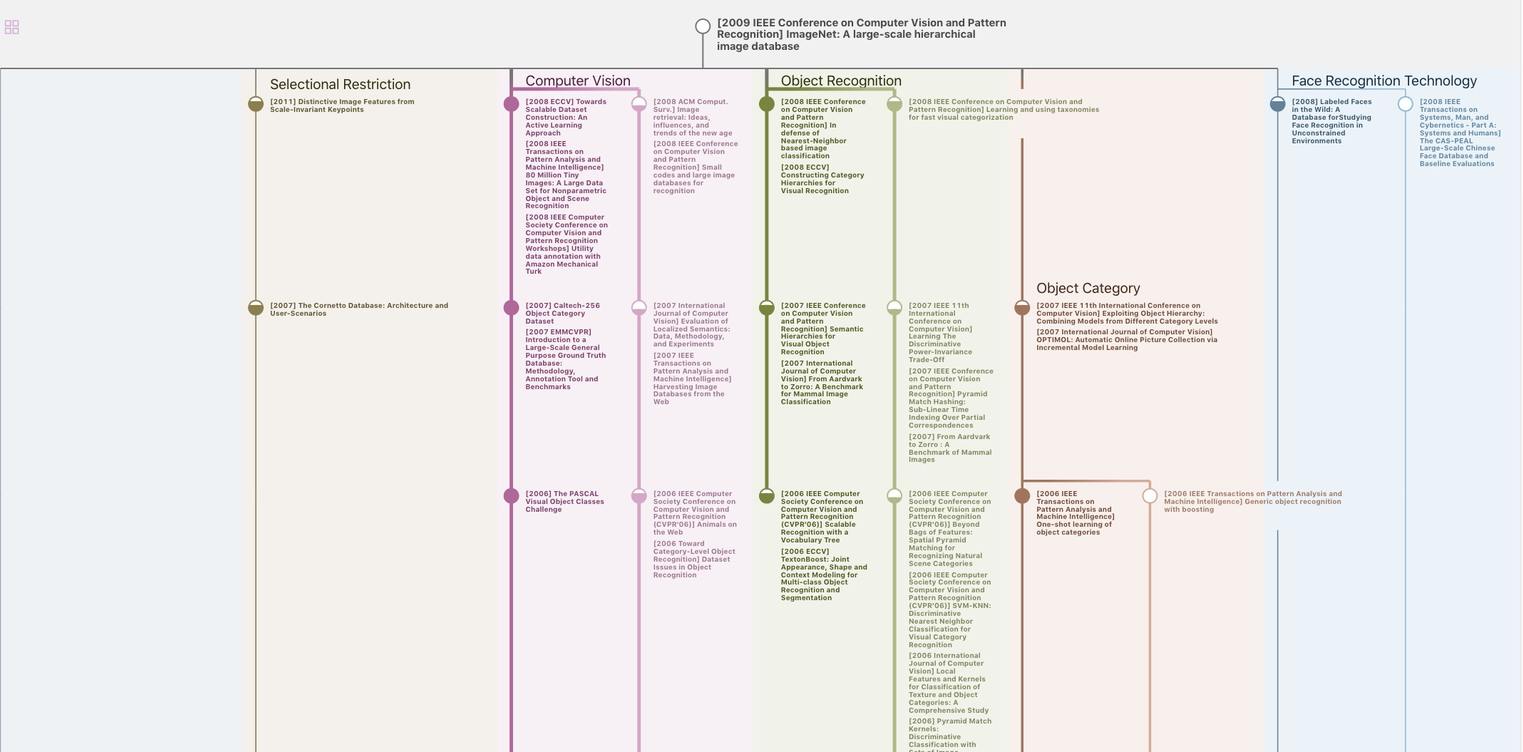
生成溯源树,研究论文发展脉络
Chat Paper
正在生成论文摘要