Fairface: Face Attribute Dataset For Balanced Race, Gender, And Age For Bias Measurement And Mitigation
2021 IEEE WINTER CONFERENCE ON APPLICATIONS OF COMPUTER VISION (WACV 2021)(2021)
摘要
Existing public face image datasets are strongly biased toward Caucasian faces, and other races (e.g., Latino) are significantly underrepresented. The models trained from such datasets suffer from inconsistent classification accuracy, which limits the applicability of face analytic systems to non-White race groups. To mitigate the race bias problem in these datasets, we constructed a novel face image dataset containing 108,501 images which is balanced on race. We define 7 race groups: White, Black, Indian, East Asian, Southeast Asian, Middle Eastern, and Latino. Images were collected from the YFCC-100M Flickr dataset and labeled with race, gender, and age groups. Evaluations were performed on existing face attribute datasets as well as novel image datasets to measure the generalization performance. We find that the model trained from our dataset is substantially more accurate on novel datasets and the accuracy is consistent across race and gender groups. We also compare several commercial computer vision APIs and report their balanced accuracy across gender, race, and age groups.
更多查看译文
关键词
bias measurement,public face image datasets,Caucasian faces,inconsistent classification accuracy,face analytic systems,race bias problem,face image dataset,YFCC-100M Flickr dataset,age groups,novel datasets,gender groups,balanced accuracy,face attribute dataset,non-White race groups,commerical computer vision APIs
AI 理解论文
溯源树
样例
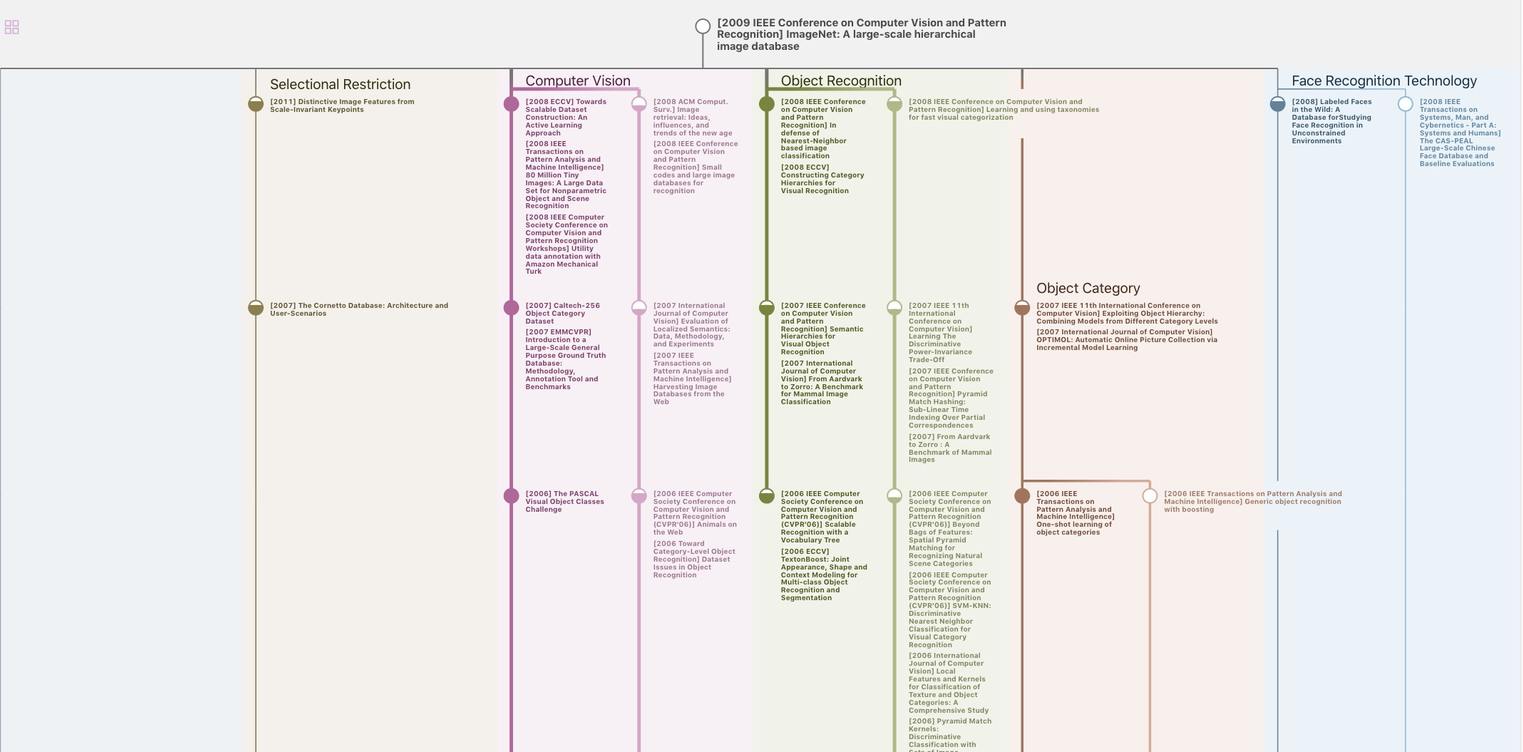
生成溯源树,研究论文发展脉络
Chat Paper
正在生成论文摘要