Improving Robustness And Uncertainty Modelling In Neural Ordinary Differential Equations
2021 IEEE WINTER CONFERENCE ON APPLICATIONS OF COMPUTER VISION WACV 2021(2021)
摘要
Deep learning models such as Resnets have resulted in state-of-the-art accuracy in many computer vision problems. Neural ordinary differential equations (NODE) provides a continuous depth generalization of Resnets and overcome drawbacks of Resnet such as model selection and parameter complexity. Though NODE is more robust than Resnet, we find that NODE based architectures are still far away from providing robustness and uncertainty handling required for many computer vision problems. We propose novel NODE models which address these drawbacks. In particular, we propose Gaussian processes (GPs) to model the fully connected neural networks in NODE (NODE-GP) to improve robustness and uncertainty handling capabilities of NODE. The proposed model is flexible to accommodate different NODE architectures, and further improves the model selection capabilities in NODEs. We also find that numerical techniques play an important role in modelling NODE robustness, and propose to use different numerical techniques to improve NODE robustness. We demonstrate the superior robustness and uncertainty handling capabilities of proposed models on adversarial attacks and out-of-distribution experiments for the image classification tasks.
更多查看译文
关键词
robustness improvement,uncertainty modelling,neural ordinary differential equations,deep learning models,Resnet,computer vision problems,parameter complexity,NODE based architectures,fully connected neural networks,NODE-GP,model selection capabilities,NODE robustness modelling,NODE architectures,Gaussian processes,image classification,adversarial attacks,out-of-distribution experiments
AI 理解论文
溯源树
样例
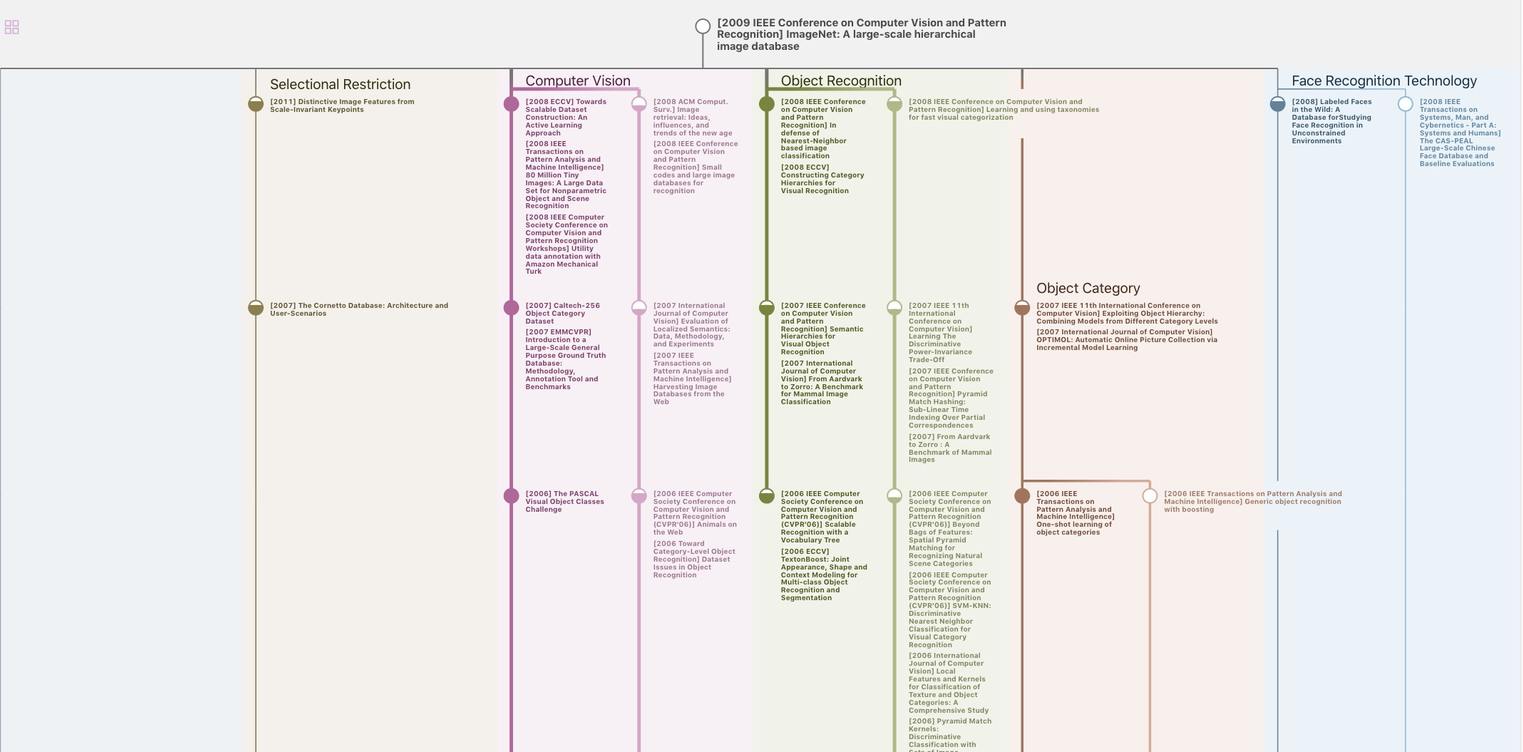
生成溯源树,研究论文发展脉络
Chat Paper
正在生成论文摘要