Mobility-Aware Qos Promotion And Load Balancing In Mec-Based Vehicular Networks: A Deep Learning Approach
2021 IEEE 93RD VEHICULAR TECHNOLOGY CONFERENCE (VTC2021-SPRING)(2021)
摘要
Recently, Multi-access Edge Computing (MEC) has become a promising enabler to support emerging applications in vehicular networks by offloading compute-intensive tasks from vehicles to proximate MEC servers. However, the high mobility of vehicles brings difficulties to provide reliable services in the MEC system due to potential outages of communication in the process of offloading. Also, load balancing of the MEC system is seldom considered in previous offloading schemes, which may increase the risk of system failure and reduce Quality of Service (QoS) of vehicles due to congestions. Currently, we still lack a low-complexity method to address these issues. In this paper, we aim to promote QoS of vehicular applications by taking vehicles' mobility and latency requirements into account while guaranteeing load balancing of the MEC system. Specifically, we first formulate the joint offloading decision and resource allocation problem as a Mixed Integer NonLinear Programming (MINLP) problem. Then, by taking advantage of both Deep Neural Network (DNN) and Particle Swarm Optimization (PSO), we propose a novel framework to effectively address the problem, where PSO accelerates the training by providing high quality labeled data to DNN. Finally, simulation results show that our proposed method outperforms traditional heuristic algorithms in terms of QoS and runtime.
更多查看译文
关键词
Computation offloading, Deep neural network, Multi-access edge computing, Mobility, Load balancing
AI 理解论文
溯源树
样例
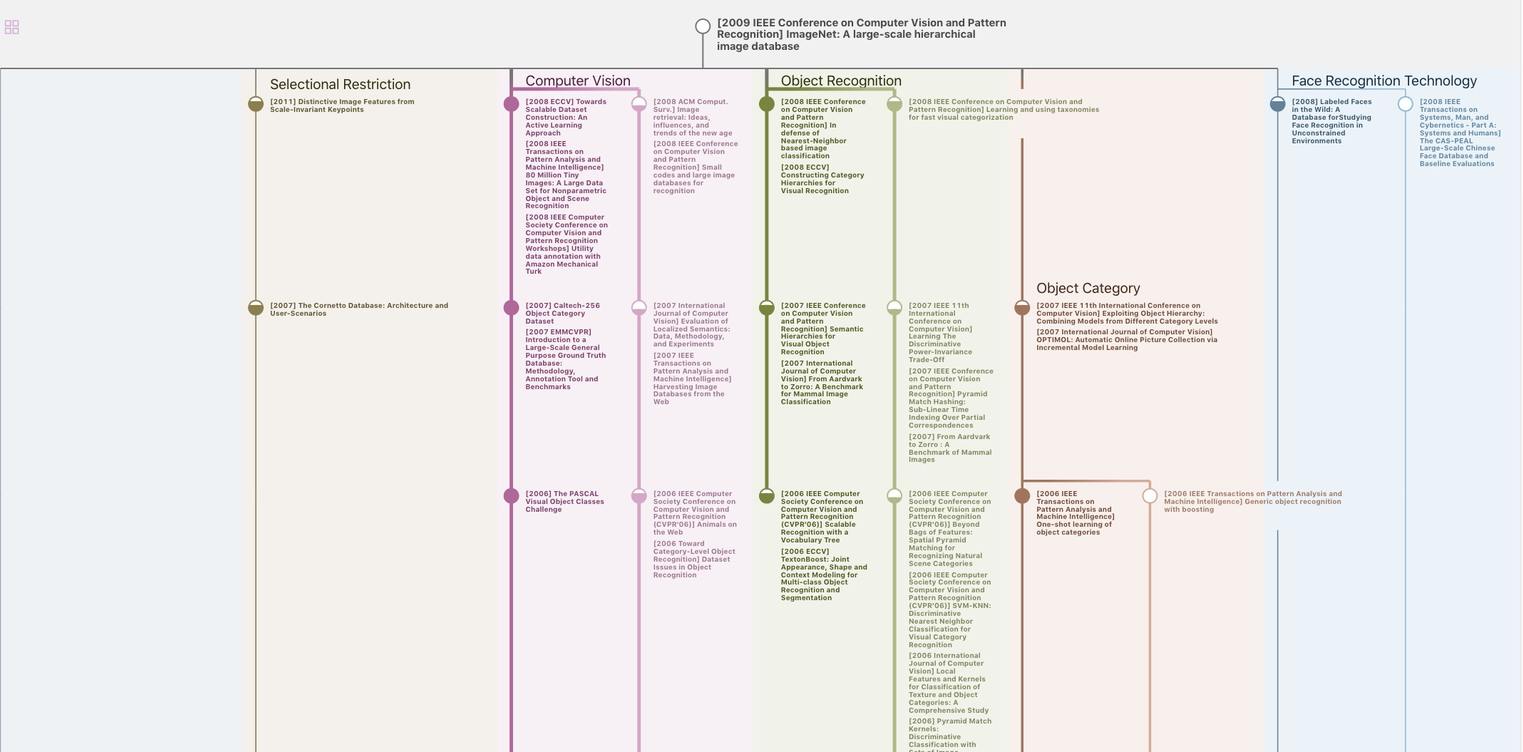
生成溯源树,研究论文发展脉络
Chat Paper
正在生成论文摘要