Self-supervised Incremental Deep Graph Learning for Ethereum Phishing Scam Detection
arxiv(2021)
摘要
In recent years, phishing scams have become the crime type with the largest money involved on Ethereum, the second-largest blockchain platform. Meanwhile, graph neural network (GNN) has shown promising performance in various node classification tasks. However, for Ethereum transaction data, which could be naturally abstracted to a real-world complex graph, the scarcity of labels and the huge volume of transaction data make it difficult to take advantage of GNN methods. Here in this paper, to address the two challenges, we propose a Self-supervised Incremental deep Graph learning model (SIEGE), for the phishing scam detection problem on Ethereum. In our model, two pretext tasks designed from spatial and temporal perspectives help us effectively learn useful node embedding from the huge amount of unlabelled transaction data. And the incremental paradigm allows us to efficiently handle large-scale transaction data and help the model maintain good performance when the data distribution is drastically changing. We collect transaction records about half a year from Ethereum and our extensive experiments show that our model consistently outperforms strong baselines in both transductive and inductive settings.
更多查看译文
关键词
ethereum phishing
AI 理解论文
溯源树
样例
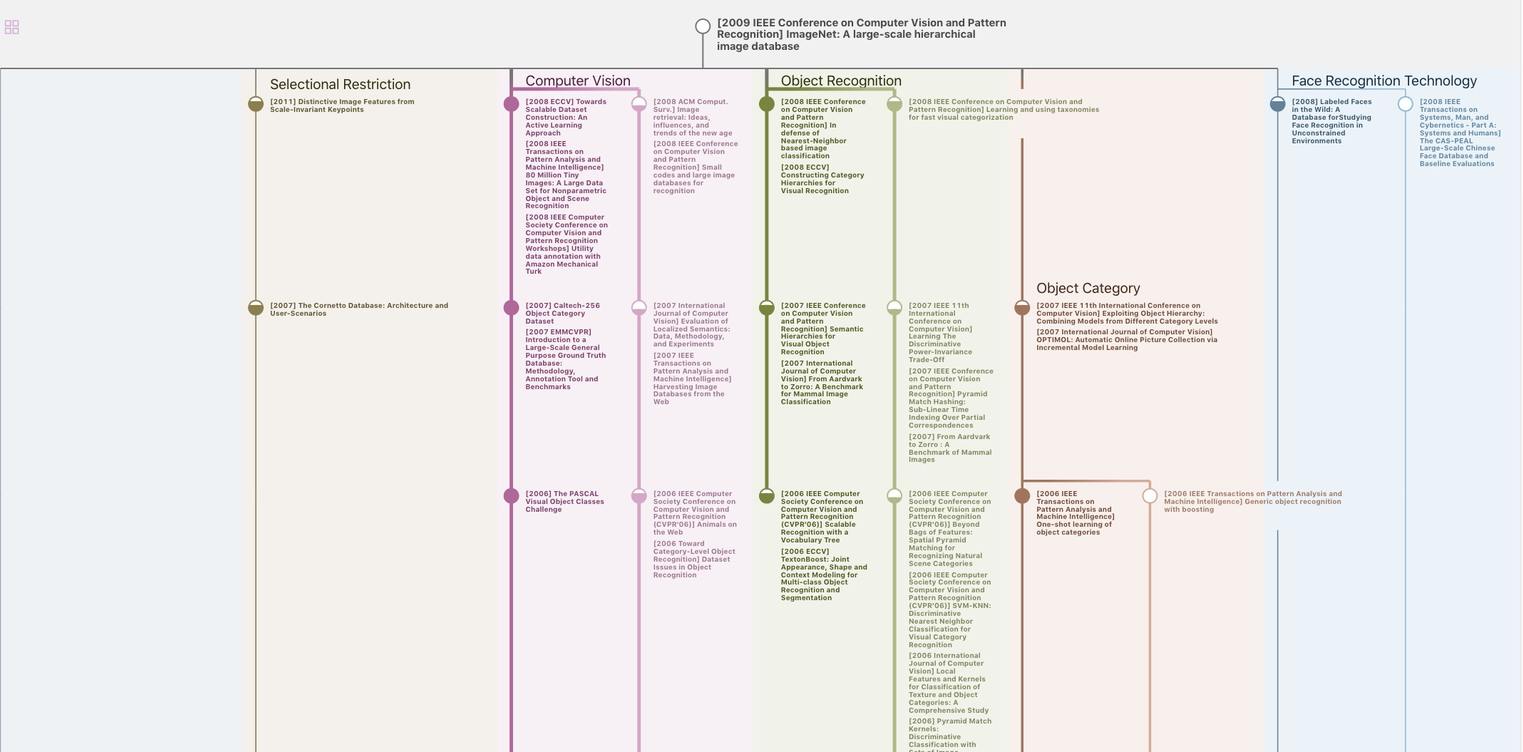
生成溯源树,研究论文发展脉络
Chat Paper
正在生成论文摘要