Multilayer Sparsity-Based Tensor Decomposition for Low-Rank Tensor Completion
IEEE Transactions on Neural Networks and Learning Systems(2022)
摘要
Existing methods for tensor completion (TC) have limited ability for characterizing low-rank (LR) structures. To depict the complex hierarchical knowledge with implicit sparsity attributes hidden in a tensor, we propose a new multilayer sparsity-based tensor decomposition (MLSTD) for the low-rank tensor completion (LRTC). The method encodes the structured sparsity of a tensor by the multiple-layer representation. Specifically, we use the CANDECOMP/PARAFAC (CP) model to decompose a tensor into an ensemble of the sum of rank-1 tensors, and the number of rank-1 components is easily interpreted as the first-layer sparsity measure. Presumably, the factor matrices are smooth since local piecewise property exists in within-mode correlation. In subspace, the local smoothness can be regarded as the second-layer sparsity. To describe the refined structures of factor/subspace sparsity, we introduce a new sparsity insight of subspace smoothness: a self-adaptive low-rank matrix factorization (LRMF) scheme, called the third-layer sparsity. By the progressive description of the sparsity structure, we formulate an MLSTD model and embed it into the LRTC problem. Then, an effective alternating direction method of multipliers (ADMM) algorithm is designed for the MLSTD minimization problem. Various experiments in RGB images, hyperspectral images (HSIs), and videos substantiate that the proposed LRTC methods are superior to state-of-the-art methods.
更多查看译文
关键词
CANDECOMP/PARAFAC (CP) decomposition,factor smooth prior,low-rank tensor completion (LRTC),multilayer sparsity (MLS) constraints,subspace structured sparsity
AI 理解论文
溯源树
样例
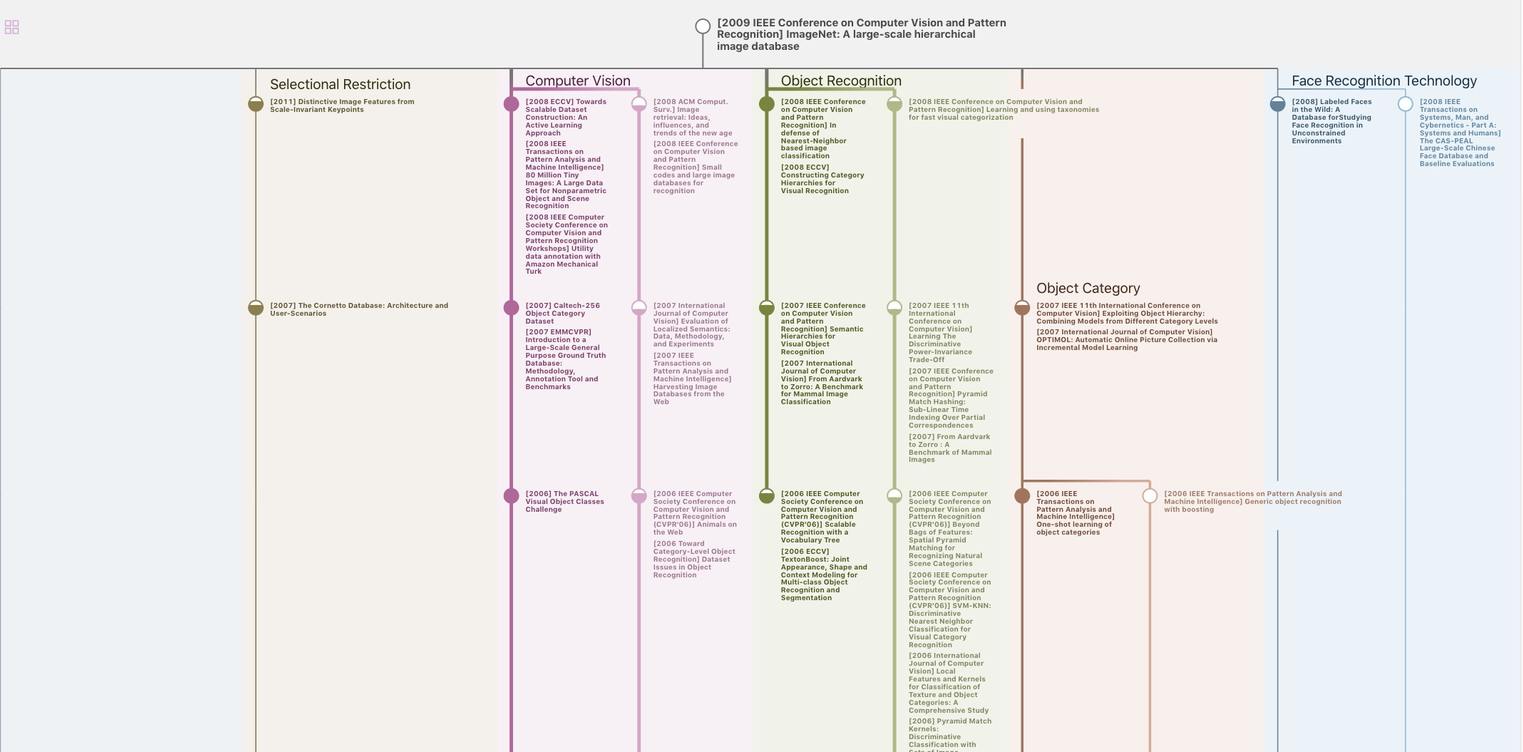
生成溯源树,研究论文发展脉络
Chat Paper
正在生成论文摘要