Using Machine Learning To Predict Severe Hypoglycaemia In Hospital
DIABETES OBESITY & METABOLISM(2021)
摘要
Aim To predict the risk of hypoglycaemia using machine-learning techniques in hospitalized patients. Methods We conducted a retrospective cohort study of patients hospitalized under general internal medicine (GIM) and cardiovascular surgery (CV) at a tertiary care teaching hospital in Toronto, Ontario. Three models were generated using supervised machine learning: least absolute shrinkage and selection operator (LASSO) logistic regression; gradient-boosted trees; and a recurrent neural network. Each model included baseline patient data and time-varying data. Natural-language processing was used to incorporate text data from physician and nursing notes. Results We included 8492 GIM admissions and 8044 CV admissions. Hypoglycaemia occurred in 16% of GIM admissions and 13% of CV admissions. The area under the curve for the models in the held-out validation set was approximately 0.80 on the GIM ward and 0.82 on the CV ward. When the threshold for hypoglycaemia was lowered to 2.9 mmol/L (52 mg/dL), similar results were observed. Among the patients at the highest decile of risk, the positive predictive value was approximately 50% and the sensitivity was 99%. Conclusion Machine-learning approaches can accurately identify patients at high risk of hypoglycaemia in hospital. Future work will involve evaluating whether implementing this model with targeted clinical interventions can improve clinical outcomes.
更多查看译文
关键词
hypoglycaemia
AI 理解论文
溯源树
样例
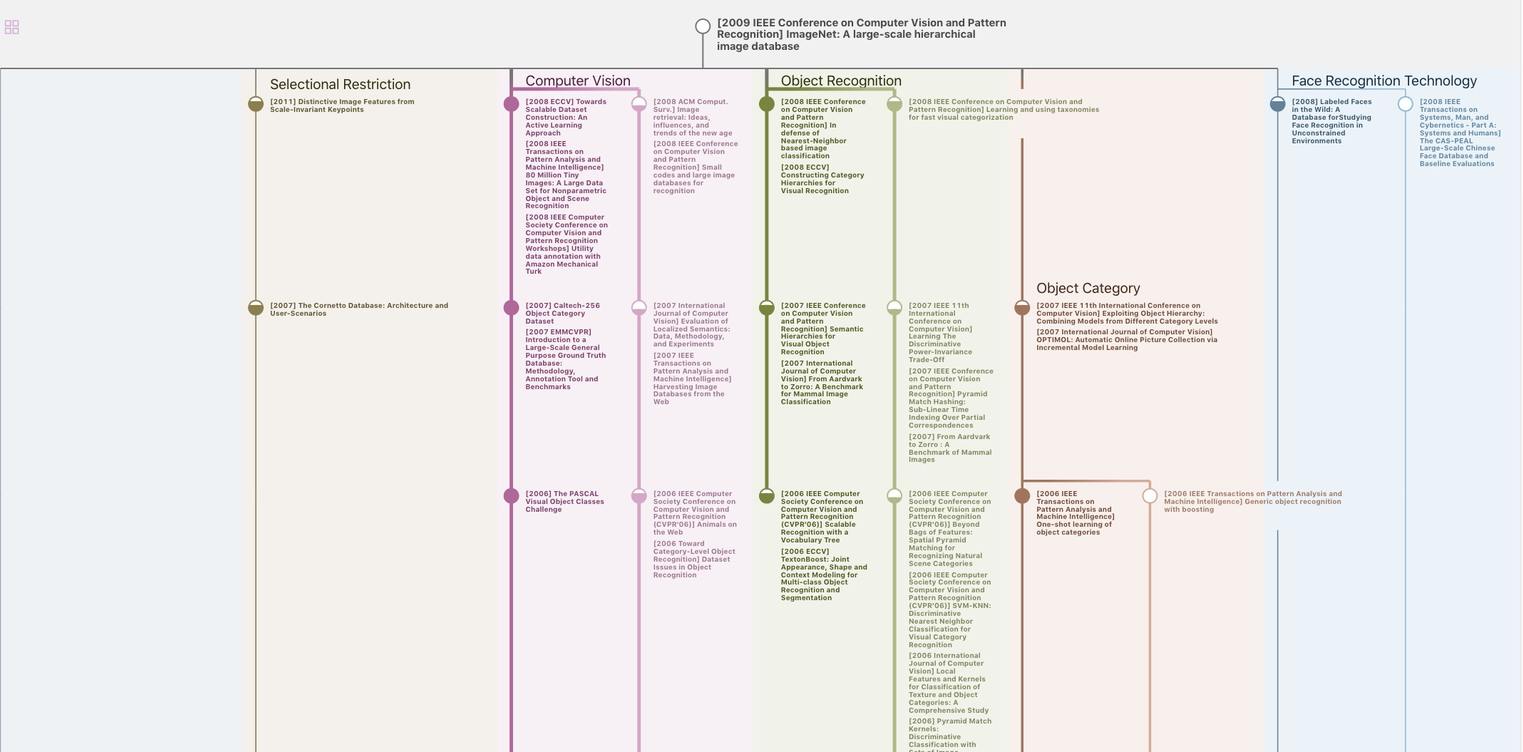
生成溯源树,研究论文发展脉络
Chat Paper
正在生成论文摘要