Scaling-up Diverse Orthogonal Convolutional Networks by a Paraunitary Framework.
International Conference on Machine Learning(2022)
摘要
Enforcing orthogonality in convolutional neural networks is a remedy for gradient vanishing/exploding problems and sensitivity to perturbation. Many previous approaches for orthogonal convolutions enforce orthogonality on its flattened kernel, which, however, do not lead to the orthogonality of the operation. Some recent approaches consider orthogonality for standard convolutional layers and propose specific classes of their realizations. In this work, we propose a theoretical framework that establishes the equivalence between diverse orthogonal convolutional layers in the spatial domain and the paraunitary systems in the spectral domain. Since 1D paraunitary systems admit a complete factorization, we can parameterize any separable orthogonal convolution as a composition of spatial filters. As a result, our framework endows high expressive power to various convolutional layers while maintaining their exact orthogonality. Furthermore, our layers are memory and computationally efficient for deep networks compared to previous designs. Our versatile framework, for the first time, enables the study of architectural designs for deep orthogonal networks, such as choices of skip connection, initialization, stride, and dilation. Consequently, we scale up orthogonal networks to deep architectures, including ResNet and ShuffleNet, substantially outperforming their shallower counterparts. Finally, we show how to construct residual flows, a flow-based generative model that requires strict Lipschitzness, using our orthogonal networks. Our code will be publicly available at https://github.com/umd-huang-lab/ortho-conv
更多查看译文
关键词
diverse orthogonal convolutional networks,paraunitary framework
AI 理解论文
溯源树
样例
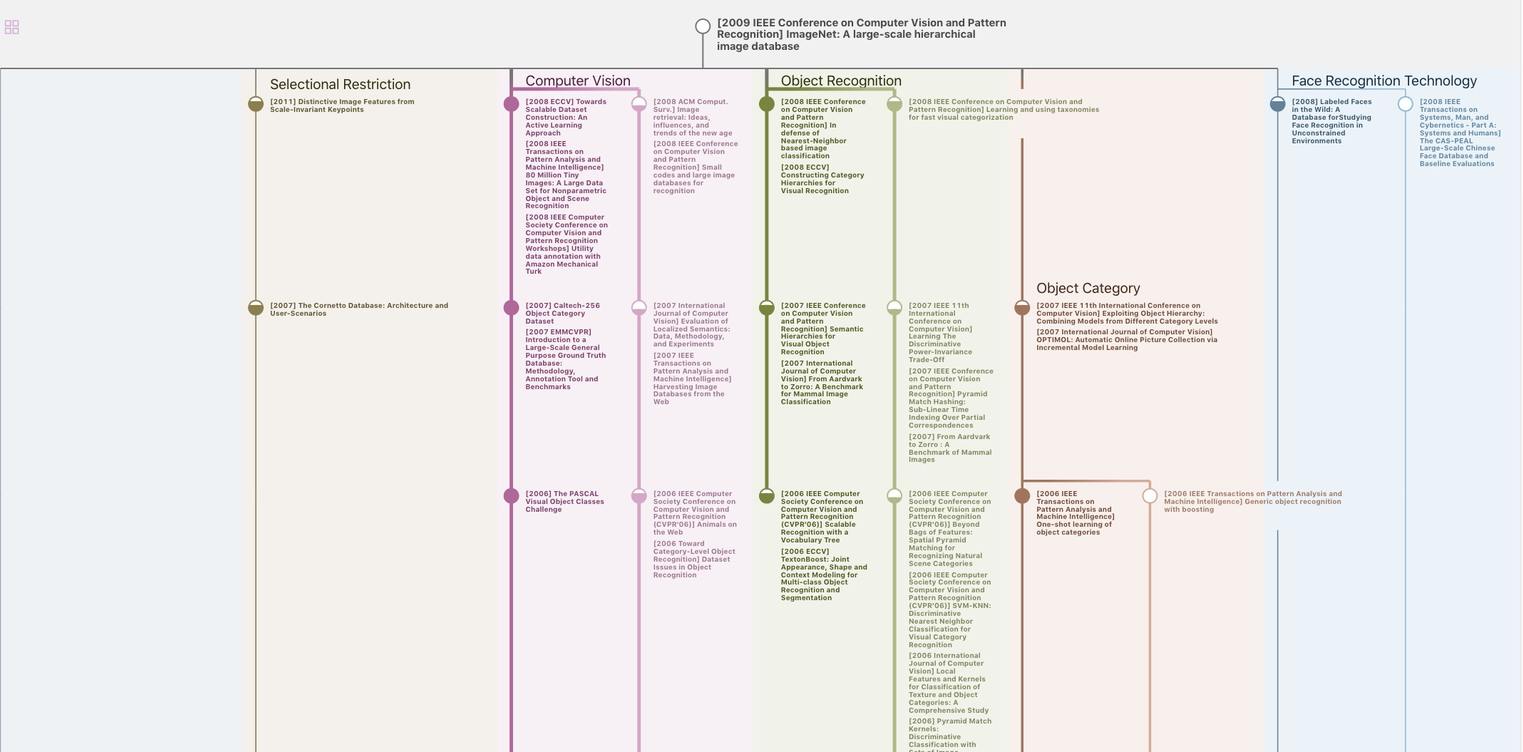
生成溯源树,研究论文发展脉络
Chat Paper
正在生成论文摘要