Improved CNN-Based Learning of Interpolation Filters for Low-Complexity Inter Prediction in Video Coding
IEEE Open Journal of Signal Processing(2021)
摘要
The versatility of recent machine learning approaches makes them ideal for improvement of next generation video compression solutions. Unfortunately, these approaches typically bring significant increases in computational complexity and are difficult to interpret into explainable models, affecting their potential for implementation within practical video coding applications. This paper introduces a novel explainable neural network-based inter-prediction scheme, to improve the interpolation of reference samples needed for fractional precision motion compensation. The approach requires a single neural network to be trained from which a full quarter-pixel interpolation filter set is derived, as the network is easily interpretable due to its linear structure. A novel training framework enables each network branch to resemble a specific fractional shift. This practical solution makes it very efficient to use alongside conventional video coding schemes. When implemented in the context of the state-of-the-art Versatile Video Coding (VVC) test model, 0.77%, 1.27% and 2.25% BD-rate savings can be achieved on average for lower resolution sequences under the random access, low-delay B and low-delay P configurations, respectively, while the complexity of the learned interpolation schemes is significantly reduced compared to the interpolation with full CNNs.
更多查看译文
关键词
Video compression,motion compensation,interpolation,machine learning,complexity reduction
AI 理解论文
溯源树
样例
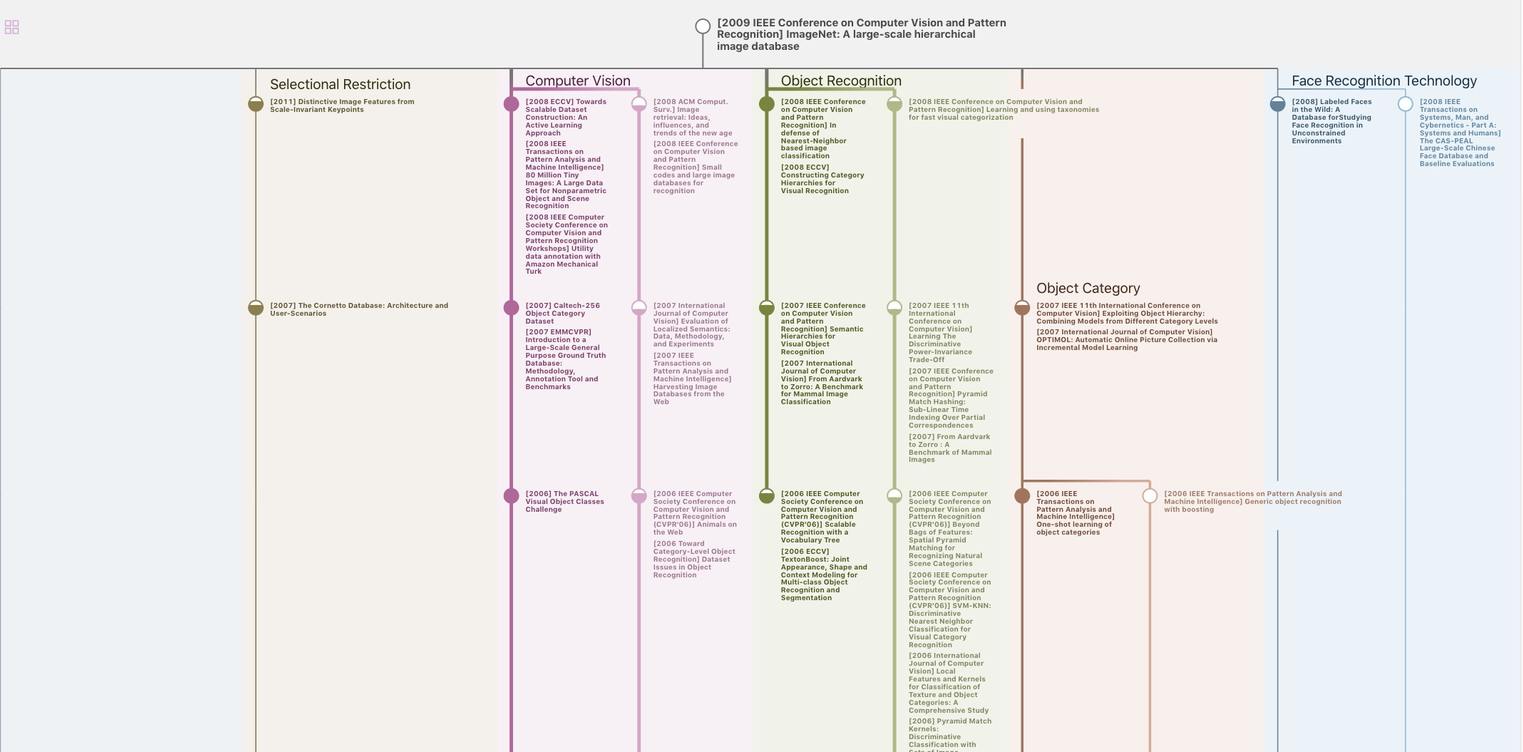
生成溯源树,研究论文发展脉络
Chat Paper
正在生成论文摘要