X-MAN: Explaining multiple sources of anomalies in video
2021 IEEE/CVF CONFERENCE ON COMPUTER VISION AND PATTERN RECOGITION WORKSHOPS (CVPRW 2021)(2021)
摘要
Our objective is to detect anomalies in video while also automatically explaining the reason behind the detector's response. In a practical sense, explainability is crucial for this task as the required response to an anomaly depends on its nature and severity. However, most leading methods (based on deep neural networks) are not interpretable and hide the decision making process in uninterpretable feature representations. In an effort to tackle this problem we make the following contributions: (1) we show how to build interpretable feature representations suitable for detecting anomalies with state of the art performance, (2) we propose an interpretable probabilistic anomaly detector which can describe the reason behind it's response using high level concepts, (3) we are the first to directly consider object interactions for anomaly detection and (4) we propose a new task of explaining anomalies and release a large dataset for evaluating methods on this task. Our method competes well with the state of the art on public datasets while also providing anomaly explanation based on objects and their interactions.
更多查看译文
关键词
anomaly explanation,explainability,deep neural networks,uninterpretable feature representations,interpretable feature representations,interpretable probabilistic anomaly detector,anomaly detection,X-MAN,explaining multiple sources of anomalies,video,decision making process
AI 理解论文
溯源树
样例
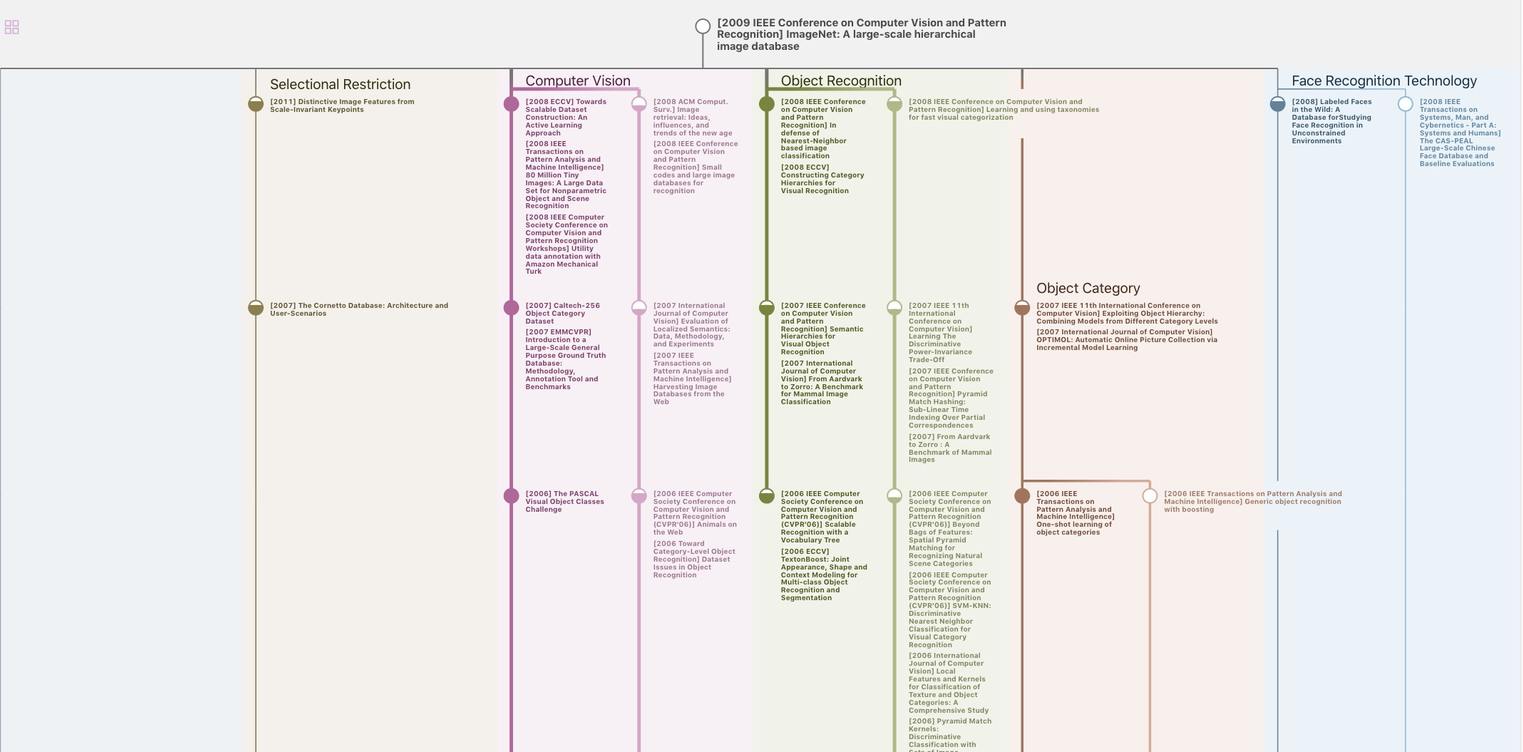
生成溯源树,研究论文发展脉络
Chat Paper
正在生成论文摘要