Knowledge-Adaptation Priors
arxiv(2021)
摘要
Humans and animals have a natural ability to quickly adapt to their surroundings, but machine-learning models, when subjected to changes, often require a complete retraining from scratch. We present Knowledge-adaptation priors (K-priors) to reduce the cost of retraining by enabling quick and accurate adaptation for a wide-variety of tasks and models. This is made possible by a combination of weight and function-space priors to reconstruct the gradients of the past, which recovers and generalizes many existing, but seemingly-unrelated, adaptation strategies. Training with simple first-order gradient methods can often recover the exact retrained model to an arbitrary accuracy by choosing a sufficiently large memory of the past data. Empirical results confirm that the adaptation can be cheap and accurate, and a promising alternative to retraining.
更多查看译文
关键词
knowledge-adaptation
AI 理解论文
溯源树
样例
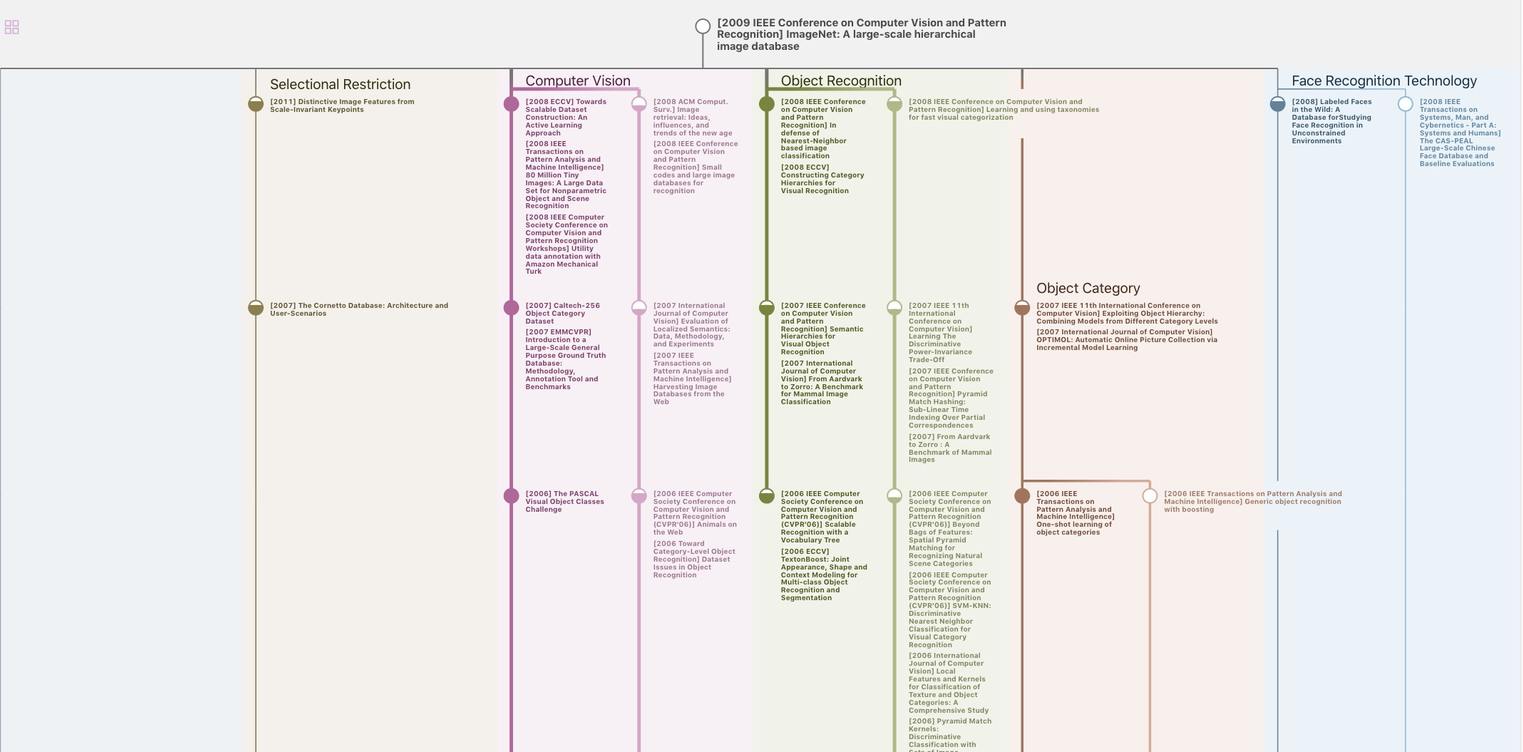
生成溯源树,研究论文发展脉络
Chat Paper
正在生成论文摘要