Optimal Accounting of Differential Privacy via Characteristic Function
INTERNATIONAL CONFERENCE ON ARTIFICIAL INTELLIGENCE AND STATISTICS, VOL 151(2022)
摘要
Characterizing the privacy degradation over compositions, i.e., privacy accounting, is a fundamental topic in differential privacy (DP) with many applications to differentially private machine learning and federated learning. We propose a unification of recent advances (Renyi DP, privacy profiles, f-DP and the PLD formalism) via the characteristic function (phi-function) of a certain dominating privacy loss random variable. We show that our approach allows natural adaptive composition like Renyi DP, provides exactly tight privacy accounting like PLD, and can be (often losslessly) converted to privacy profile and f-DP, thus providing (epsilon, delta)-DP guarantees and interpretable tradeoff functions. Algorithmically, we propose an analytical Fourier accountant that represents the complex logarithm of phi-functions symbolically and uses Gaussian quadrature for numerical computation. On several popular DP mechanisms and their subsampled counterparts, we demonstrate the flexibility and tightness of our approach in theory and experiments.
更多查看译文
关键词
differential privacy,optimal accounting
AI 理解论文
溯源树
样例
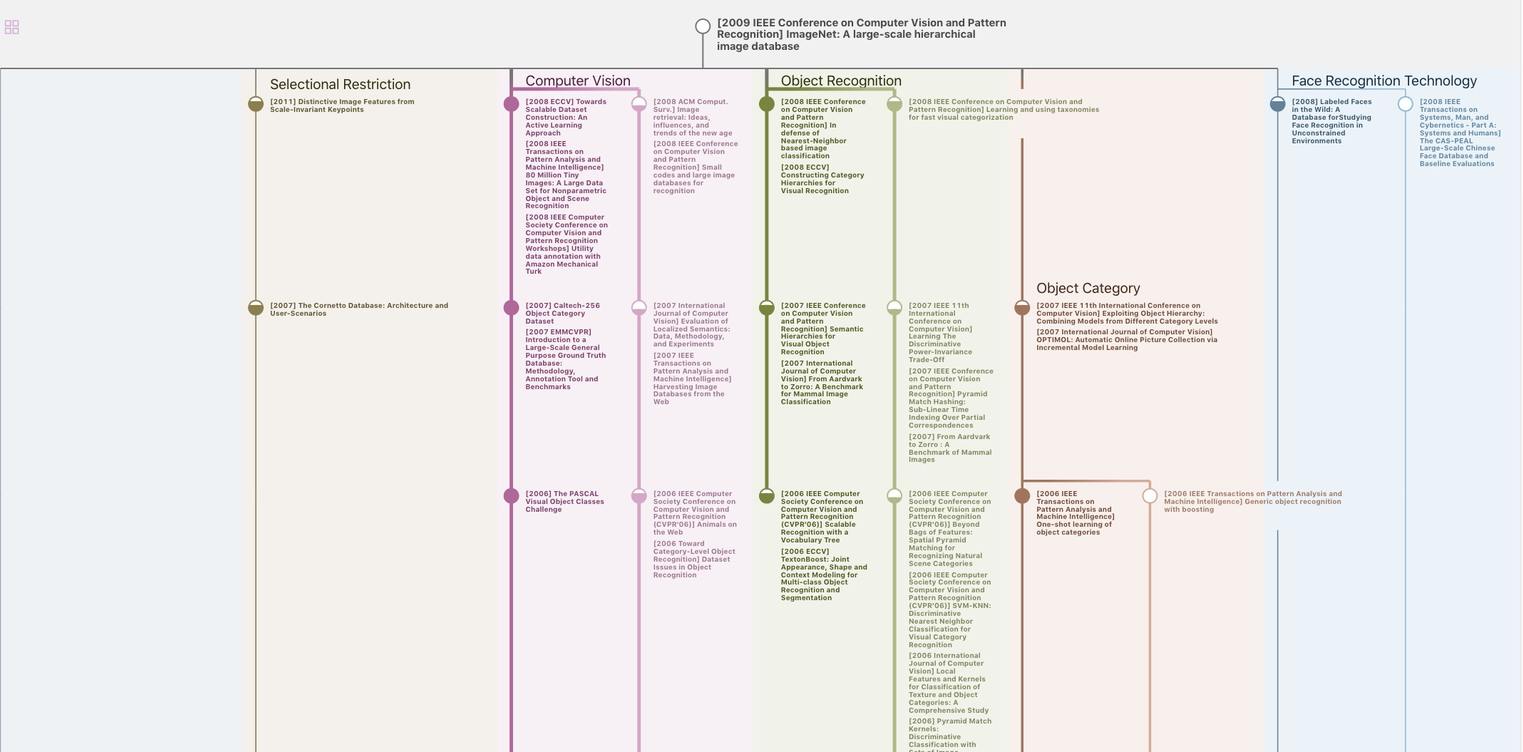
生成溯源树,研究论文发展脉络
Chat Paper
正在生成论文摘要