Tackling the Challenges in Scene Graph Generation With Local-to-Global Interactions.
IEEE transactions on neural networks and learning systems(2022)
摘要
In this work, we seek new insights into the underlying challenges of the scene graph generation (SGG) task. Quantitative and qualitative analysis of the visual genome (VG) dataset implies: 1) ambiguity: even if interobject relationship contains the same object (or predicate), they may not be visually or semantically similar; 2) asymmetry: despite the nature of the relationship that embodied the direction, it was not well addressed in previous studies; and 3) higher-order contexts: leveraging the identities of certain graph elements can help generate accurate scene graphs. Motivated by the analysis, we design a novel SGG framework, Local-to-global interaction networks (LOGINs). Locally, interactions extract the essence between three instances of subject, object, and background, while baking direction awareness into the network by explicitly constraining the input order of subject and object. Globally, interactions encode the contexts between every graph component (i.e., nodes and edges). Finally, Attract and Repel loss is utilized to fine-tune the distribution of predicate embeddings. By design, our framework enables predicting the scene graph in a bottom-up manner, leveraging the possible complementariness. To quantify how much LOGIN is aware of relational direction, a new diagnostic task called Bidirectional Relationship Classification (BRC) is also proposed. Experimental results demonstrate that LOGIN can successfully distinguish relational direction than existing methods (in BRC task), while showing state-of-the-art results on the VG benchmark (in SGG task).
更多查看译文
关键词
Task analysis,Visualization,Semantics,Context modeling,Benchmark testing,Learning systems,Analytical models,Bidirectional relationship classification (BRC),scene graph generation,scene understanding,visual relationship detection
AI 理解论文
溯源树
样例
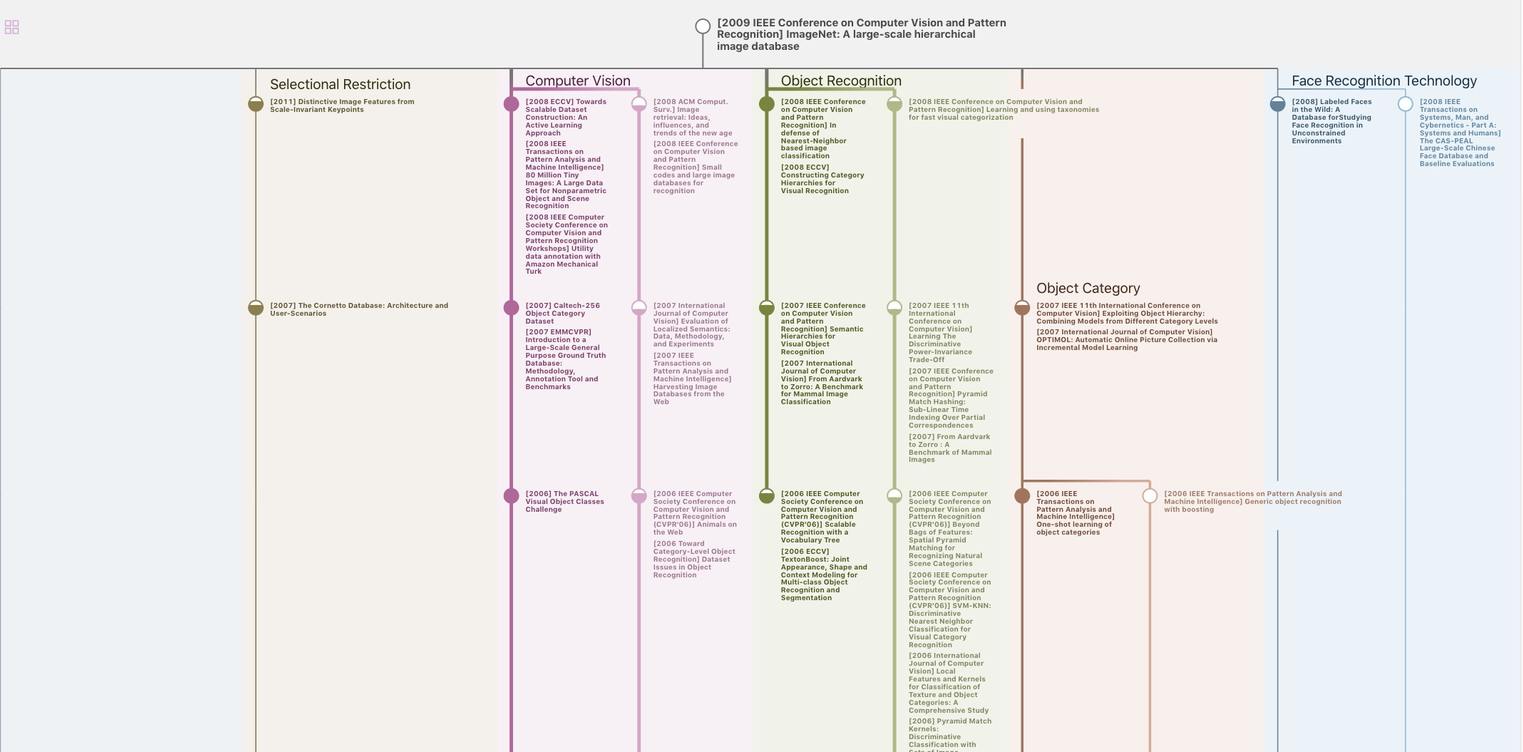
生成溯源树,研究论文发展脉络
Chat Paper
正在生成论文摘要