Understanding and Evaluating Racial Biases in Image Captioning.
ICCV(2021)
摘要
Image captioning is an important task for benchmarking visual reasoning and for enabling accessibility for people with vision impairments. However, as in many machine learning settings, social biases can influence image captioning in undesirable ways. In this work, we study bias propagation pathways within image captioning, focusing specifically on the COCO dataset. Prior work has analyzed gender bias in captions using automatically-derived gender labels; here we examine racial and intersectional biases using manual annotations. Our first contribution is in annotating the perceived gender and skin color of 28,315 of the depicted people after obtaining IRB approval. Using these annotations, we compare racial biases present in both manual and automatically-generated image captions. We demonstrate differences in caption performance, sentiment, and word choice between images of lighter versus darker-skinned people. Further, we find the magnitude of these differences to be greater in modern captioning systems compared to older ones, thus leading to concerns that without proper consideration and mitigation these differences will only become increasingly prevalent. Code and data is available at https://princetonvisualai.github.io/imagecaptioning-bias .
更多查看译文
关键词
Fairness,accountability,transparency,and ethics in vision,Datasets and evaluation,Vision + language
AI 理解论文
溯源树
样例
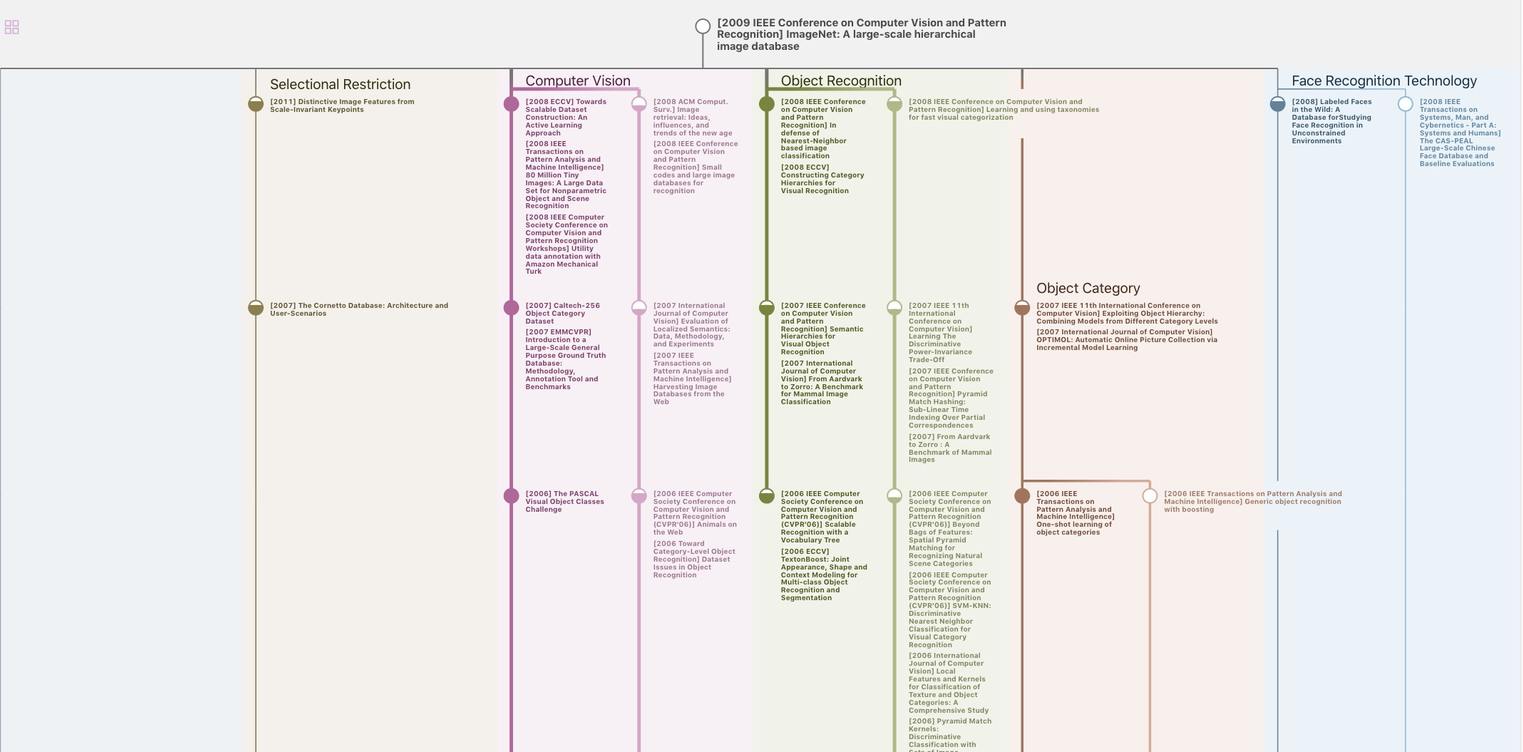
生成溯源树,研究论文发展脉络
Chat Paper
正在生成论文摘要