Exploring Distance Based Approaches For Reducing Sensor Data In Defect Related Prognosis
12TH INTERNATIONAL CONFERENCE ON AMBIENT SYSTEMS, NETWORKS AND TECHNOLOGIES (ANT) / THE 4TH INTERNATIONAL CONFERENCE ON EMERGING DATA AND INDUSTRY 4.0 (EDI40) / AFFILIATED WORKSHOPS(2021)
摘要
Vibration data consists of batches of time series which if accumulated over a period of time is a huge collection of numeric data. Reducing such data for use in deep learning models for computational efficiency is a challenge. Combinatorial and discrete approaches, on the other hand, is not an extensively explored area when it comes to datasets. This paper aims to identify feature reduction techniques based on discrete approaches such as euclidean distance using dot products on vibration data samples from accelerometers fitted on bearings. In this limited experimentation, the procured dataset by this approach is considerably smaller in size as compared to the actual complete data, and with comparable results in prediction models, it can be used as a smaller representation of a sensor timeline. The results based on different models show that such reductions can be considered in building IoT applications in industries based on sensors. (C) 2021 The Authors. Published by Elsevier B.V.
更多查看译文
关键词
sensors, data, reduced distance, machine learning, accuracy scores
AI 理解论文
溯源树
样例
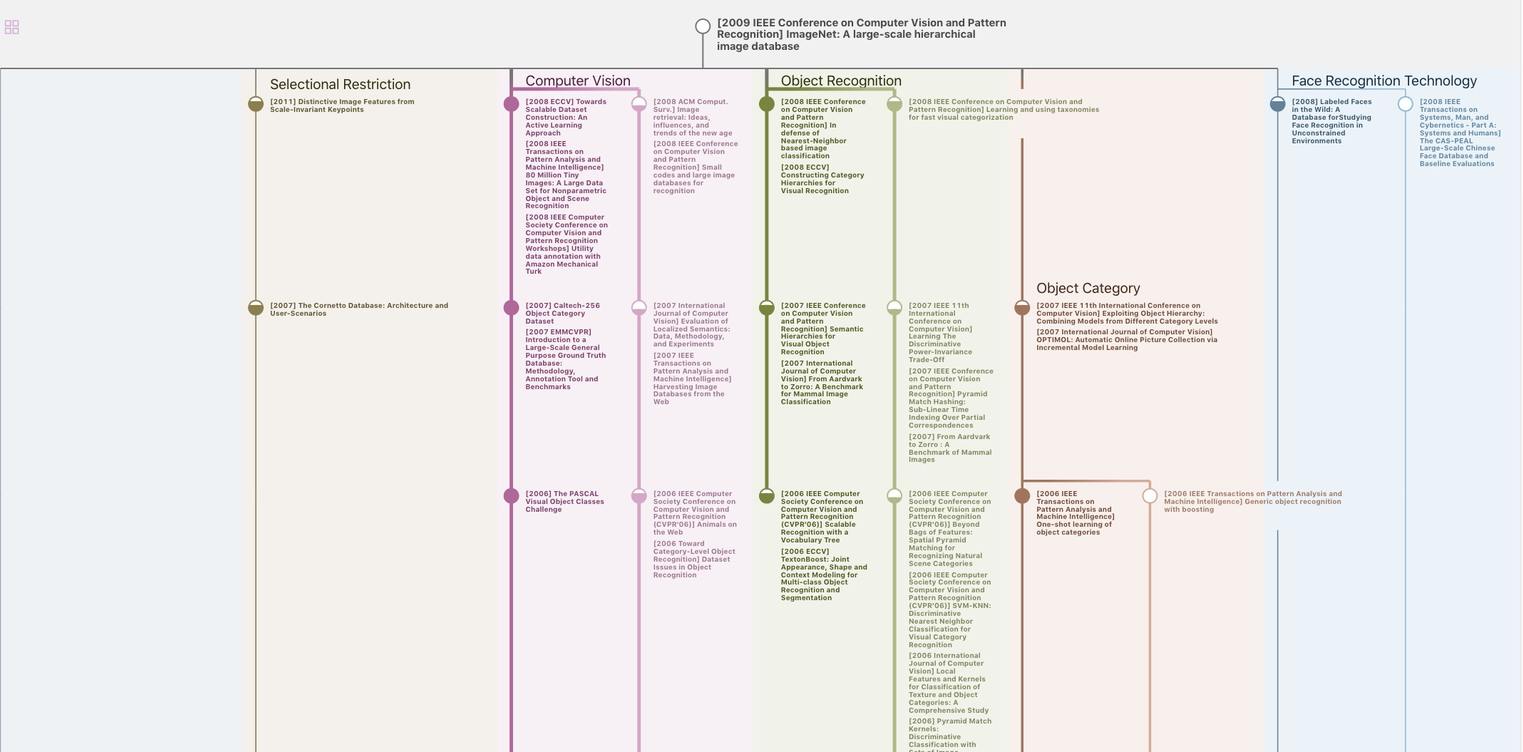
生成溯源树,研究论文发展脉络
Chat Paper
正在生成论文摘要