Input Doubling Method Based On Svr With Rbf Kernel In Clinical Practice: Focus On Small Data
12TH INTERNATIONAL CONFERENCE ON AMBIENT SYSTEMS, NETWORKS AND TECHNOLOGIES (ANT) / THE 4TH INTERNATIONAL CONFERENCE ON EMERGING DATA AND INDUSTRY 4.0 (EDI40) / AFFILIATED WORKSHOPS(2021)
摘要
In recent years, machine-learning-based approaches have become of considerable interest to the efficient processing of short or limited data samples. Its so-called small data approach. This is due to the significant growth of new intellectual analysis tasks in various industries, which are characterized by limited historical data. These include Materials Science, Economics, Medicine, and so on. An effective processing of short datasets is especially acute in medicine. Insufficient number of vectors, significant gaps in the data collected during the supervision of patient's treatment or rehabilitation, reduces the effectiveness or prevents effective intellectual analysis based on them. This paper presents a new approach to processing short medical data samples. The basis of the developed method is SVR with RBF kernel. The algorithmic implementation of the method in both operation modes is described. Experimental modeling on a real short data set (Trabecular bone data) is conducted. It contained only 35 observations. A comparison of the method with a number of existing machine learning methods is conducted. It is experimental established the highest accuracy of the method among those considered. The developed method has potential opportunities for wide application in various fields of medicine. (C) 2021 The Authors. Published by Elsevier B.V.
更多查看译文
关键词
small data approach, machile learning, input doubling method, SVR, rbf-kernel, limited datasets, medical application
AI 理解论文
溯源树
样例
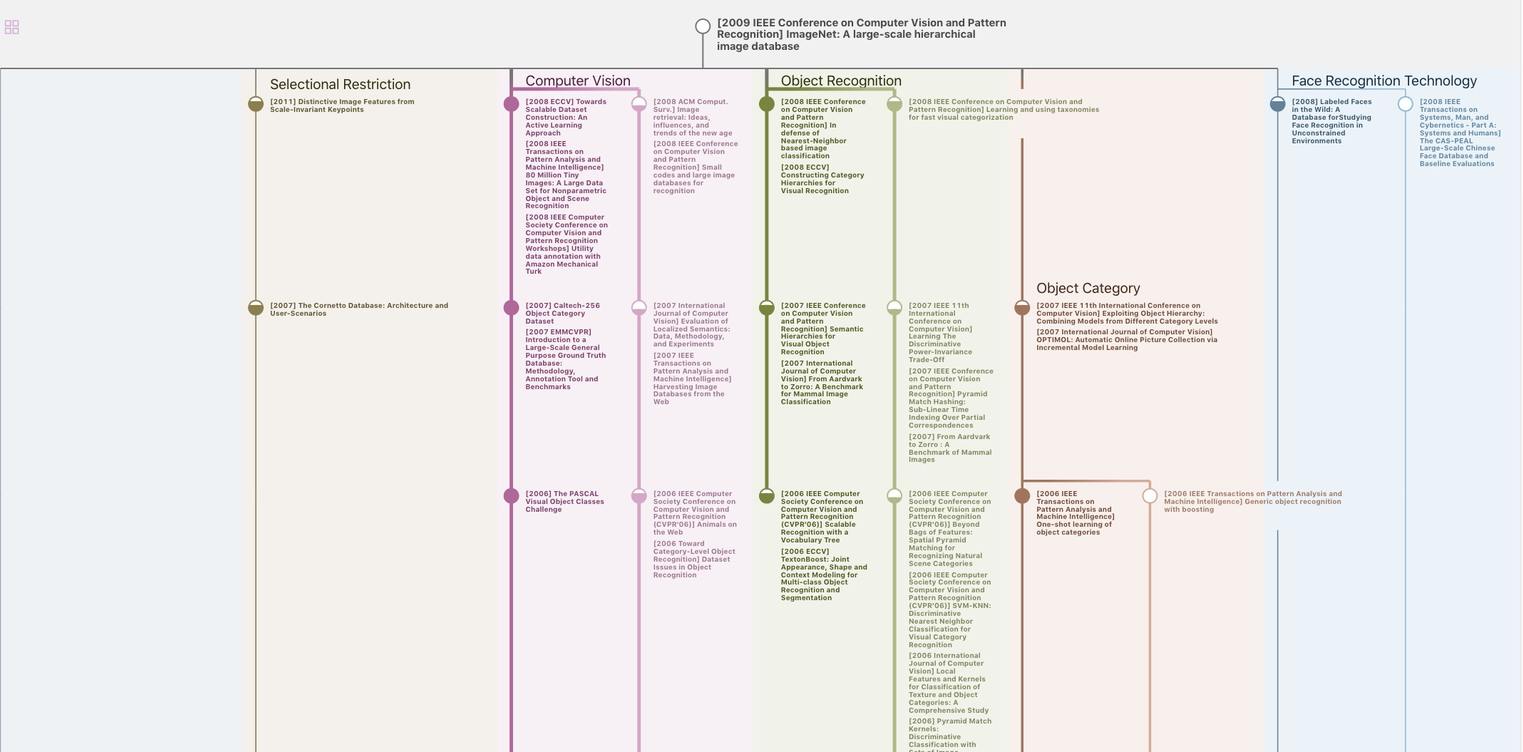
生成溯源树,研究论文发展脉络
Chat Paper
正在生成论文摘要