An Overlapping Community Detection Approach In Ego-Splitting Networks Using Symmetric Nonnegative Matrix Factorization
SYMMETRY-BASEL(2021)
摘要
Overlapping clustering is a fundamental and widely studied subject that identifies all densely connected groups of vertices and separates them from other vertices in complex networks. However, most conventional algorithms extract modules directly from the whole large-scale graph using various heuristics, resulting in either high time consumption or low accuracy. To address this issue, we develop an overlapping community detection approach in Ego-Splitting networks using symmetric Nonnegative Matrix Factorization (ESNMF). It primarily divides the whole network into many sub-graphs under the premise of preserving the clustering property, then extracts the well-connected sub-sub-graph round each community seed as prior information to supplement symmetric adjacent matrix, and finally identifies precise communities via nonnegative matrix factorization in each sub-network. Experiments on both synthetic and real-world networks of publicly available datasets demonstrate that the proposed approach outperforms the state-of-the-art methods for community detection in large-scale networks.
更多查看译文
关键词
overlapping community detection, ego-splitting network, nonnegative matrix factorization, graph symmetry theory, priori information embedding
AI 理解论文
溯源树
样例
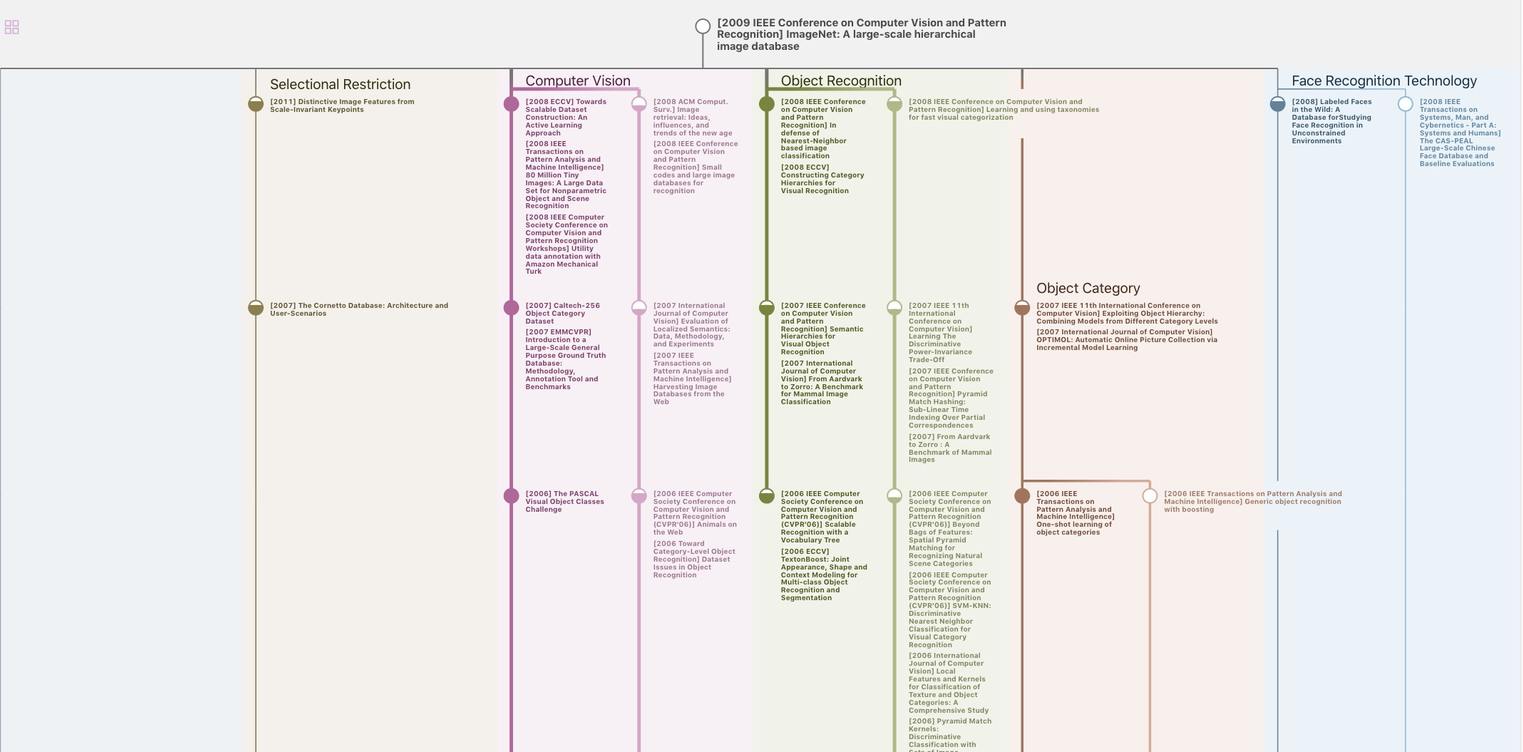
生成溯源树,研究论文发展脉络
Chat Paper
正在生成论文摘要