Unbiased Mean Teacher for Cross-domain Object Detection
2021 IEEE/CVF CONFERENCE ON COMPUTER VISION AND PATTERN RECOGNITION, CVPR 2021(2021)
摘要
Cross-domain object detection is challenging, because object detection model is often vulnerable to data variance, especially to the considerable domain shift between two distinctive domains. In this paper, we propose a new Unbiased Mean Teacher (UMT) model for cross-domain object detection. We reveal that there often exists a considerable model bias for the simple mean teacher (MT) model in cross-domain scenarios, and eliminate the model bias with several simple yet highly effective strategies. In particular, for the teacher model, we propose a cross-domain distillation method for MT to maximally exploit the expertise of the teacher model. Moreover, for the student model, we alleviate its bias by augmenting training samples with pixel-level adaptation. Finally, for the teaching process, we employ an out-of-distribution estimation strategy to select samples that most fit the current model to further enhance the cross-domain distillation process. By tackling the model bias issue with these strategies, our UMT model achieves mAPs of 44.1%, 58.1%, 41.7%, and 43.1% on benchmark datasets Clipart1k, Watercolor2k, Foggy Cityscapes, and Cityscapes, respectively, which outperforms the (listing state-of-the-art results in notable margins. Our implementation is available at hups://github.com/kinredon/umt.
更多查看译文
关键词
distinctive domains,cross-domain object detection,cross-domain distillation method,student model,UMT model,unbiased mean teacher model
AI 理解论文
溯源树
样例
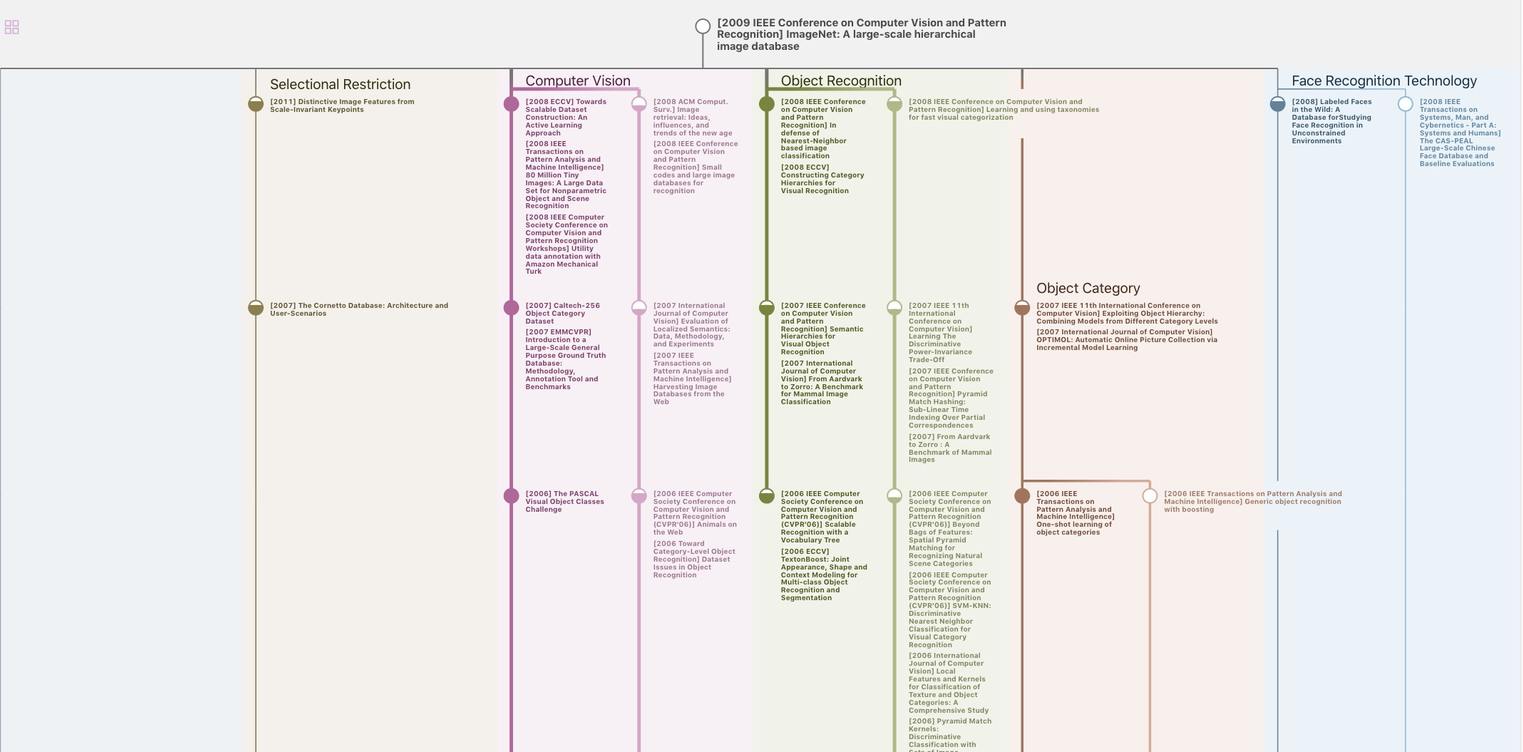
生成溯源树,研究论文发展脉络
Chat Paper
正在生成论文摘要