LayoutTransformer: Scene Layout Generation with Conceptual and Spatial Diversity
2021 IEEE/CVF CONFERENCE ON COMPUTER VISION AND PATTERN RECOGNITION, CVPR 2021(2021)
摘要
When translating text inputs into layouts or images, existing works typically require explicit descriptions of each object in a scene, including their spatial information or the associated relationships. To better exploit the text input, so that implicit objects or relationships can be properly inferred during layout generation, we propose a LayoutTransformer Network (LT-Net) in this paper. Given a scene-graph input, our LT-Net uniquely encodes the semantic features for exploiting their co-occurrences and implicit relationships. This allows one to manipulate conceptually diverse yet plausible layout outputs. Moreover, the decoder of our LT-Net translates the encoded contextual features into bounding boxes with self-supervised relation consistency preserved. By fitting their distributions to Gaussian mixture models, spatially-diverse layouts can be additionally produced by LT-Net. We conduct extensive experiments on the datasets of MS-COCO and Visual Genome, and confirm the effectiveness and plausibility of our LT-Net over recent layout generation models. Codes will be released at LaynaTransformer.
更多查看译文
关键词
spatial diversity,translating text,explicit descriptions,spatial information,associated relationships,text input,implicit objects,scene-graph input,implicit relationships,plausible layout outputs,LT-Net translates,encoded contextual features,spatially-diverse layouts,layout generation models,LayoutTransformer network
AI 理解论文
溯源树
样例
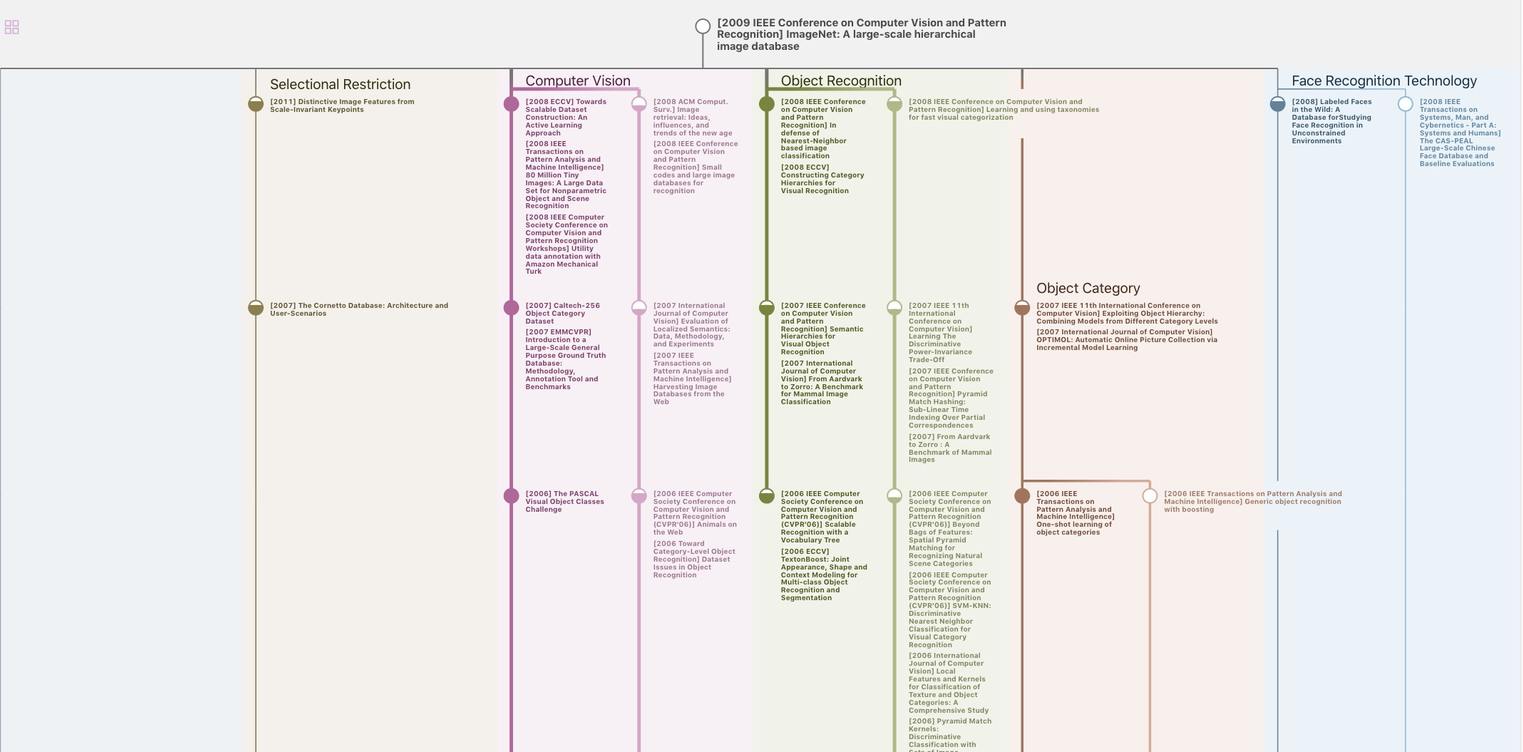
生成溯源树,研究论文发展脉络
Chat Paper
正在生成论文摘要