Quality-Agnostic Image Recognition via Invertible Decoder
2021 IEEE/CVF CONFERENCE ON COMPUTER VISION AND PATTERN RECOGNITION, CVPR 2021(2021)
摘要
Despite the remarkable performance of deep models on image recognition tasks, they are known to be susceptible to common corruptions such as blur, noise, and low-resolution. Data augmentation is a conventional way to build a robust model by considering these common corruptions during the training. However, a naive data augmentation scheme may result in a non-specialized model for particular corruptions, as the model tends to learn the averaged distribution among corruptions. To mitigate the issue, we propose a new paradigm of training deep image recognition networks that produce clean-like features from any quality image via an invertible neural architecture. The proposed method consists of two stages. In the first stage, we train an invertible network with only clean images under the recognition objective. In the second stage, its inversion, i.e., the invertible decoder, is attached to a new recognition network and we train this encoder-decoder network using both clean and corrupted images by considering recognition and reconstruction objectives. Our two-stage scheme allows the network to produce clean-like and robust features from any quality images, by reconstructing their clean images via the invertible decoder. We demonstrate the effectiveness of our method on image classification and face recognition tasks.
更多查看译文
关键词
image recognition tasks,naive data augmentation scheme,training deep image recognition networks,quality image,invertible neural architecture,recognition objective,recognition network,encoder-decoder network,corrupted images,image classification,face recognition tasks,quality-agnostic image recognition,deep models
AI 理解论文
溯源树
样例
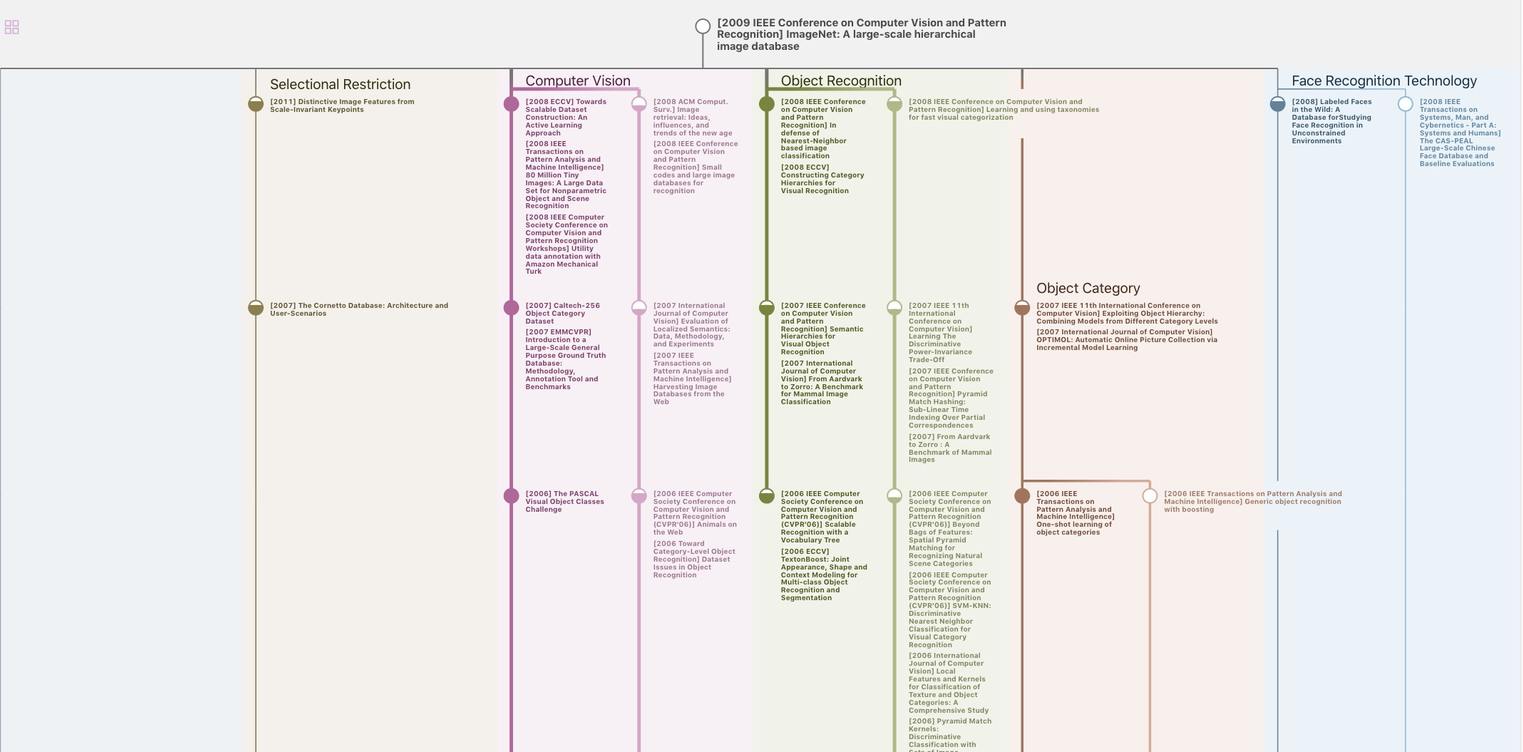
生成溯源树,研究论文发展脉络
Chat Paper
正在生成论文摘要