Learning 3D Shape Feature for Texture-insensitive Person Re-identification
2021 IEEE/CVF CONFERENCE ON COMPUTER VISION AND PATTERN RECOGNITION, CVPR 2021(2021)
摘要
It is well acknowledged that person re-identification (person ReID) highly relies on visual texture information like clothing. Despite significant progress has been made in recent years, texture-confusing situations like clothing changing and persons wearing the same clothes receive little attention from most existing ReID methods. In this paper, rather than relying on texture based information, we propose to improve the robustness of person ReID against clothing texture by exploiting the information of a person’s 3D shape. Existing shape learning schemas for person ReID either ignore the 3D information of a person, or require extra physical devices to collect 3D source data. Differently, we propose a novel ReID learning framework that directly extracts a texture-insensitive 3D shape embedding from a 2D image by adding 3D body reconstruction as an auxiliary task and regularization, called 3D Shape Learning (3DSL). The 3D reconstruction based regularization forces the ReID model to decouple the 3D shape information from the visual texture, and acquire discriminative 3D shape ReID features. To solve the problem of lacking 3D ground truth, we design an adversarial self-supervised projection (ASSP) model, performing 3D reconstruction without ground truth. Extensive experiments on common ReID datasets and texture-confusing datasets validate the effectiveness of our model.
更多查看译文
关键词
3D shape learning,3D reconstruction based regularization,ReID model,3D shape information,discriminative 3D shape ReID features,texture-confusing datasets,3D shape feature,texture-insensitive person,person ReID,visual texture information,texture-confusing situations,texture based information,clothing texture,3D source data,texture-insensitive 3D shape,3D body reconstruction,ReID learning framework
AI 理解论文
溯源树
样例
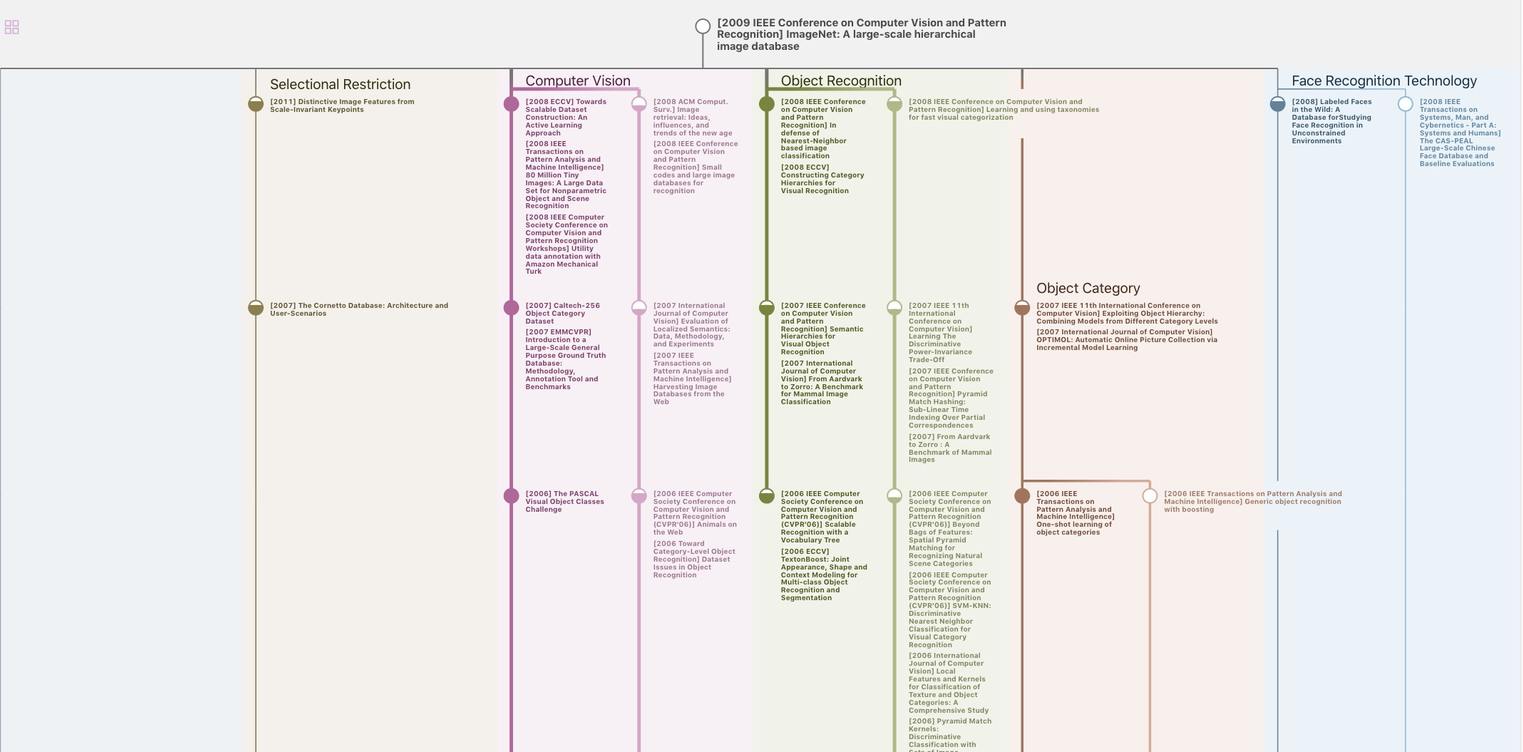
生成溯源树,研究论文发展脉络
Chat Paper
正在生成论文摘要