Stay Positive: Non-Negative Image Synthesis for Augmented Reality
2021 IEEE/CVF CONFERENCE ON COMPUTER VISION AND PATTERN RECOGNITION, CVPR 2021(2021)
摘要
In applications such as optical see-through and projector augmented reality, producing images amounts to solving non-negative image generation, where one can only add light to an existing image. Most image generation methods, however, are ill-suited to this problem setting, as they make the assumption that one can assign arbitrary color to each pixel. In fact, naive application of existing methods fails even in simple domains such as MNIST digits, since one cannot create darker pixels by adding light. We know, however, that the human visual system can be fooled by optical illusions involving certain spatial configurations of brightness and contrast. Our key insight is that one can leverage this behavior to produce high quality images with negligible artifacts. For example, we can create the illusion of darker patches by brightening surrounding pixels. We propose a novel optimization procedure to produce images that satisfy both semantic and non-negativity constraints. Our approach can incorporate existing state-of-the-art methods, and exhibits strong performance in a variety of tasks including image-to-image translation and style transfer.
更多查看译文
关键词
style transfer,stay positive,nonnegative image synthesis,augmented reality,images amounts,solving nonnegative image generation,existing image,image generation methods,arbitrary color,naive application,simple domains,MNIST digits,darker pixels,adding light,human visual system,optical illusions,high quality images,surrounding pixels,nonnegativity constraints,image-to-image translation
AI 理解论文
溯源树
样例
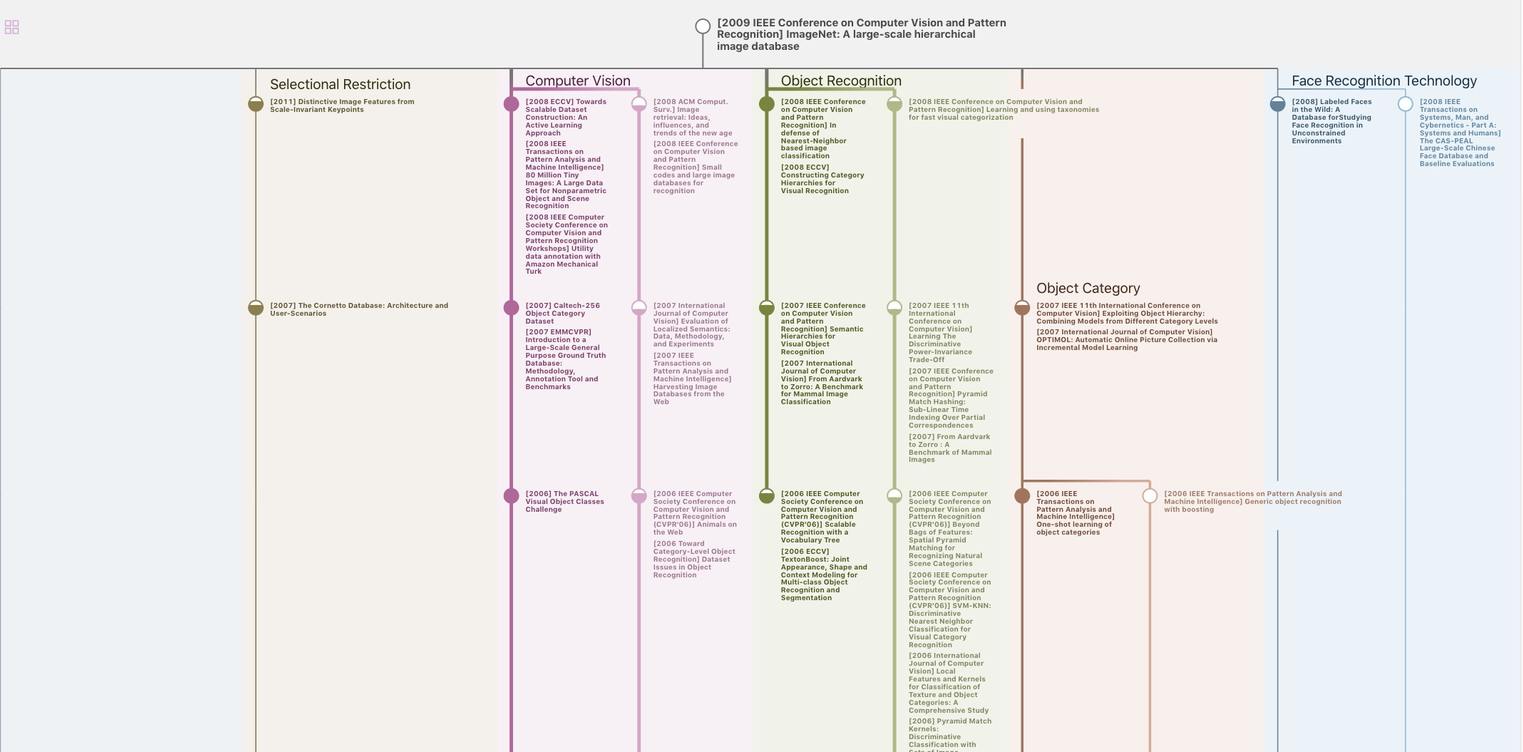
生成溯源树,研究论文发展脉络
Chat Paper
正在生成论文摘要