Very Deep Graph Neural Networks Via Noise Regularisation
arxiv(2021)
摘要
Graph Neural Networks (GNNs) perform learned message passing over an input graph, but conventional wisdom says performing more than handful of steps makes training difficult and does not yield improved performance. Here we show the contrary. We train a deep GNN with up to 100 message passing steps and achieve several state-of-the-art results on two challenging molecular property prediction benchmarks, Open Catalyst 2020 IS2RE and QM9. Our approach depends crucially on a novel but simple regularisation method, which we call ``Noisy Nodes'', in which we corrupt the input graph with noise and add an auxiliary node autoencoder loss if the task is graph property prediction. Our results show this regularisation method allows the model to monotonically improve in performance with increased message passing steps. Our work opens new opportunities for reaping the benefits of deep neural networks in the space of graph and other structured prediction problems.
更多查看译文
关键词
3d molecular property prediction,simple gnn regularisation
AI 理解论文
溯源树
样例
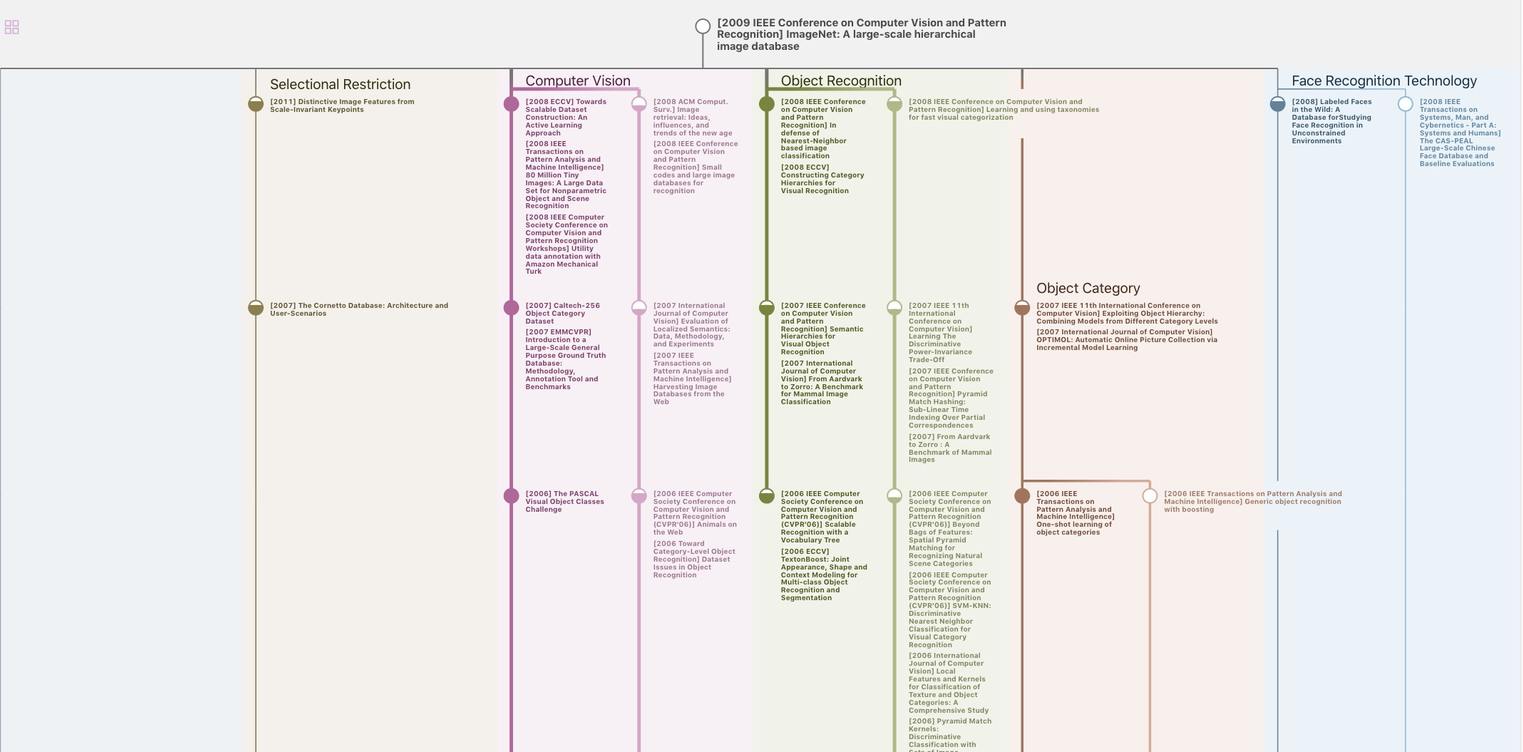
生成溯源树,研究论文发展脉络
Chat Paper
正在生成论文摘要