Learning to Bridge Metric Spaces - Few-shot Joint Learning of Intent Detection and Slot Filling.
ACL/IJCNLP(2021)
摘要
In this paper, we investigate few-shot joint learning for dialogue language understanding. Most existing few-shot models learn a single task each time with only a few examples. However, dialogue language understanding contains two closely related tasks, i.e., intent detection and slot filling, and often benefits from jointly learning the two tasks. This calls for new few-shot learning techniques that are able to capture task relations from only a few examples and jointly learn multiple tasks. To achieve this, we propose a similarity-based few-shot learning scheme, named Contrastive Prototype Merging network (ConProm), that learns to bridge metric spaces of intent and slot on data-rich domains, and then adapt the bridged metric space to the specific few-shot domain. Experiments on two public datasets, Snips and FewJoint, show that our model significantly outperforms the strong baselines in one and five shots settings.
更多查看译文
关键词
intent
AI 理解论文
溯源树
样例
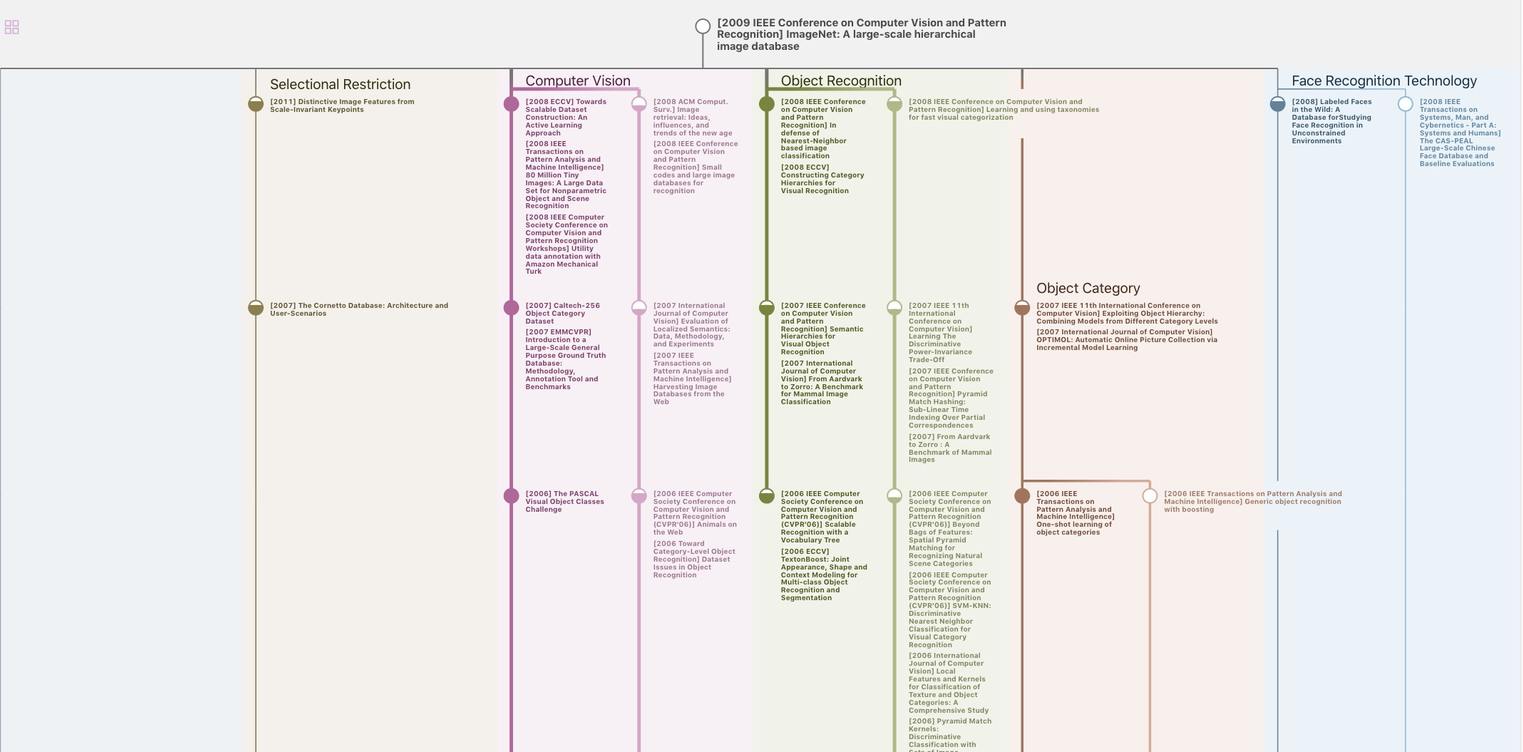
生成溯源树,研究论文发展脉络
Chat Paper
正在生成论文摘要