Deep Bayesian Unsupervised Lifelong Learning
Neural Networks(2022)
摘要
Lifelong Learning (LL) refers to the ability to continually learn and solve new problems with incremental available information over time while retaining previous knowledge. Much attention has been given lately to Supervised Lifelong Learning (SLL) with a stream of labelled data. In contrast, we focus on resolving challenges in Unsupervised Lifelong Learning (ULL) with streaming unlabelled data when the data distribution and the unknown class labels evolve over time. Bayesian framework is natural to incorporate past knowledge and sequentially update the belief with new data. We develop a fully Bayesian inference framework for ULL with a novel end-to-end Deep Bayesian Unsupervised Lifelong Learning (DBULL) algorithm, which can progressively discover new clusters without forgetting the past with unlabelled data while learning latent representations. To efficiently maintain past knowledge, we develop a novel knowledge preservation mechanism via sufficient statistics of the latent representation for raw data. To detect the potential new clusters on the fly, we develop an automatic cluster discovery and redundancy removal strategy in our inference inspired by Nonparametric Bayesian statistics techniques. We demonstrate the effectiveness of our approach using image and text corpora benchmark datasets in both LL and batch settings.
更多查看译文
关键词
Unsupervised Lifelong Learning,Bayesian Learning,Deep generative models,Deep Neural Networks,Sufficient statistics
AI 理解论文
溯源树
样例
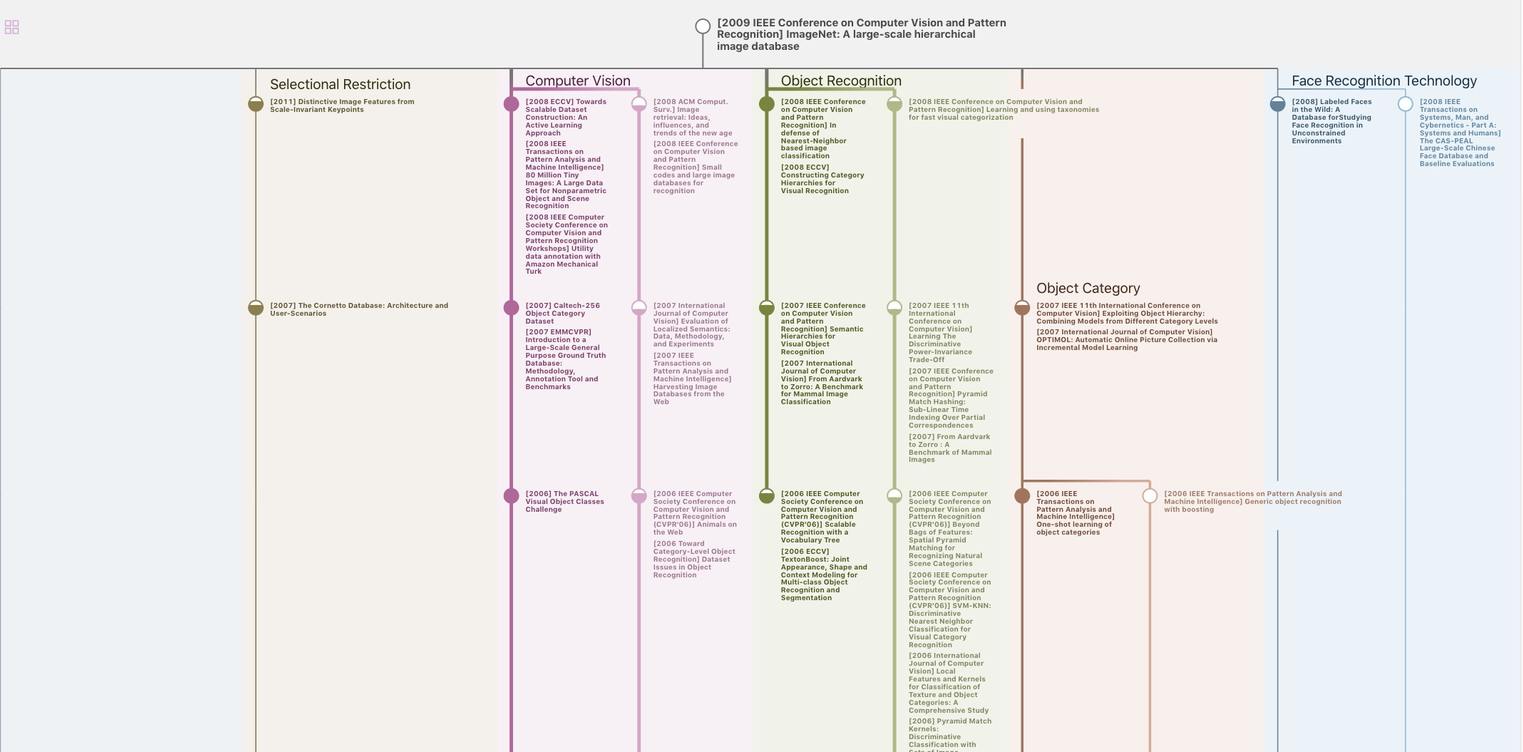
生成溯源树,研究论文发展脉络
Chat Paper
正在生成论文摘要