Feedback Pyramid Attention Networks for Single Image Super-Resolution
arxiv(2023)
摘要
Recently, convolutional neural network (CNN) based image super-resolution (SR) methods have achieved significant performance improvement. However, most CNN-based methods mainly focus on feed-forward architecture design and neglect to explore the feedback mechanism, which usually exists in the human visual system. In this paper, we propose feedback pyramid attention networks (FPAN) to fully exploit the mutual dependencies of features. Specifically, a novel feedback connection structure is developed to enhance low-level feature expression with high-level information. In our method, the output of each layer in the first stage is also used as the input of the corresponding layer in the next state to re-update the previous low-level filters. Moreover, we introduce a pyramid non-local structure to model global contextual information in different scales and improve the discriminative representation of the network. Extensive experimental results on various datasets demonstrate the superiority of our FPAN in comparison with the state-of-the-art SR methods.
更多查看译文
关键词
attention,networks,feedback,image,super-resolution
AI 理解论文
溯源树
样例
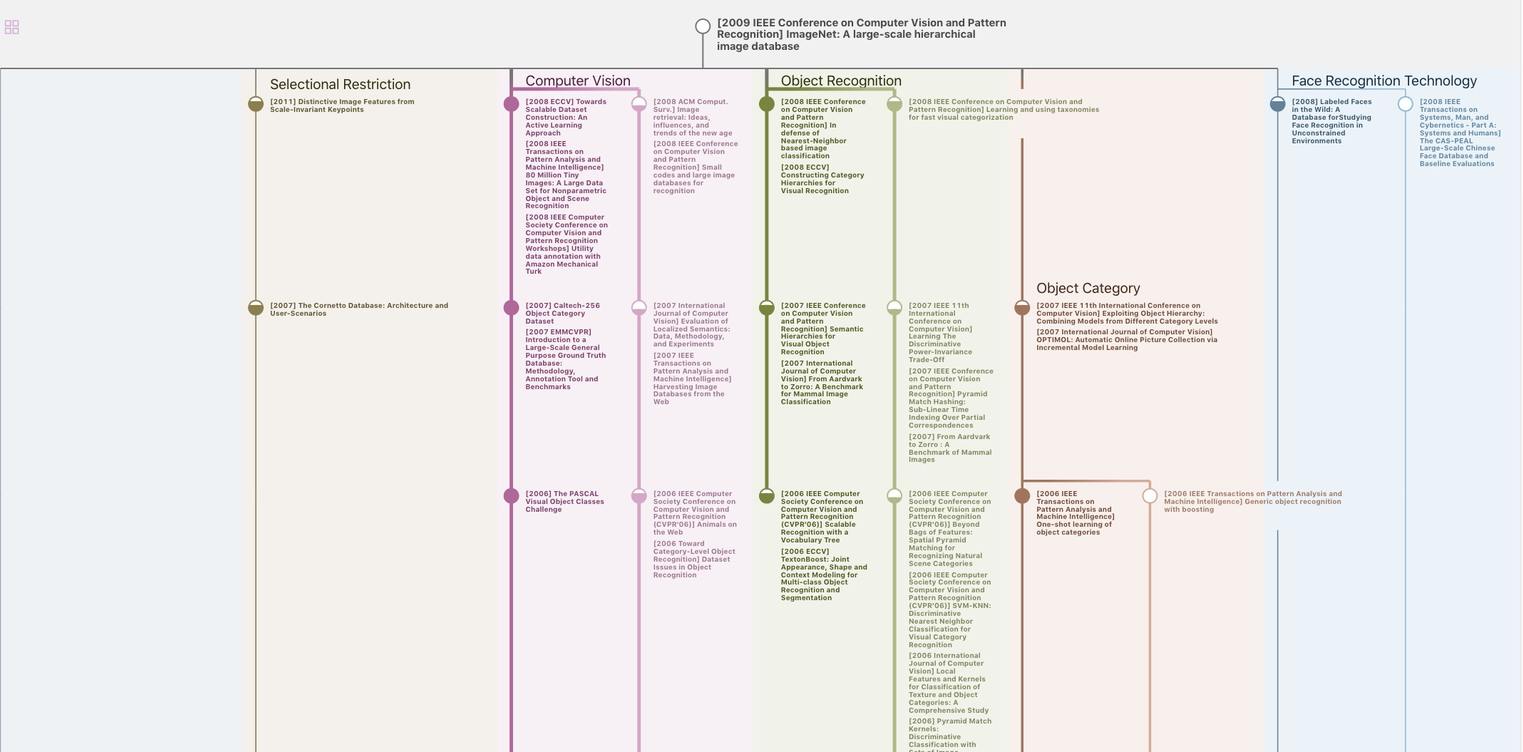
生成溯源树,研究论文发展脉络
Chat Paper
正在生成论文摘要