Contrastive Attention for Automatic Chest X-ray Report Generation.
ACL/IJCNLP(2021)
摘要
Recently, chest X-ray report generation, which aims to automatically generate descriptions of given chest X-ray images, has received growing research interests. The key challenge of chest X-ray report generation is to accurately capture and describe the abnormal regions. In most cases, the normal regions dominate the entire chest X-ray image, and the corresponding descriptions of these normal regions dominate the final report. Due to such data bias, learning-based models may fail to attend to abnormal regions. In this work, to effectively capture and describe abnormal regions, we propose the Contrastive Attention (CA) model. Instead of solely focusing on the current input image, the CA model compares the current input image with normal images to distill the contrastive information. The acquired contrastive information can better represent the visual features of abnormal regions. According to the experiments on the public IU-X-ray and MIMIC-CXR datasets, incorporating our CA into several existing models can boost their performance across most metrics. In addition, according to the analysis, the CA model can help existing models better attend to the abnormal regions and provide more accurate descriptions which are crucial for an interpretable diagnosis. Specifically, we achieve the state-of-the-art results on the two public datasets.
更多查看译文
关键词
contrastive attention,report,x-ray
AI 理解论文
溯源树
样例
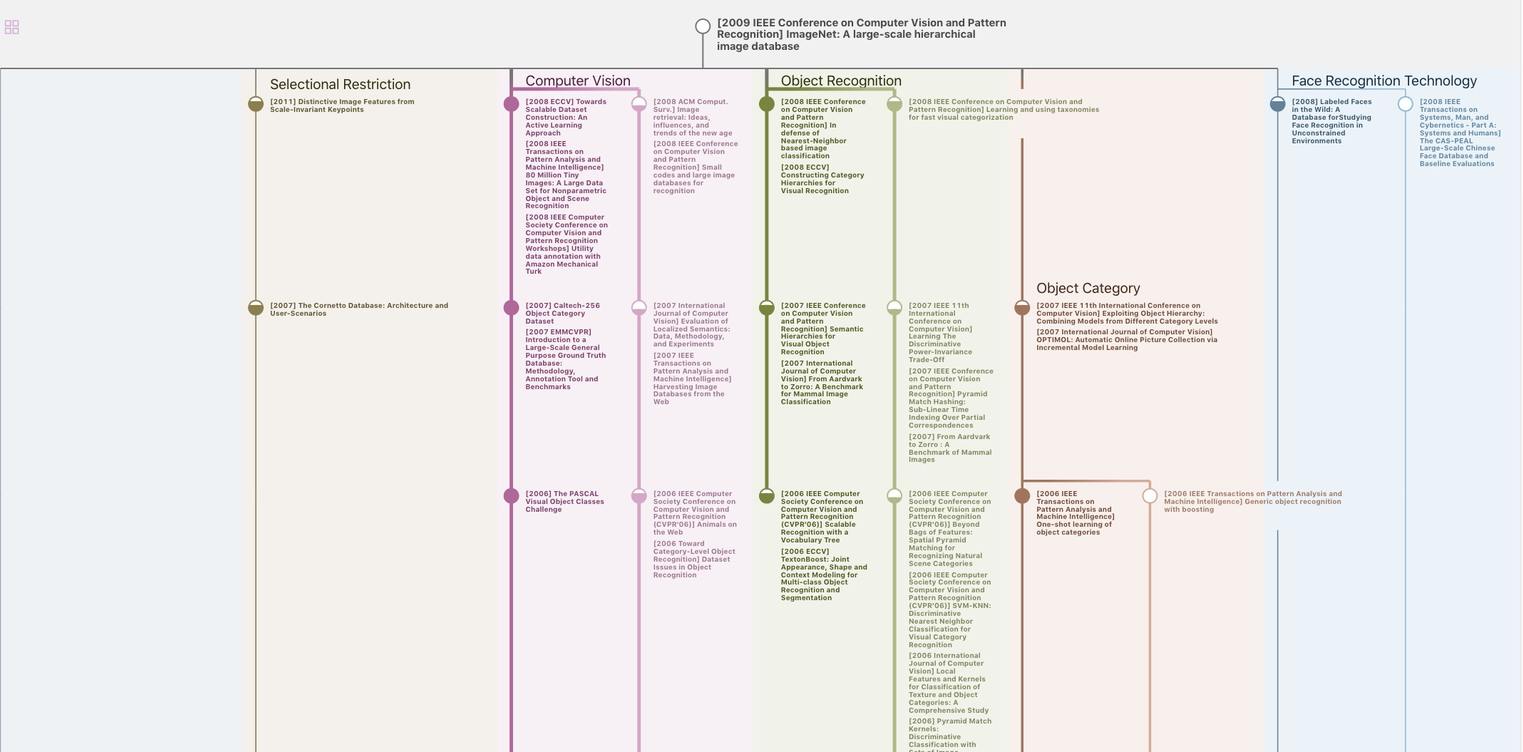
生成溯源树,研究论文发展脉络
Chat Paper
正在生成论文摘要