Cross-sentence Neural Language Models for Conversational Speech Recognition
2021 INTERNATIONAL JOINT CONFERENCE ON NEURAL NETWORKS (IJCNN)(2021)
摘要
An important research direction in automatic speech recognition (ASR) has centered around the development of effective methods to rerank the output hypotheses of an ASR system with more sophisticated language models (LMs) for further gains. A current mainstream school of thoughts for ASR N-best hypothesis reranking is to employ a recurrent neural network (RNN)-based LM or its variants, with performance superiority over the conventional n-gram LMs across a range of ASR tasks. In real scenarios such as a long conversation, a sequence of consecutive sentences may jointly contain ample cues of conversation-level information such as topical coherence, lexical entrainment and adjacency pairs, which however remains to be underexplored. In view of this, we first formulate ASR N-best reranking as a prediction problem, putting forward an effective cross-sentence neural LM approach that reranks the ASR N-best hypotheses of an upcoming sentence by taking into consideration the word usage in its precedent sentences. Furthermore, we also explore to extract task-specific global topical information of the cross-sentence history in an unsupervised manner for better ASR performance. Extensive experiments conducted on the AMI conversational benchmark corpus indicate the effectiveness and feasibility of our methods in comparison to several state-of-the-art reranking methods.
更多查看译文
关键词
automatic speech recognition, language models, conversational language modeling, cross-sentence, N-best hypothesis reranking, BERT
AI 理解论文
溯源树
样例
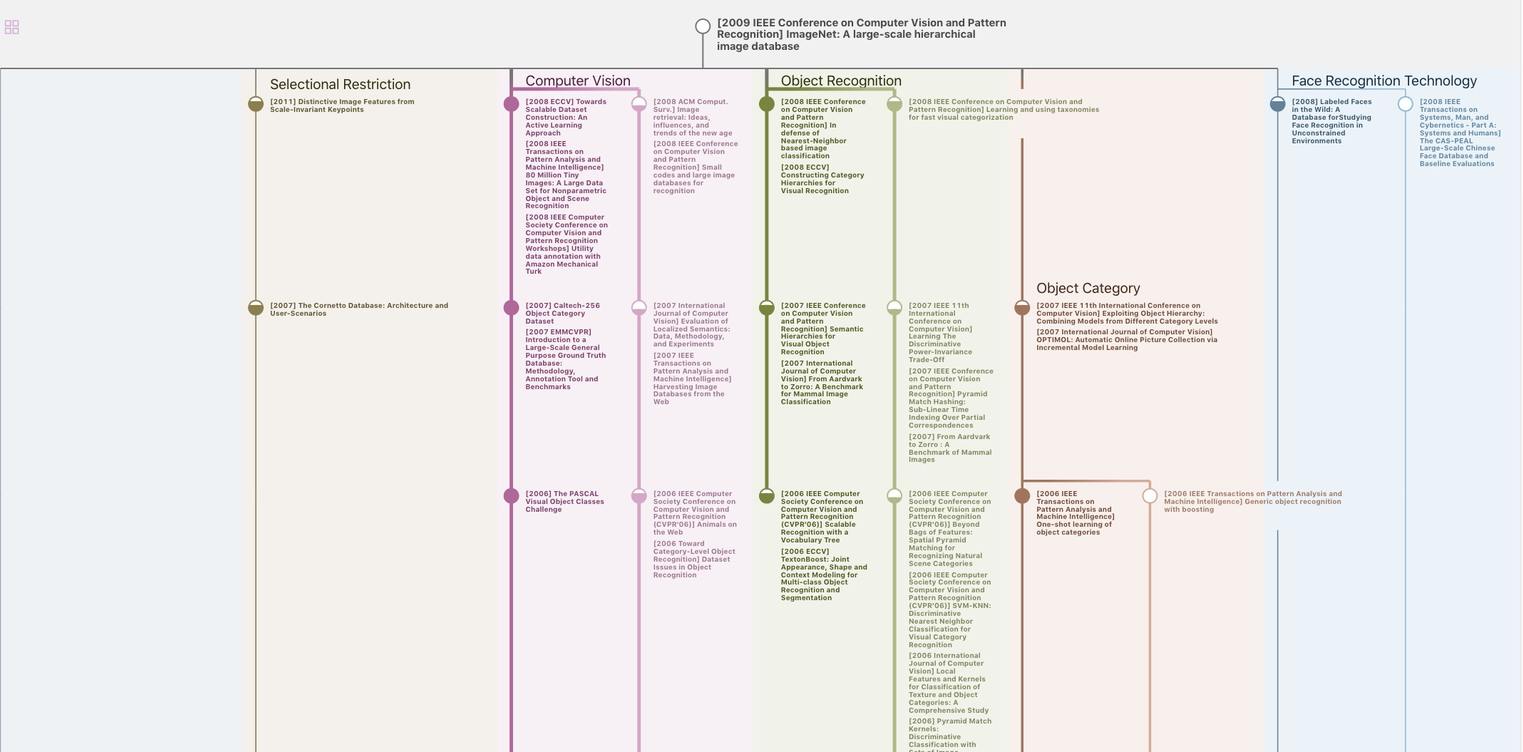
生成溯源树,研究论文发展脉络
Chat Paper
正在生成论文摘要