Adversarial Robustness Via Fisher-Rao Regularization
IEEE Transactions on Pattern Analysis and Machine Intelligence(2023)
摘要
Adversarial robustness has become a topic of growing interest in machine learning since it was observed that neural networks tend to be brittle. We propose an information-geometric formulation of adversarial defense and introduce
Fire
, a new Fisher-Rao regularization for the categorical cross-entropy loss, which is based on the geodesic distance between the softmax outputs corresponding to natural and perturbed input features. Based on the information-geometric properties of the class of softmax distributions, we derive an explicit characterization of the Fisher-Rao Distance (
FRD
) for the binary and multiclass cases, and draw some interesting properties as well as connections with standard regularization metrics. Furthermore, we verify on a simple linear and Gaussian model, that all Pareto-optimal points in the accuracy-robustness region can be reached by
Fire
while other state-of-the-art methods fail. Empirically, we evaluate the performance of various classifiers trained with the proposed loss on standard datasets, showing up to a simultaneous 1% of improvement in terms of clean and robust performances while reducing the training time by 20% over the best-performing methods.
更多查看译文
关键词
Adversarial regularization,adversarial training,computer vision,deep learning,fisher-rao distance,information geometry,neural networks,safety AI
AI 理解论文
溯源树
样例
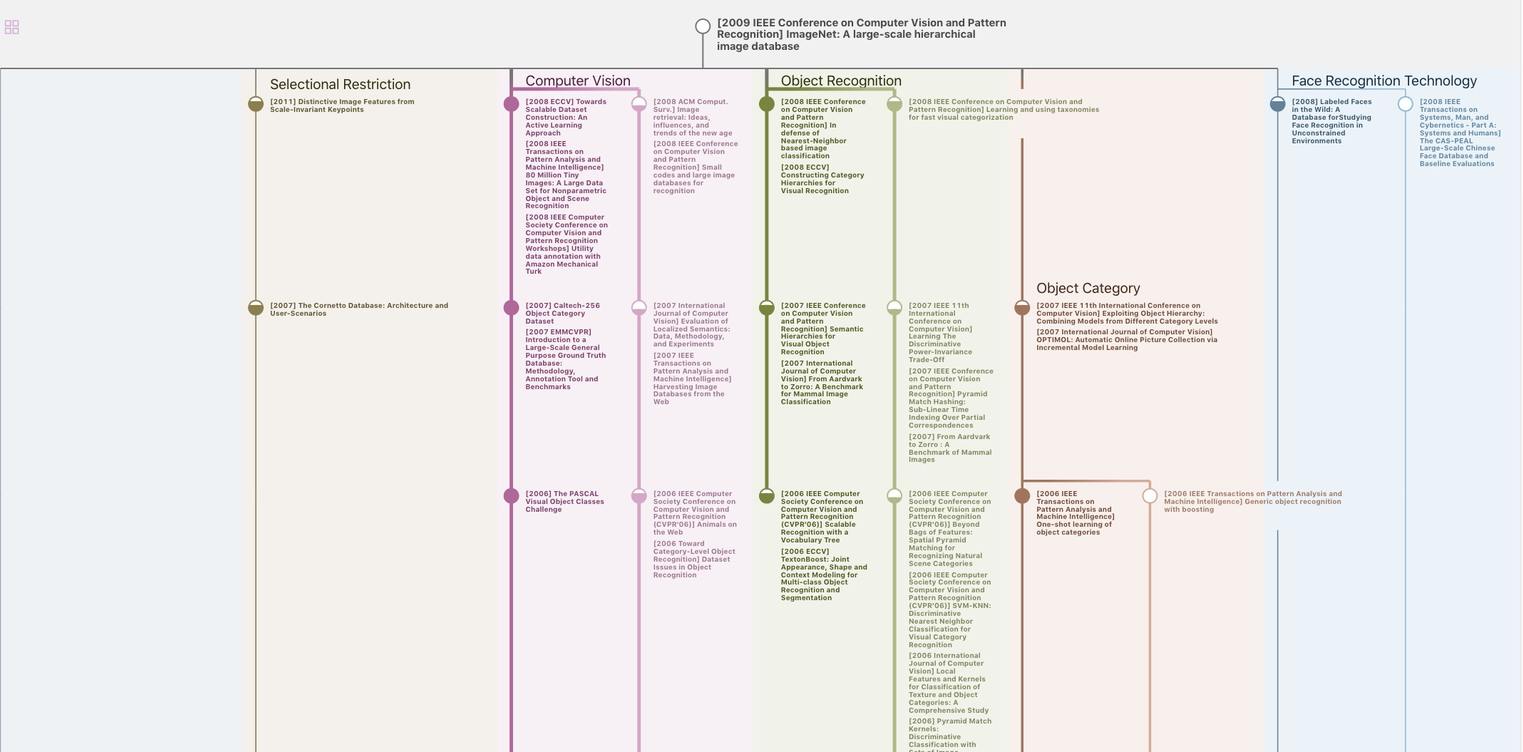
生成溯源树,研究论文发展脉络
Chat Paper
正在生成论文摘要