Understanding Deflation Process in Over-parametrized Tensor Decomposition.
Annual Conference on Neural Information Processing Systems(2021)
摘要
In this paper we study the training dynamics for gradient flow on over-parametrized tensor decomposition problems. Empirically, such training process often first fits larger components and then discovers smaller components, which is similar to a tensor deflation process that is commonly used in tensor decomposition algorithms. We prove that for orthogonally decomposable tensor, a slightly modified version of gradient flow would follow a tensor deflation process and recover all the tensor components. Our proof suggests that for orthogonal tensors, gradient flow dynamics works similarly as greedy low-rank learning in the matrix setting, which is a first step towards understanding the implicit regularization effect of over-parametrized models for low-rank tensors.
更多查看译文
关键词
deflation process,tensor,decomposition,over-parametrized
AI 理解论文
溯源树
样例
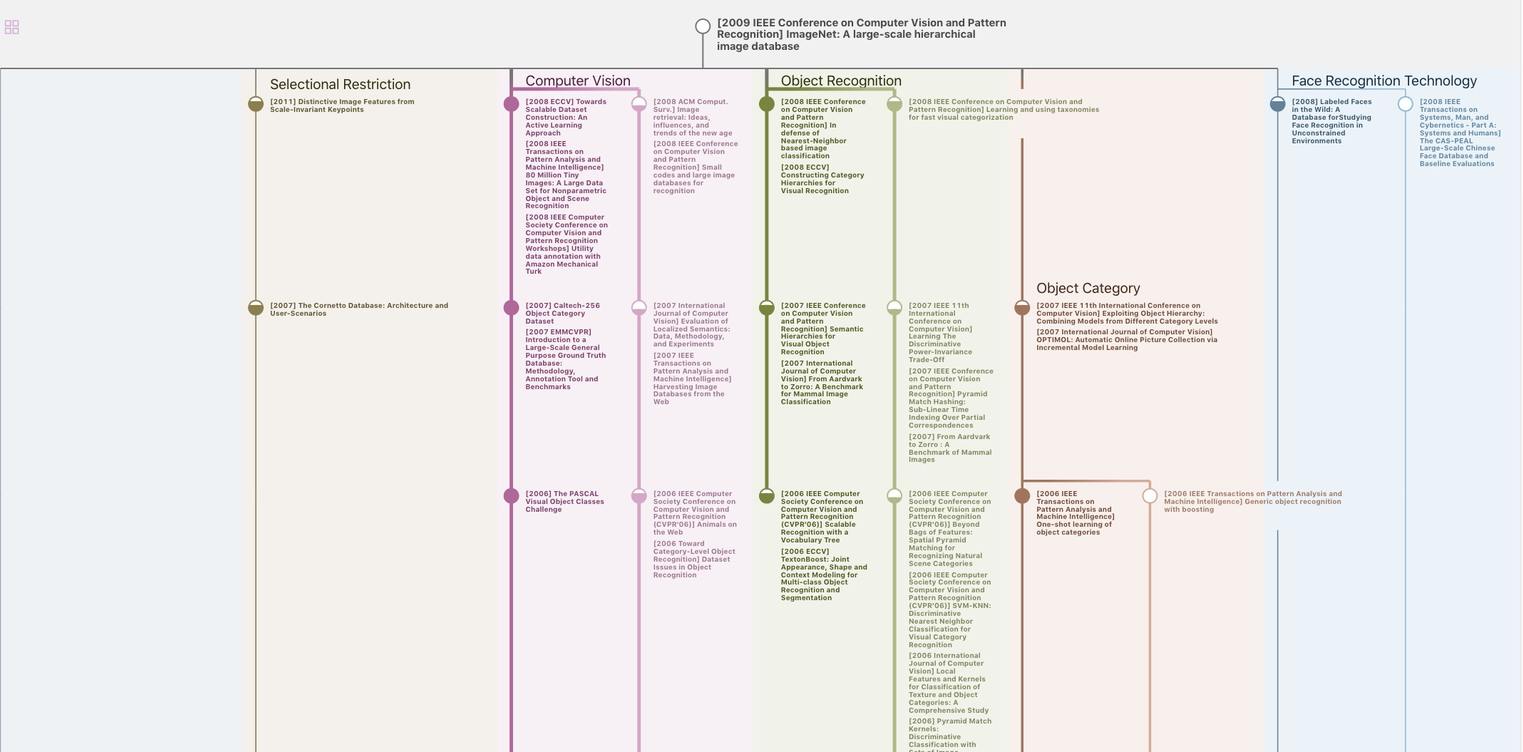
生成溯源树,研究论文发展脉络
Chat Paper
正在生成论文摘要