Detecting climate signals in populations across life histories
GLOBAL CHANGE BIOLOGY(2022)
摘要
Climate impacts are not always easily discerned in wild populations as detecting climate change signals in populations is challenged by stochastic noise associated with natural climate variability, variability in biotic and abiotic processes, and observation error in demographic rates. Detection of the impact of climate change on populations requires making a formal distinction between signals in the population associated with long-term climate trends from those generated by stochastic noise. The time of emergence (ToE) identifies when the signal of anthropogenic climate change can be quantitatively distinguished from natural climate variability. This concept has been applied extensively in the climate sciences, but has not been explored in the context of population dynamics. Here, we outline an approach to detecting climate-driven signals in populations based on an assessment of when climate change drives population dynamics beyond the envelope characteristic of stochastic variations in an unperturbed state. Specifically, we present a theoretical assessment of the time of emergence of climate-driven signals in population dynamics (ToEpop). We identify the dependence of ToEpop on the magnitude of both trends and variability in climate and also explore the effect of intrinsic demographic controls on ToEpop. We demonstrate that different life histories (fast species vs. slow species), demographic processes (survival, reproduction), and the relationships between climate and demographic rates yield population dynamics that filter climate trends and variability differently. We illustrate empirically how to detect the point in time when anthropogenic signals in populations emerge from stochastic noise for a species threatened by climate change: the emperor penguin. Finally, we propose six testable hypotheses and a road map for future research.
更多查看译文
关键词
climate change, emperor penguin, life histories, population trend, population variability, signal to noise, time of emergence
AI 理解论文
溯源树
样例
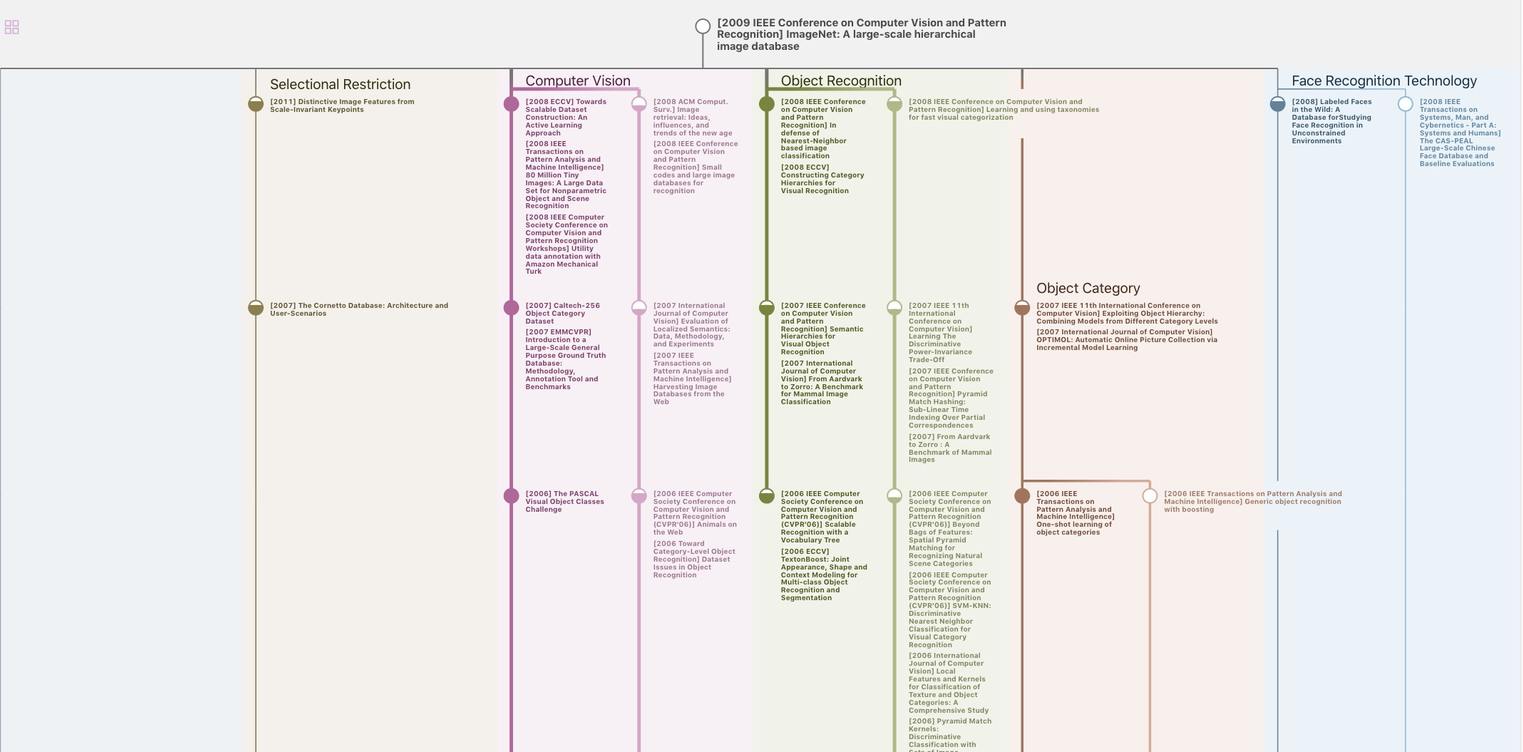
生成溯源树,研究论文发展脉络
Chat Paper
正在生成论文摘要