Deep Hashing with Hash Center Update for Efficient Image Retrieval
IEEE International Conference on Acoustics, Speech, and Signal Processing (ICASSP)(2022)
摘要
In this paper, we propose an approach for learning binary hash codes for image retrieval. Canonical Correlation Analysis (CCA) is used to design two loss functions for training a neural network such that the correlation between the two views to CCA is maximized. The first loss, maximizes the correlation between the hash centers and learned hash codes. The second loss maximizes the correlation between the class labels and classification scores. A novel weighted mean and thresholding based hash center update scheme is proposed for adapting the hash centers in each epoch. The training loss reaches the theoretical lower bound of the proposed loss functions, showing that the correlation coefficients are maximized during training and substantiating the formation of an efficient feature space for image retrieval. The measured mean average precision shows that the proposed approach outperforms other state-of-the-art approaches in both single-labeled and multi-labeled image datasets.
更多查看译文
关键词
Binary hashing,Hash center update,Canonical Correlation Analysis,Image Retrieval
AI 理解论文
溯源树
样例
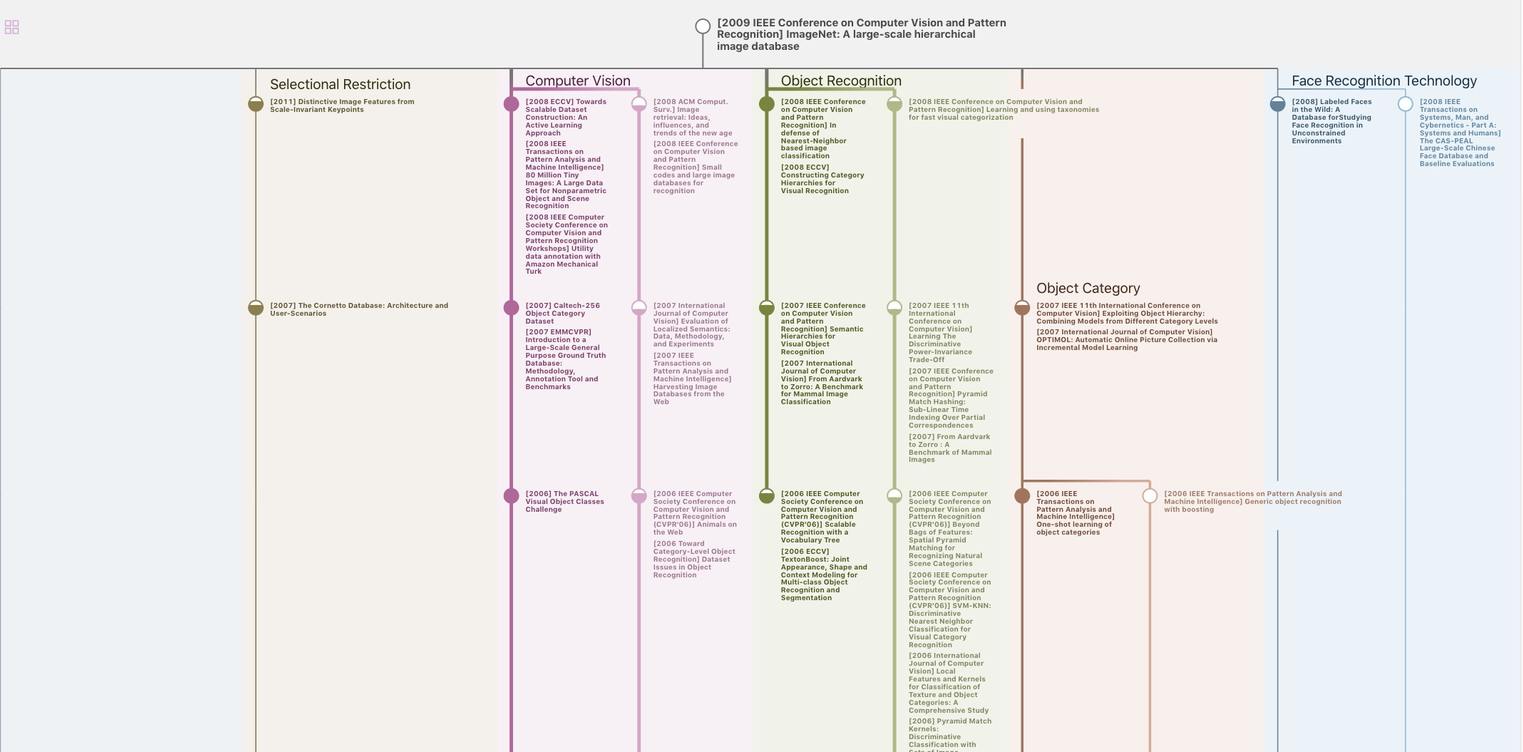
生成溯源树,研究论文发展脉络
Chat Paper
正在生成论文摘要