Statistical modeling of on-street parking lot occupancy in smart cities
arxiv(2021)
摘要
Many studies suggest that searching for parking is associated with significant direct and indirect costs. Therefore, it is appealing to reduce the time which car drivers spend on finding an available parking lot, especially in urban areas where the space for all road users is limited. The prediction of on-street parking lot occupancy can provide drivers a guidance where clear parking lots are likely to be found. This field of research has gained more and more attention in the last decade through the increasing availability of real-time parking lot occupancy data. In this paper, we pursue a statistical approach for the prediction of parking lot occupancy, where we make use of time to event models and semi-Markov process theory. The latter involves the employment of Laplace transformations as well as their inversion which is an ambitious numerical task. We apply our methodology to data from the City of Melbourne in Australia. Our main result is that the semi-Markov model outperforms a Markov model in terms of both true negative rate and true positive rate while this is essentially achieved by respecting the current duration which a parking lot already sojourns in its initial state.
更多查看译文
关键词
smart cities,statistical modeling,on-street
AI 理解论文
溯源树
样例
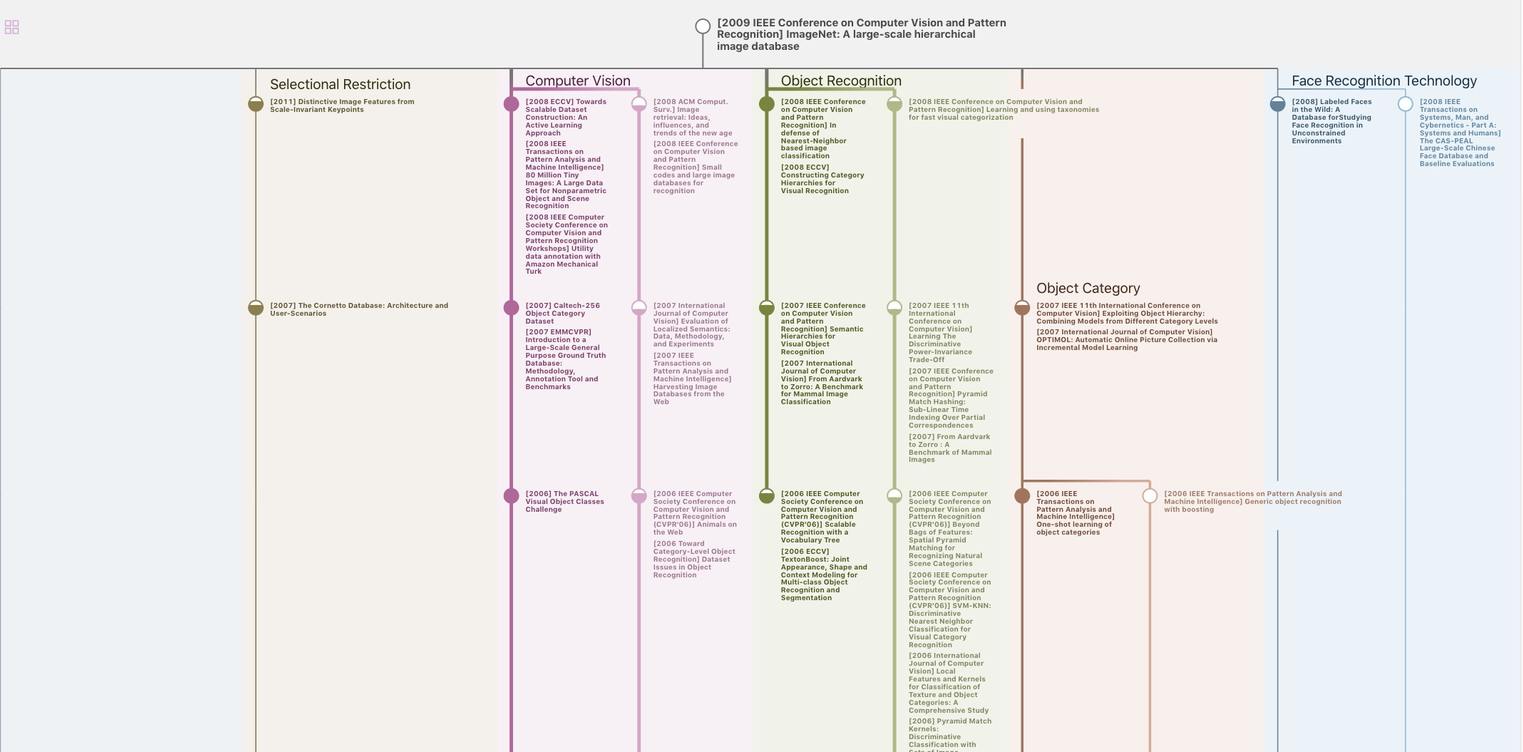
生成溯源树,研究论文发展脉络
Chat Paper
正在生成论文摘要