Predicting drag on rough surfaces by transfer learning of empirical correlations
JOURNAL OF FLUID MECHANICS(2022)
摘要
Recent developments in neural networks have shown the potential of estimating drag on irregular rough surfaces. Nevertheless, the difficulty of obtaining a large high-fidelity dataset to train neural networks is deterring their use in practical applications. In this study, we propose a transfer learning framework to model the drag on irregular rough surfaces even with a limited amount of direct numerical simulations. We show that transfer learning of empirical correlations, reported in the literature, can significantly improve the performance of neural networks for drag prediction. This is because empirical correlations include 'approximate knowledge' of the drag dependency in high-fidelity physics. The `approximate knowledge' allows neural networks to learn the surface statistics known to affect drag more efficiently. The developed framework can be applied to applications where acquiring a large dataset is difficult but empirical correlations have been reported.
更多查看译文
关键词
machine learning
AI 理解论文
溯源树
样例
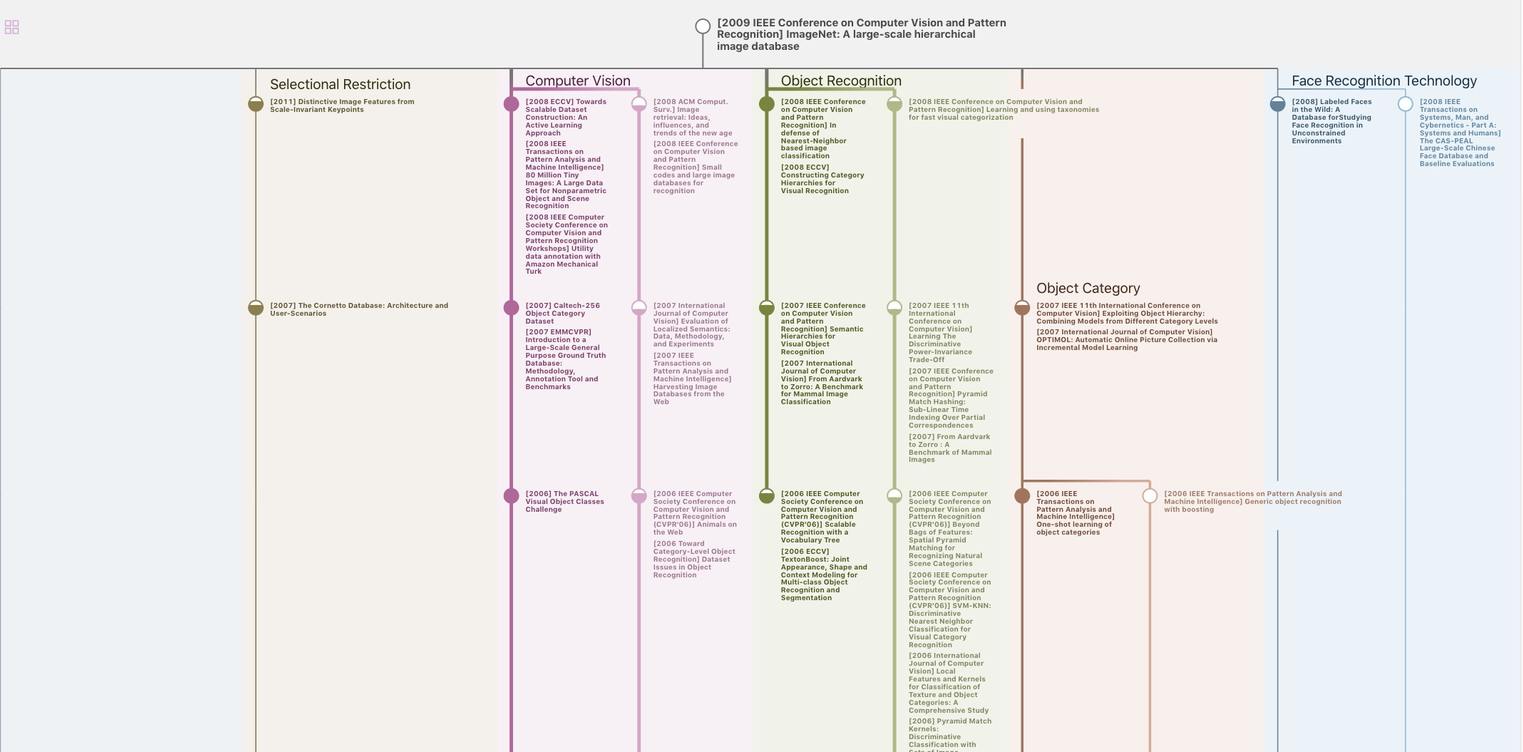
生成溯源树,研究论文发展脉络
Chat Paper
正在生成论文摘要