Nodule Slices Detection based on Weak Labels with a Novel Deep Learning Method.
ICRAI(2020)
摘要
Early detection of lung nodules is essential to the diagnosis and treatment of lung cancer. In this paper, we proposed an improved method to automatically identify the slices with lung nodules from computed tomography (CT). This deep learning-based method aimed to serve as a tool for the fast screening of lung nodules, in order to reduce CT reading time for radiologists. The proposed deep learning model combined convolutional neural networks (CNN) and variable length bidirectional Long Short-Term Memory networks (LSTM). It relied on a supervised learning approach that only required slice labels on the training dataset. The labels indicated the CT slices that contained a nodule, but not the exact location of the nodule. The proposed method was evaluated on two datasets with 5-fold cross-validation. The first dataset was collected from two 3A grade hospitals in China. It contained 1726 CT volumes (positives vs. negatives, 1:1). Each volume was labeled by at least three radiologists with more than five years of experience. The second dataset was the publicly available LIDC-IDRI database containing 888 scans, which underwent a two-phase annotation process by four experienced radiologists. For the first dataset, our method reached a high detection sensitivity of 88.2% with 0.5 false positives per CT volume. For the second dataset, we achieved a high sensitivity of 86.9% with an average of 0.8 false positives per subject. The results demonstrated that the proposed method achieved high sensitivity and specificity in identifying CT slices with lung nodules. Moreover, this study revealed that the proposed method has promising potential in reducing radiologists’ CT reading time, which only required slice labels on the training data for easy implementation.
更多查看译文
AI 理解论文
溯源树
样例
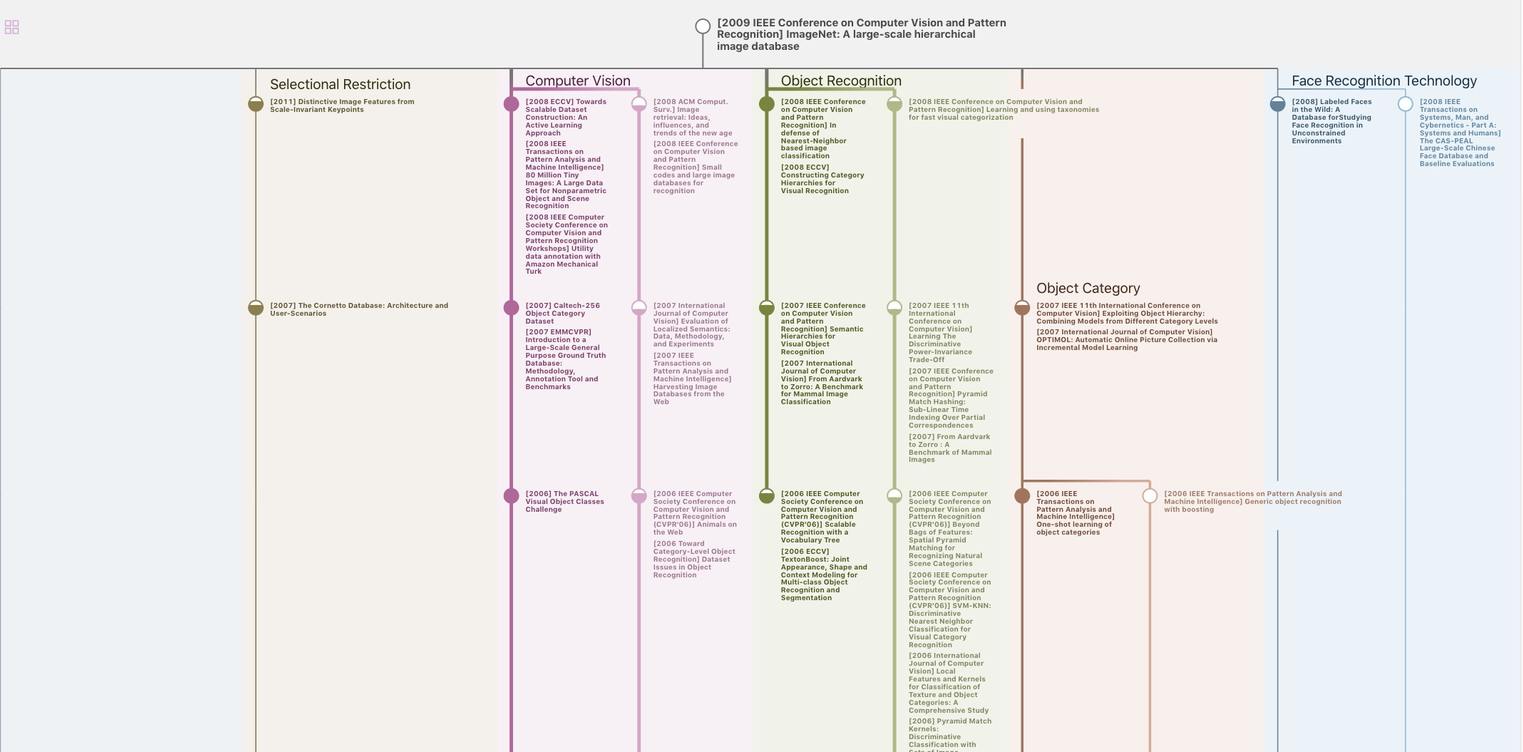
生成溯源树,研究论文发展脉络
Chat Paper
正在生成论文摘要