CAT: Cross Attention in Vision Transformer
2022 IEEE International Conference on Multimedia and Expo (ICME)(2022)
摘要
Since Transformer has found widespread use in NLP, the potential of Transformer in CV has been realized and has inspired many new approaches. However, the computation required for replacing word tokens with image patches for Transformer after the tokenization of the image is vast(e.g., ViT), which bottlenecks model training and inference. In this paper, we propose a new attention mechanism in Transformer termed Cross Attention, which alternates attention inner the image patch instead of the whole image to capture local information and apply attention between image patches which are divided from single-channel feature maps to capture global information. Both operations have less computation than standard self-attention in Transformer. Based on that, we build a hierarchical network called Cross Attention Transformer(CAT) for other vision tasks. Our model achieves 82.8% on ImageNet-1K, and improves the performance of other methods on COCO and ADE20K, illustrating that our network has the potential to serve as general backbones. The code and models are avalible at https://github.com/linhezheng19/CAT.
更多查看译文
关键词
cross attention,vision transformer,image processing
AI 理解论文
溯源树
样例
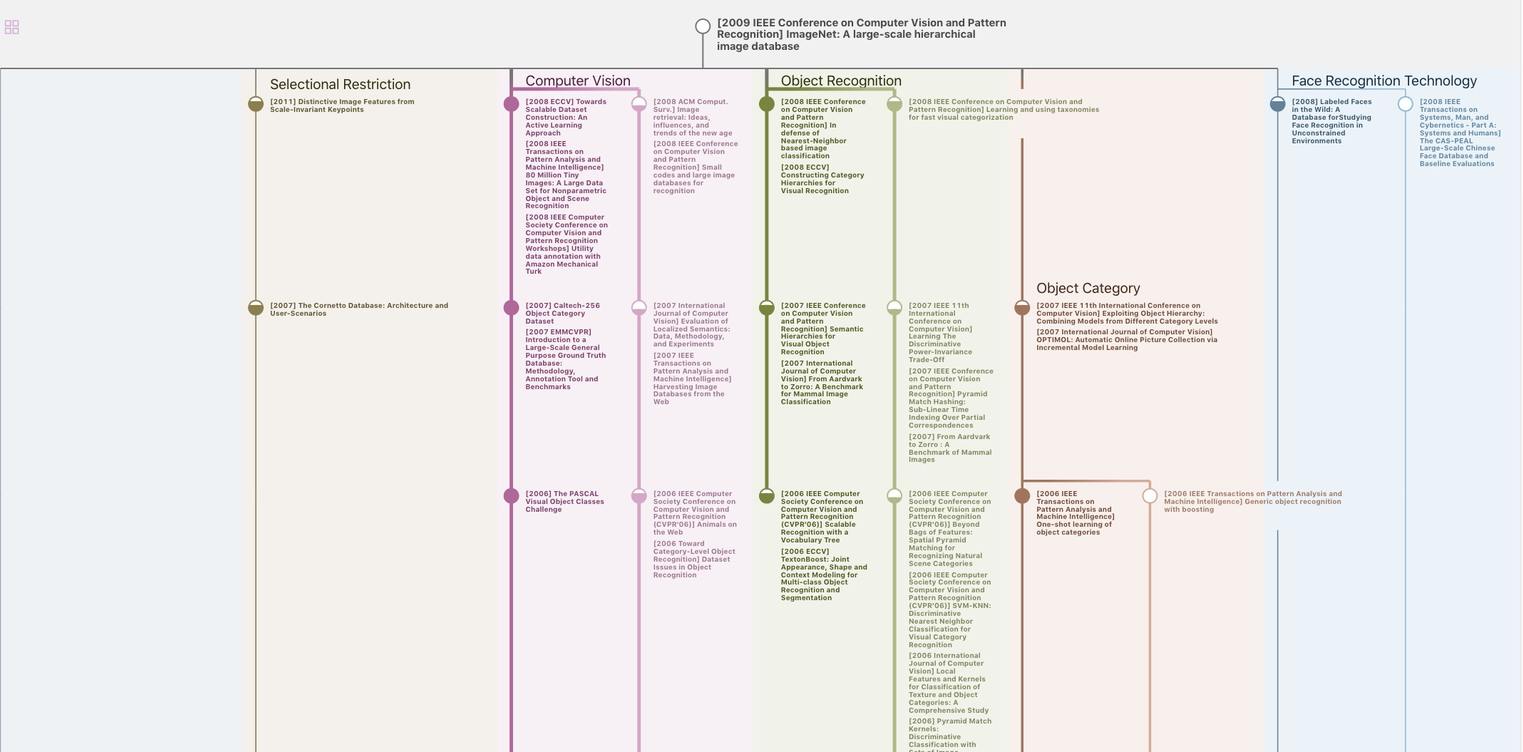
生成溯源树,研究论文发展脉络
Chat Paper
正在生成论文摘要