Fairness for Cooperative Multi-Agent Learning with Equivariant Policies
arxiv(2021)
摘要
We study fairness through the lens of cooperative multi-agent learning. Our work is motivated by empirical evidence that naive maximization of team reward yields unfair outcomes for individual team members. To address fairness in multi-agent contexts, we introduce team fairness, a group-based fairness measure for multi-agent learning. We then incorporate team fairness into policy optimization -- introducing Fairness through Equivariance (Fair-E), a novel learning strategy that achieves provably fair reward distributions. We then introduce Fairness through Equivariance Regularization (Fair-ER) as a soft-constraint version of Fair-E and show that Fair-ER reaches higher levels of utility than Fair-E and fairer outcomes than policies with no equivariance. Finally, we investigate the fairness-utility trade-off in multi-agent settings.
更多查看译文
关键词
Multiagent Systems (MAS)
AI 理解论文
溯源树
样例
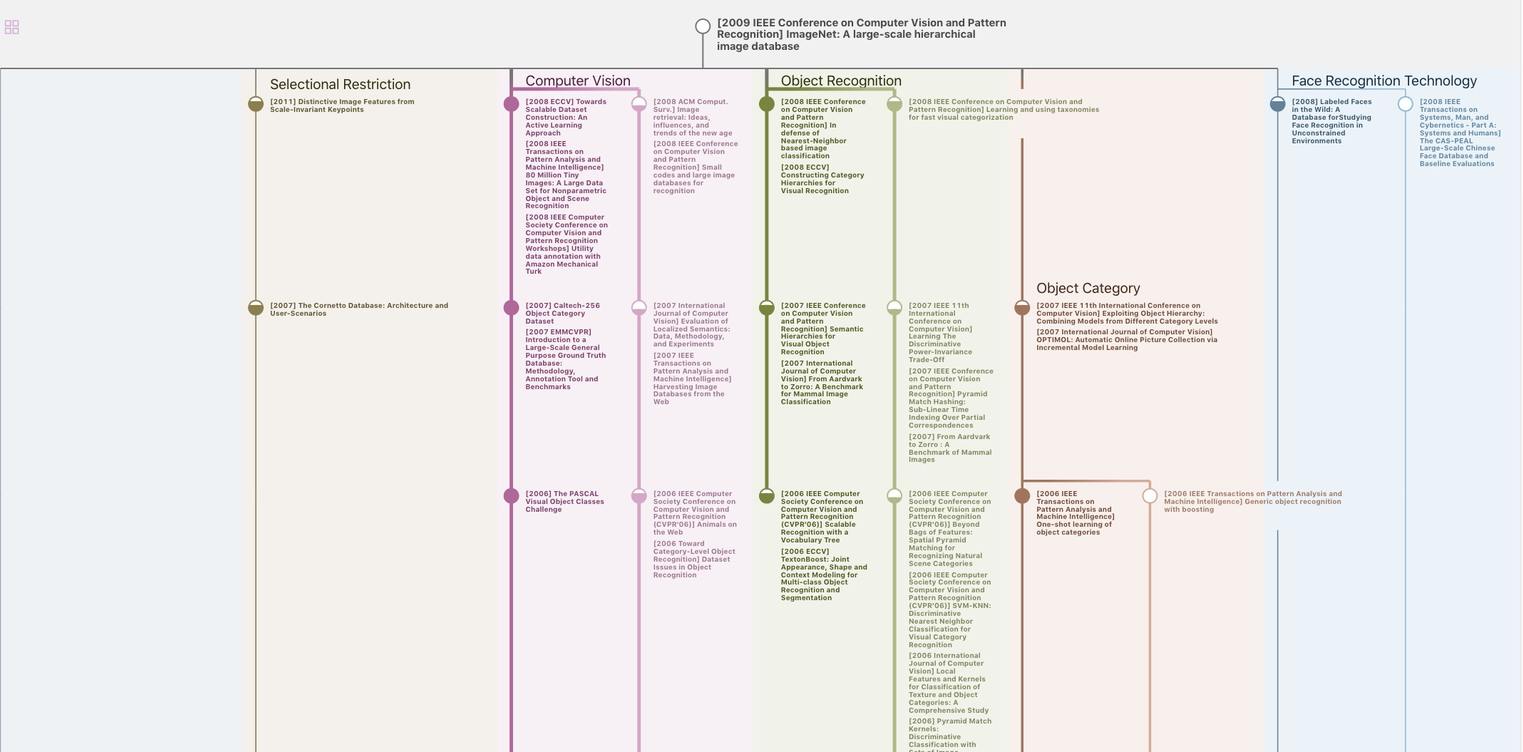
生成溯源树,研究论文发展脉络
Chat Paper
正在生成论文摘要