Cross-Domain Contrastive Learning for Unsupervised Domain Adaptation
arXiv (Cornell University)(2023)
Abstract
Unsupervised domain adaptation (UDA) aims to transfer knowledge learned from a fully-labeled source domain to a different unlabeled target domain. Most existing UDA methods learn domain-invariant feature representations by minimizing feature distances across domains. In this work, we build upon contrastive self-supervised learning to align features so as to reduce the domain discrepancy between training and testing sets. Exploring the same set of categories shared by both domains, we introduce a simple yet effective framework CDCL, for domain alignment. In particular, given an anchor image from one domain, we minimize its distances to cross-domain samples from the same class relative to those from different categories. Since target labels are unavailable, we use a clustering-based approach with carefully initialized centers to produce pseudo labels. In addition, we demonstrate that CDCL is a general framework and can be adapted to the data-free setting, where the source data are unavailable during training, with minimal modification. We conduct experiments on two widely used domain adaptation benchmarks, i.e., Office-31 and VisDA-2017, for image classification tasks, and demonstrate that CDCL achieves state-of-the-art performance on both datasets.
MoreTranslated text
Key words
Contrastive learning,unsupervised domain adaptation,source data-free
AI Read Science
Must-Reading Tree
Example
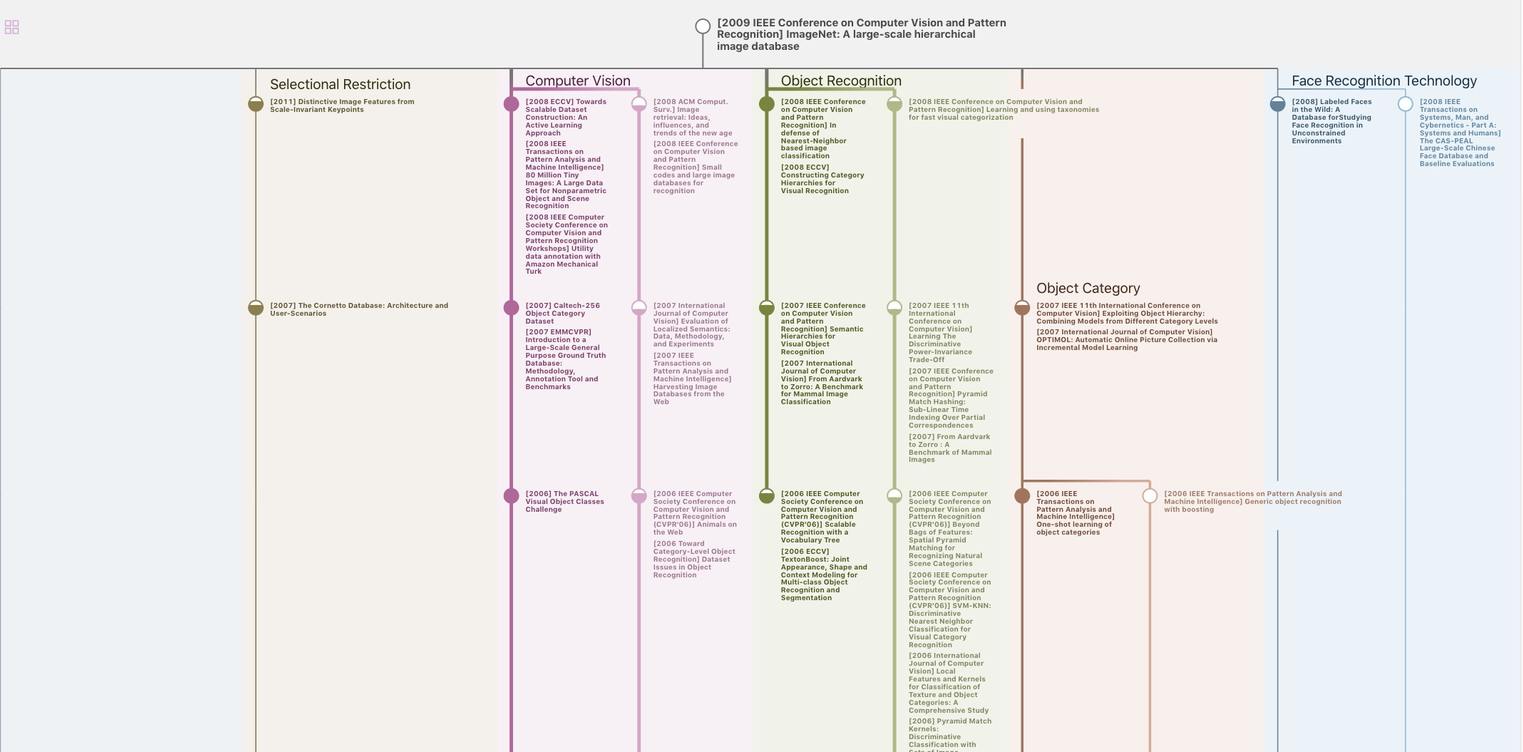
Generate MRT to find the research sequence of this paper
Chat Paper
Summary is being generated by the instructions you defined