Fine-Grained System Identification of Nonlinear Neural Circuits
Knowledge Discovery and Data Mining(2021)
摘要
ABSTRACTWe study the problem of sparse nonlinear model recovery of high dimensional compositional functions. Our study is motivated by emerging opportunities in neuroscience to recover fine-grained models of biological neural circuits using collected measurement data. Guided by available domain knowledge in neuroscience, we explore conditions under which one can recover the underlying biological circuit that generated the training data. Our results suggest insights of both theoretical and practical interests. Most notably, we find that a sign constraint on the weights is a necessary condition for system recovery, which we establish both theoretically with an identifiability guarantee and empirically on simulated biological circuits. We conclude with a case study on retinal ganglion cell circuits using data collected from mouse retina, showcasing the practical potential of this approach.
更多查看译文
关键词
nonlinear system identification, neural networks, neuroscience
AI 理解论文
溯源树
样例
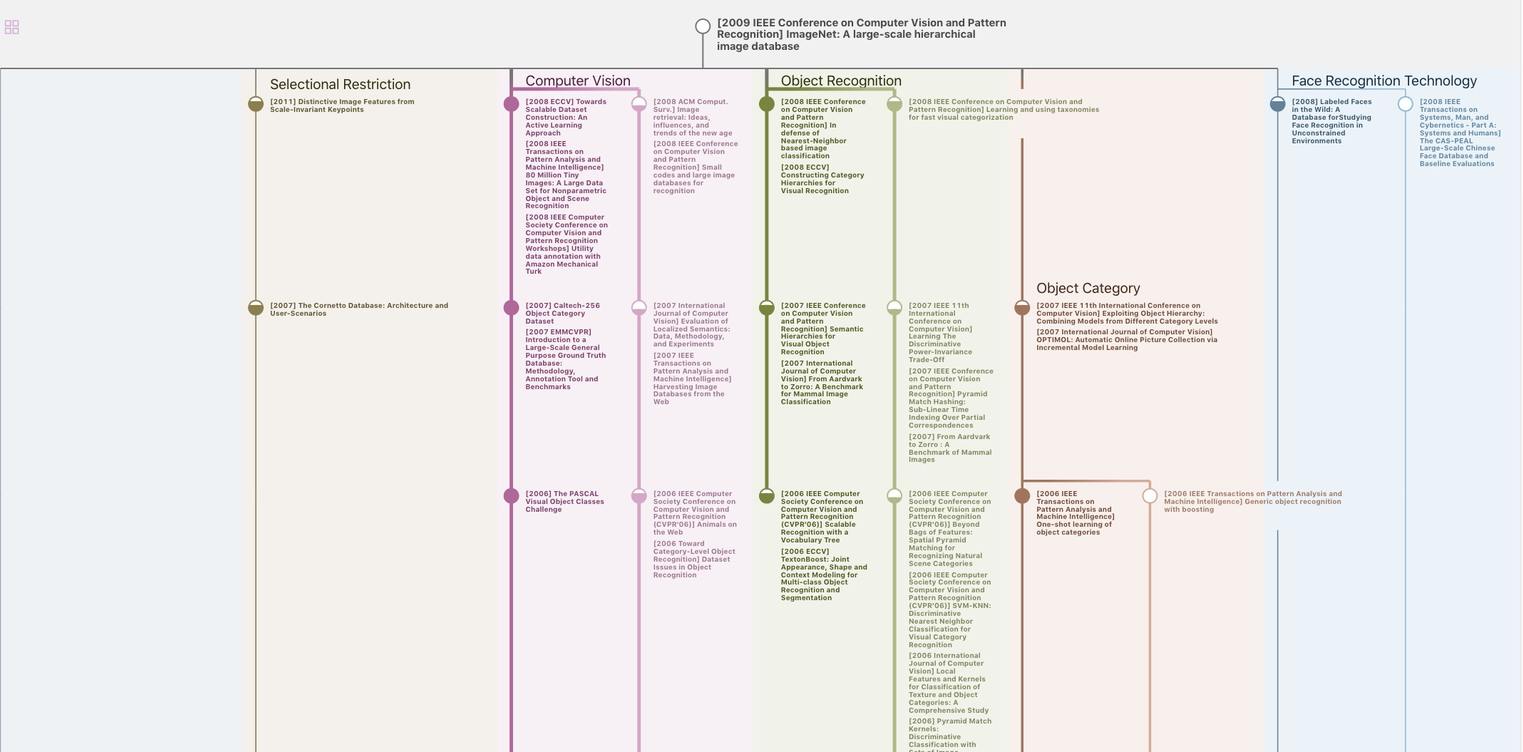
生成溯源树,研究论文发展脉络
Chat Paper
正在生成论文摘要