Adaptive Cognitive Mechanisms To Maintain Calibrated Trust And Reliance In Automation
FRONTIERS IN ROBOTICS AND AI(2021)
摘要
Trust calibration for a human-machine team is the process by which a human adjusts their expectations of the automation's reliability and trustworthiness; adaptive support for trust calibration is needed to engender appropriate reliance on automation. Herein, we leverage an instance-based learning ACT-R cognitive model of decisions to obtain and rely on an automated assistant for visual search in a UAV interface. This cognitive model matches well with the human predictive power statistics measuring reliance decisions; we obtain from the model an internal estimate of automation reliability that mirrors human subjective ratings. The model is able to predict the effect of various potential disruptions, such as environmental changes or particular classes of adversarial intrusions on human trust in automation. Finally, we consider the use of model predictions to improve automation transparency that account for human cognitive biases in order to optimize the bidirectional interaction between human and machine through supporting trust calibration. The implications of our findings for the design of reliable and trustworthy automation are discussed.
更多查看译文
关键词
cognitive architectures, ACT-R, trust in automation, automation transparency, trust calibration, human-machine teaming
AI 理解论文
溯源树
样例
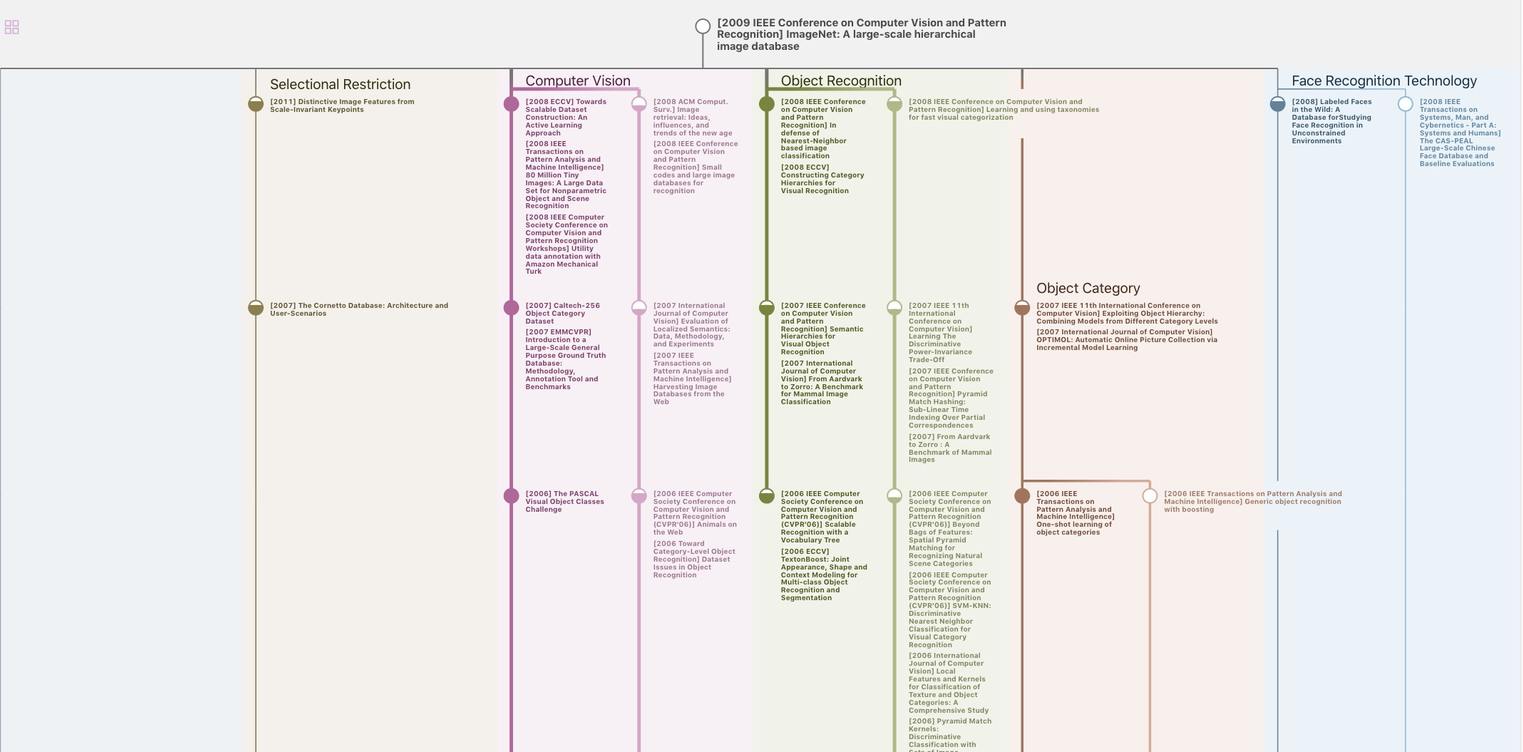
生成溯源树,研究论文发展脉络
Chat Paper
正在生成论文摘要