AMEBA: An Adaptive Approach to the Black-Box Evasion of Machine Learning Models
ASIA-CCS(2021)
摘要
ABSTRACTMachine learning models are vulnerable to evasion attacks, where the attacker starts from a correctly classified instance and perturbs it so as to induce a misclassification. In the black-box setting where the attacker only has query access to the target model, traditional attack strategies exploit a property known as transferability, i.e., the empirical observation that evasion attacks often generalize across different models. The attacker can thus rely on the following two-step attack strategy: (i) query the target model to learn how to train a surrogate model approximating it; and (ii) craft evasion attacks against the surrogate model, hoping that they "transfer" to the target model. This attack strategy is sub-optimal, because it assumes a strict separation of the two steps and under-approximates the possible actions that a real attacker might take. In this work we propose AMEBA, the first adaptive approach to the black-box evasion of machine learning models. AMEBA builds on a well-known optimization problem, known as Multi-Armed Bandit, to infer the best alternation of actions spent for surrogate model training and evasion attack crafting. We experimentally show on public datasets that AMEBA outperforms traditional two-step attack strategies.
更多查看译文
关键词
Adversarial machine learning, evasion attacks, transferability
AI 理解论文
溯源树
样例
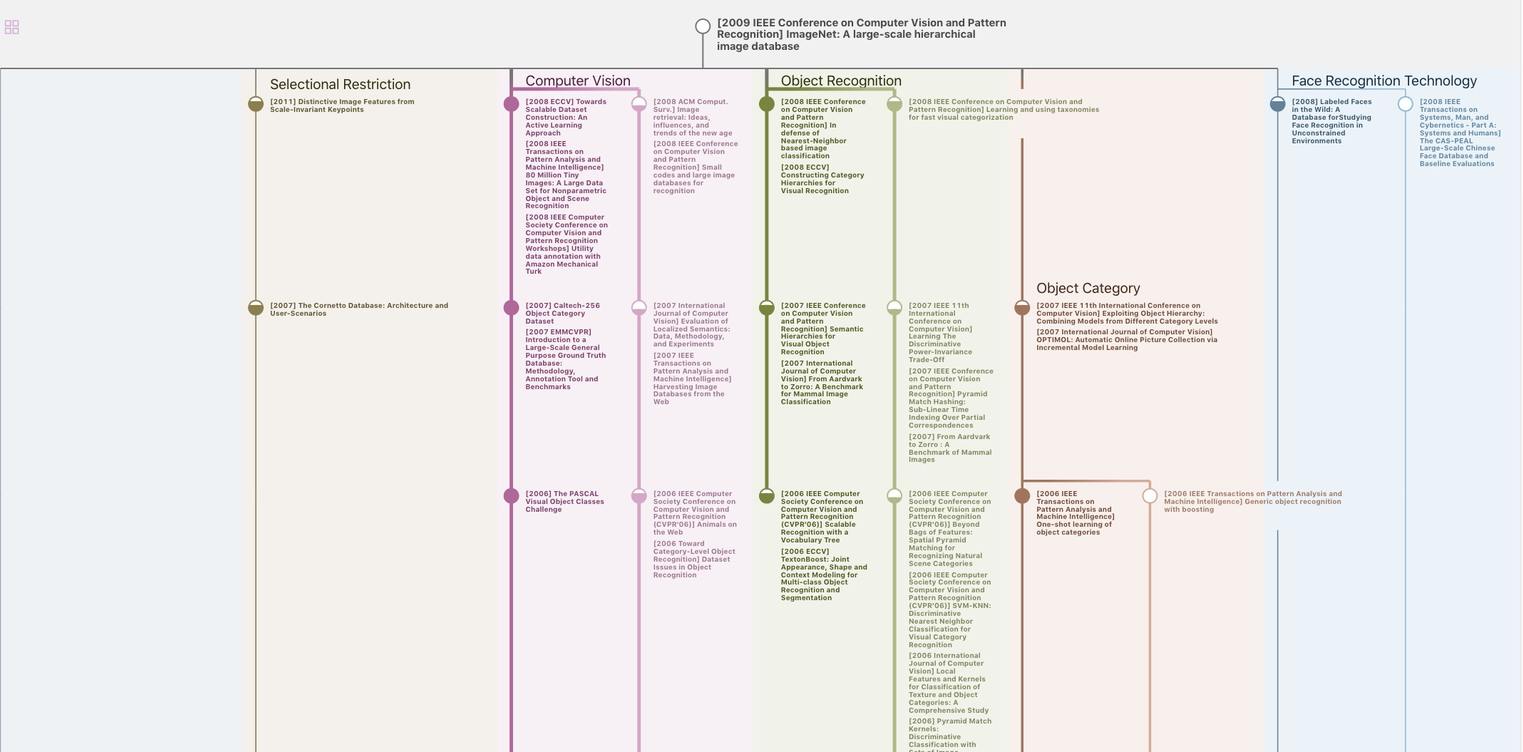
生成溯源树,研究论文发展脉络
Chat Paper
正在生成论文摘要