A Recovery Algorithm and Pooling Designs for One-Stage Noisy Group Testing Under the Probabilistic Framework.
AlCoB(2021)
摘要
Group testing saves time and resources by testing each preassigned group instead of each individual, and one-stage group testing emerged as essential for cost-effectively controlling the current COVID-19 pandemic. Yet, the practical challenge of adjusting pooling designs based on infection rate has not been systematically addressed. In particular, there are both theoretical interests and practical motivation to analyze one-stage group testing at finite, practical problem sizes, rather than asymptotic ones, under noisy, rather than perfect tests, and when the number of positives is randomly distributed, rather than fixed. Here, we study noisy group testing under the probabilistic framework by modelling the infection vector as a random vector with Bernoulli entries. Our main contributions include a practical one-stage group testing protocol guided by maximizing pool entropy and a maximum-likelihood recovery algorithm under the probabilistic framework. Our findings highlight the implications of introducing randomness to the infection vectors – we find that the combinatorial structure of the pooling designs plays a less important role than the parameters such as pool size and redundancy.
更多查看译文
关键词
Non-adaptive group testing, COVID-19, Experimental designs
AI 理解论文
溯源树
样例
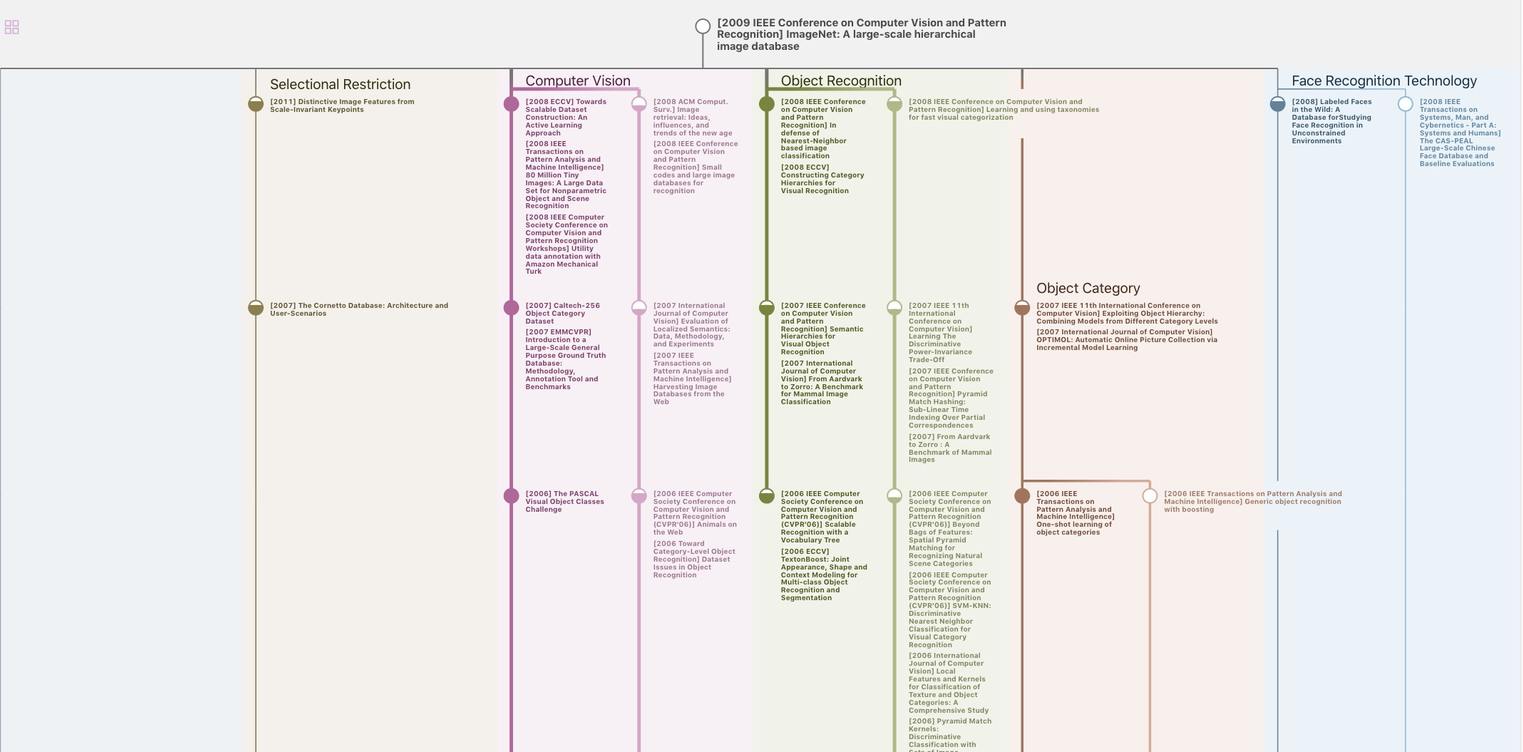
生成溯源树,研究论文发展脉络
Chat Paper
正在生成论文摘要