Joint System-Wise Optimization for Pipeline Goal-Oriented Dialog System
arxiv(2021)
摘要
Recent work (Takanobu et al., 2020) proposed the system-wise evaluation on dialog systems and found that improvement on individual components (e.g., NLU, policy) in prior work may not necessarily bring benefit to pipeline systems in system-wise evaluation. To improve the system-wise performance, in this paper, we propose new joint system-wise optimization techniques for the pipeline dialog system. First, we propose a new data augmentation approach which automates the labeling process for NLU training. Second, we propose a novel stochastic policy parameterization with Poisson distribution that enables better exploration and offers a principled way to compute policy gradient. Third, we propose a reward bonus to help policy explore successful dialogs. Our approaches outperform the competitive pipeline systems from Takanobu et al. (2020) by big margins of 12% success rate in automatic system-wise evaluation and of 16% success rate in human evaluation on the standard multi-domain benchmark dataset MultiWOZ 2.1, and also outperform the recent state-of-the-art end-to-end trained model from DSTC9.
更多查看译文
关键词
dialog,system-wise,goal-oriented
AI 理解论文
溯源树
样例
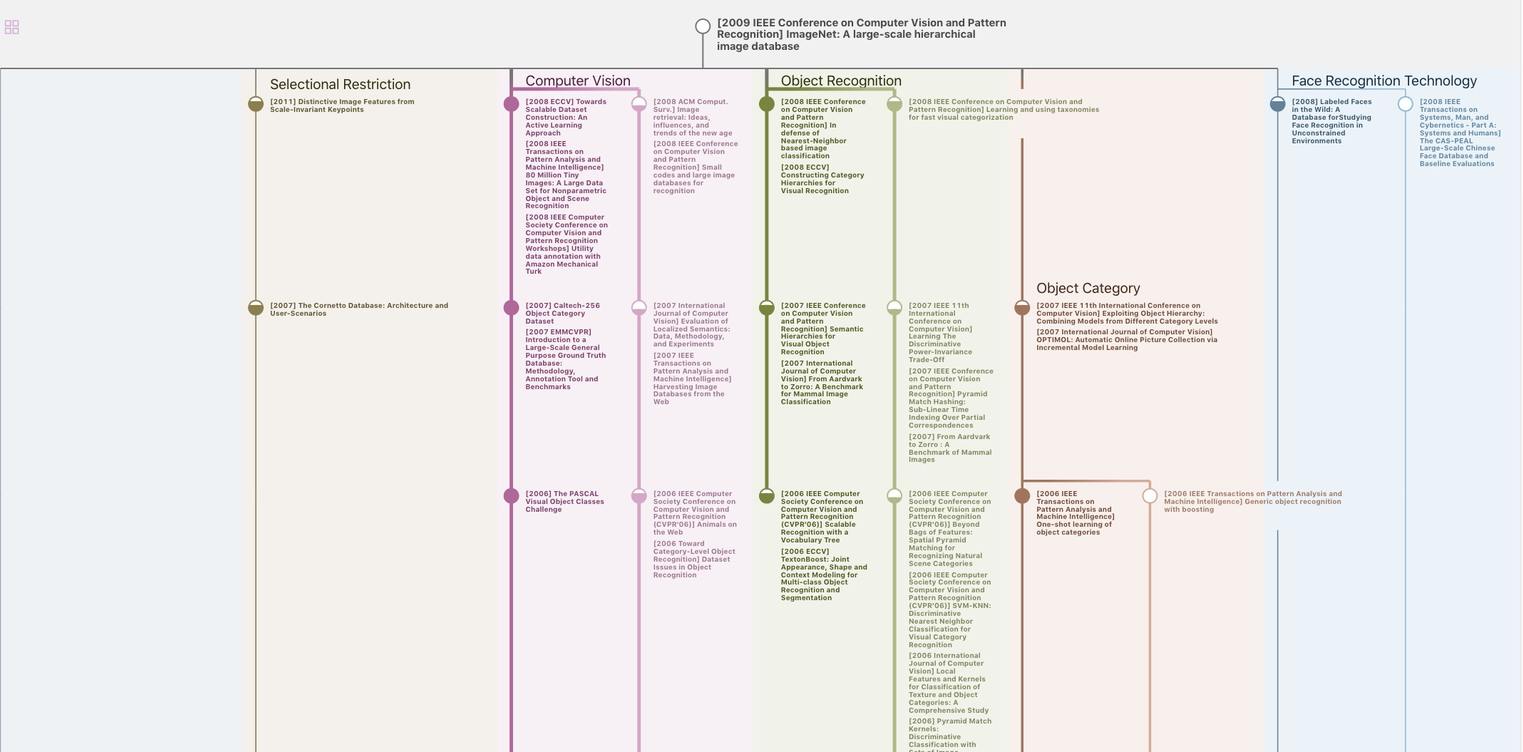
生成溯源树,研究论文发展脉络
Chat Paper
正在生成论文摘要